A Subset Of A Population Is Called A
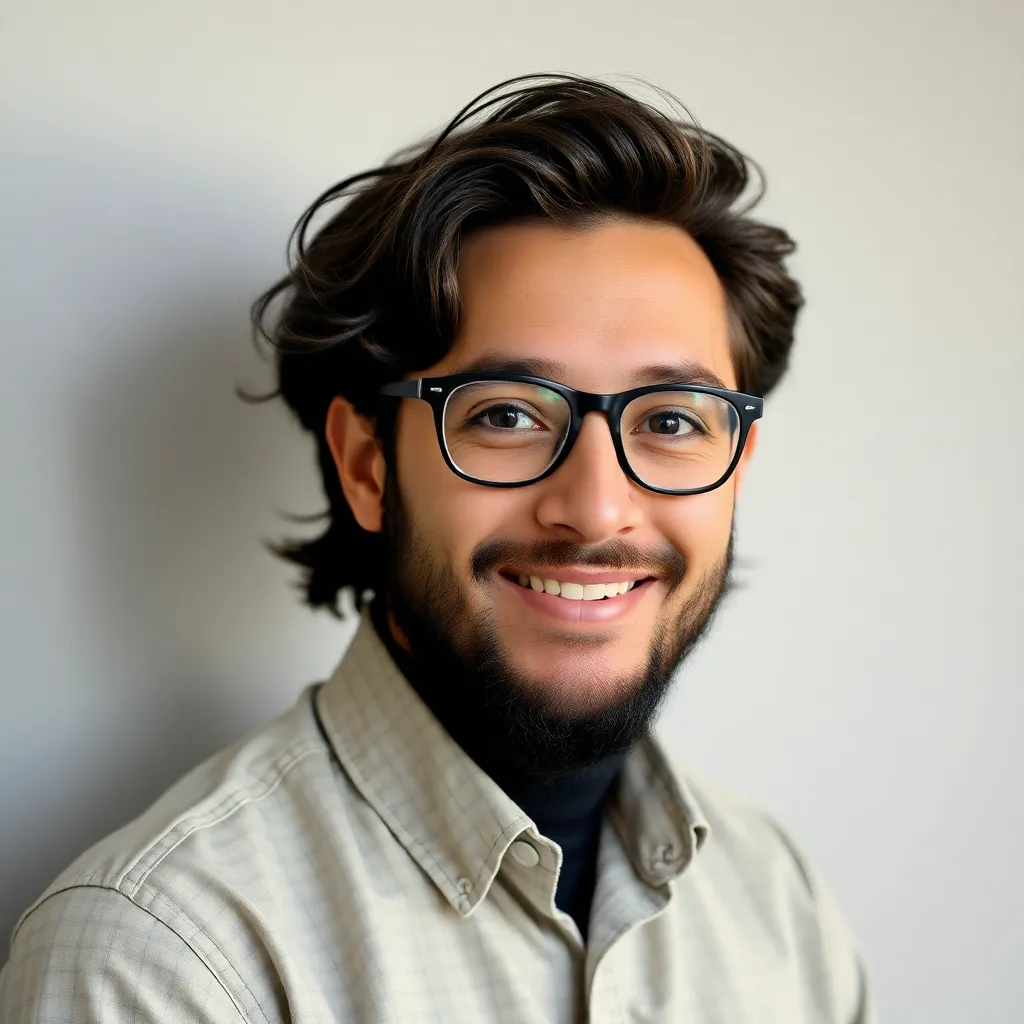
Juapaving
Mar 29, 2025 · 7 min read
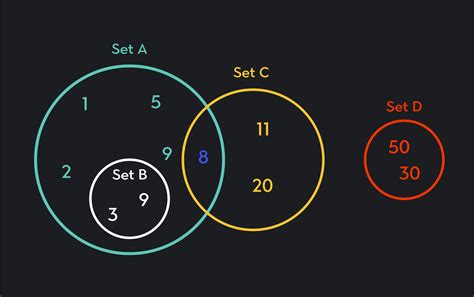
Table of Contents
A Subset of a Population is Called a: Exploring Sample Types and Statistical Inference
Understanding how we select and analyze subsets of a population is fundamental to statistics and research. A subset of a population is called a sample. This seemingly simple definition underpins a vast field of study, encompassing everything from designing effective surveys to interpreting complex clinical trials. This article will delve deep into the various types of samples, the methods used to obtain them, and the implications for making inferences about the larger population.
Defining Populations and Samples
Before we dive into the intricacies of sample types, let's clarify the core concepts:
-
Population: This refers to the entire group of individuals, objects, or events that are of interest in a study. It's the complete set from which we want to draw conclusions. For example, the population could be all registered voters in a country, all oak trees in a specific forest, or all patients diagnosed with a particular disease.
-
Sample: A sample is a smaller, representative subset of the population. It's selected to make inferences about the characteristics of the population as a whole. The sample size (the number of individuals in the sample) is crucial; a larger, well-chosen sample generally leads to more accurate and reliable inferences.
The act of selecting a sample is called sampling. The goal of good sampling is to obtain a sample that accurately reflects the characteristics of the population. If the sample is not representative, the inferences drawn from it may be biased and unreliable.
Types of Sampling Methods
There are numerous ways to select a sample from a population. These methods can be broadly classified into two main categories: probability sampling and non-probability sampling.
Probability Sampling: Every Member Has a Chance
In probability sampling, every member of the population has a known, non-zero probability of being selected for the sample. This ensures that the sample is more likely to be representative of the population and reduces the risk of bias. Several key probability sampling methods exist:
-
Simple Random Sampling: This is the most basic form of probability sampling. Every member of the population has an equal chance of being selected. Imagine drawing names from a hat—each name has the same probability of being chosen. This method is straightforward but can be impractical for large populations.
-
Stratified Random Sampling: This method involves dividing the population into distinct subgroups, or strata, based on relevant characteristics (e.g., age, gender, income). A random sample is then drawn from each stratum, ensuring representation from all subgroups. This approach is particularly useful when there are significant differences between subgroups and you want to ensure each group is adequately represented in your sample.
-
Cluster Sampling: This technique is employed when the population is naturally divided into clusters (e.g., schools, cities, hospitals). A random sample of clusters is selected, and then all members within the selected clusters are included in the sample. This is efficient for large, geographically dispersed populations, but may introduce some clustering bias if the clusters are not homogeneous.
-
Systematic Sampling: This involves selecting every kth member of the population after a random starting point. For example, selecting every 10th person from a list. This method is simple and easy to implement but can be problematic if there's a pattern in the population that aligns with the sampling interval.
Non-Probability Sampling: Not Every Member Has a Chance
In non-probability sampling, not every member of the population has a known chance of being selected. This introduces the risk of bias, but it can be more practical and cost-effective in certain situations. Some common non-probability sampling methods include:
-
Convenience Sampling: This involves selecting participants who are readily available and accessible. For example, surveying students in a university cafeteria. This method is easy but highly susceptible to bias because the sample may not be representative of the larger population.
-
Quota Sampling: This is similar to stratified sampling, but the selection within each stratum is not random. Researchers select participants to meet pre-defined quotas based on characteristics such as age, gender, or race. This ensures representation of subgroups but lacks the randomness of probability sampling.
-
Purposive Sampling (Judgmental Sampling): This involves selecting participants based on the researcher's judgment and knowledge of the population. This method is useful when specific expertise or characteristics are needed, but it's highly prone to subjective bias.
-
Snowball Sampling: This is a technique used when it's difficult to identify members of the population. Initial participants are recruited, and they then refer other participants who fit the study criteria. This method is useful for studying hidden or hard-to-reach populations, but it can lead to bias because the sample is influenced by the social networks of initial participants.
Sample Size and Statistical Power
The size of the sample is a crucial factor in determining the reliability and validity of the results. A larger sample generally provides a more accurate representation of the population and increases the statistical power of the study. Statistical power refers to the probability of finding a statistically significant result if a real effect exists. A larger sample size increases the likelihood of detecting a true effect.
However, simply increasing the sample size isn't always the solution. The quality of the sampling method is equally important. A large, biased sample will not provide reliable inferences about the population.
Inferential Statistics and Generalization
The primary purpose of sampling is to make inferences about the population based on the sample data. This involves using inferential statistics, a branch of statistics that uses sample data to draw conclusions about a population. Key concepts in inferential statistics include:
-
Estimation: Using sample data to estimate population parameters such as the mean, median, or proportion. These estimates are typically accompanied by a margin of error, which reflects the uncertainty associated with the estimate.
-
Hypothesis Testing: Formulating hypotheses about the population and testing them using sample data. This involves calculating p-values to determine the probability of observing the obtained results if the null hypothesis (the hypothesis being tested) were true. A low p-value (typically below 0.05) indicates that the null hypothesis is unlikely to be true, and the alternative hypothesis is supported.
-
Confidence Intervals: Providing a range of values within which the true population parameter is likely to fall with a specified level of confidence (e.g., a 95% confidence interval).
It's crucial to remember that inferences drawn from a sample are not definitive statements about the population. There will always be some degree of uncertainty due to sampling variability. The larger and more representative the sample, the smaller this uncertainty will be.
Avoiding Bias in Sampling
Bias in sampling can significantly distort the results and lead to inaccurate conclusions. It's essential to employ strategies to minimize bias:
-
Careful Sample Design: Selecting an appropriate sampling method is crucial. Probability sampling methods generally provide less biased samples than non-probability methods.
-
Randomization: Randomly selecting participants ensures that each member of the population has an equal chance of being selected, reducing the likelihood of systematic bias.
-
Stratification: Dividing the population into strata and sampling from each stratum can help to ensure representation from all subgroups and reduce bias.
-
Controlling for Confounding Variables: Identifying and controlling for potential confounding variables (variables that could influence the relationship between the variables of interest) can help to minimize bias.
-
Blinding: In some studies, blinding participants and/or researchers to the treatment group assignment can help to reduce bias related to expectations or subjective judgment.
Conclusion: The Importance of Accurate Sampling
A subset of a population is called a sample, and selecting a representative sample is crucial for conducting valid and reliable research. The choice of sampling method significantly impacts the accuracy and generalizability of the findings. While probability sampling methods generally produce less biased results, non-probability methods can be useful in certain situations. Careful consideration of sample size and the potential sources of bias is essential to ensure that the conclusions drawn from the sample data accurately reflect the characteristics of the larger population. Understanding these principles is fundamental to making sound judgments based on statistical data and drawing meaningful conclusions across various fields, from healthcare and market research to social sciences and environmental studies. Remember, a well-chosen sample is the foundation of strong and reliable research.
Latest Posts
Latest Posts
-
2 5 Of A Number Is 16 What Is The Number
Mar 31, 2025
-
Why Are Atoms Neutral Despite Having Charged Particles
Mar 31, 2025
-
Similarities Between A Square And A Rhombus
Mar 31, 2025
-
Coordination Of Balance And Body Movement Is Controlled By The
Mar 31, 2025
-
Why Are The Cells Generally Of A Small Size
Mar 31, 2025
Related Post
Thank you for visiting our website which covers about A Subset Of A Population Is Called A . We hope the information provided has been useful to you. Feel free to contact us if you have any questions or need further assistance. See you next time and don't miss to bookmark.