Which Of The Following Is Not A Measure Of Dispersion
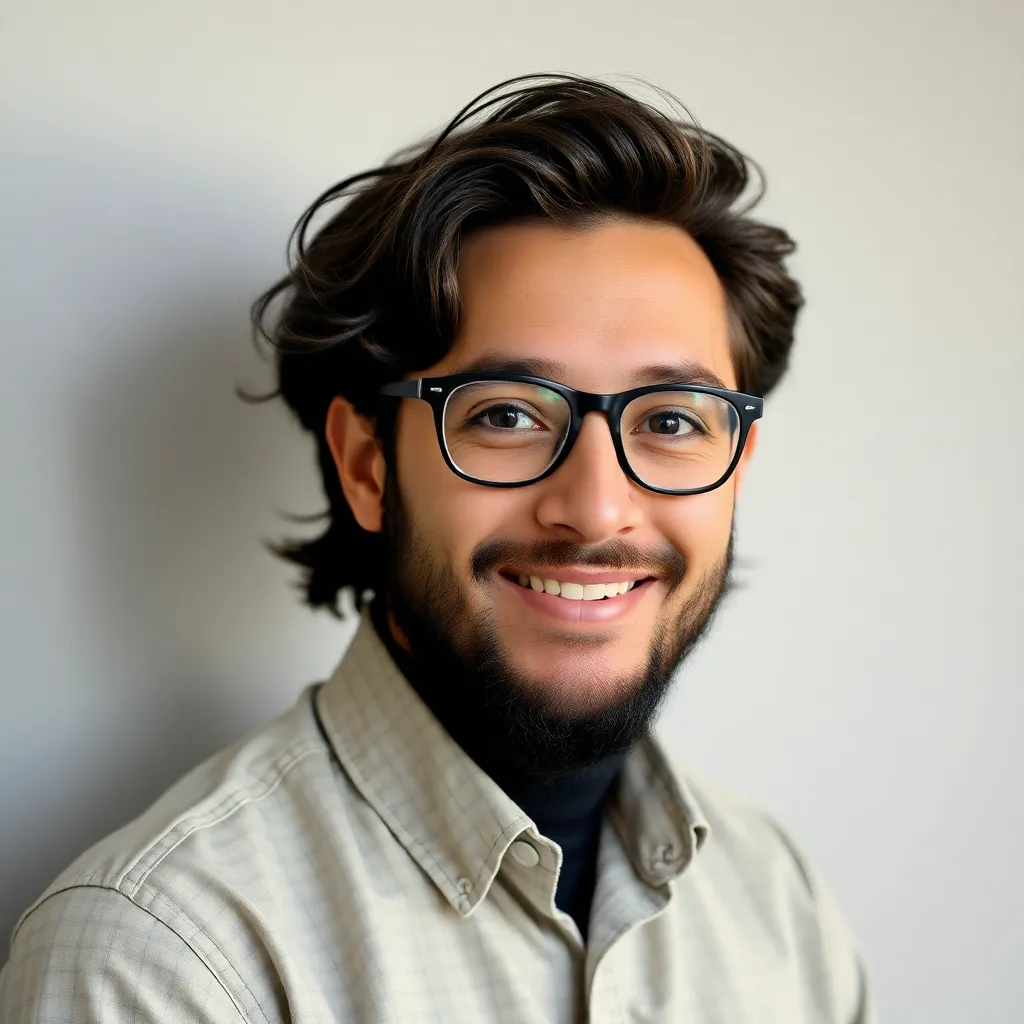
Juapaving
Apr 15, 2025 · 6 min read

Table of Contents
Which of the Following is NOT a Measure of Dispersion? Understanding Data Variability
Understanding data variability is crucial in statistics. It allows us to see how spread out our data points are, giving us a clearer picture beyond just the average. Measures of dispersion quantify this spread, revealing valuable insights into the data's characteristics. But before we delve into which measures are indicators of dispersion, let's clarify what isn't. The question, "Which of the following is NOT a measure of dispersion?" hinges on understanding the fundamental concept of dispersion itself.
What is Dispersion in Statistics?
Dispersion, also known as variability or spread, describes how spread out or scattered a dataset is. A high dispersion indicates that the data points are far apart from each other and the mean, while low dispersion suggests the data points are clustered closely around the mean. This measure is vital because it complements measures of central tendency (like mean, median, and mode) by providing a complete picture of the data distribution. Understanding both central tendency and dispersion is essential for a thorough data analysis.
Measures of Dispersion: A Comprehensive Overview
Several statistical measures quantify dispersion. Let's explore the most common ones:
1. Range: The Simplest Measure
The range is the simplest measure of dispersion. It's calculated by subtracting the smallest value from the largest value in a dataset. While easy to compute, it's highly sensitive to outliers. A single extreme value can significantly inflate the range, providing a misleading representation of the overall spread. For example, consider the dataset: {2, 4, 6, 8, 100}. The range is 98, heavily influenced by the outlier 100. Despite its limitations, the range offers a quick overview of the data's spread.
2. Interquartile Range (IQR): A More Robust Approach
The IQR addresses the range's sensitivity to outliers. It's the difference between the third quartile (Q3) and the first quartile (Q1) of a dataset. Quartiles divide the sorted data into four equal parts. The IQR represents the spread of the middle 50% of the data, making it less susceptible to extreme values. This makes it a more robust measure of dispersion than the range, especially for skewed datasets. The IQR is a key component in identifying outliers using the box plot method.
3. Variance: Measuring Average Squared Deviation
Variance measures the average squared deviation of each data point from the mean. A larger variance indicates greater dispersion. The formula for population variance (σ²) is:
σ² = Σ(xi - μ)² / N
where:
- xi represents each data point
- μ represents the population mean
- N represents the population size
For sample variance (s²), the denominator is N-1 to provide an unbiased estimate of the population variance. Variance is expressed in squared units, which can be less intuitive than other measures.
4. Standard Deviation: The Square Root of Variance
The standard deviation (σ or s) is the square root of the variance. It's expressed in the same units as the original data, making it easier to interpret. A higher standard deviation indicates greater dispersion. It's a widely used measure in many statistical analyses and is particularly valuable when dealing with normally distributed data.
5. Mean Absolute Deviation (MAD): Considering Absolute Differences
The MAD calculates the average absolute difference between each data point and the mean. It's less sensitive to outliers than the standard deviation because it uses absolute deviations instead of squared deviations. The formula for MAD is:
MAD = Σ|xi - μ| / N
where:
- xi represents each data point
- μ represents the mean
- N represents the number of data points
While less commonly used than standard deviation, MAD provides a simpler alternative for understanding the average deviation from the central tendency.
What is NOT a Measure of Dispersion?
Now, let's address the core question: which of the following is NOT a measure of dispersion? The answer depends on the options presented. However, measures of central tendency are definitively not measures of dispersion. These include:
- Mean: This represents the average value of the dataset. It indicates the central location but not the spread.
- Median: The middle value when the data is ordered. While robust to outliers, it doesn't describe the spread of data points.
- Mode: The most frequently occurring value in a dataset. It describes the most typical value but not the variability.
- Percentile: While related to quartiles (used in IQR), a single percentile value itself doesn't represent the overall dispersion. It only shows the position of a particular value within the sorted data.
Other measures, depending on the context, might not directly reflect dispersion. For example, a coefficient of variation (CV), while related to dispersion, is a relative measure comparing the standard deviation to the mean. It's useful for comparing the dispersion of datasets with different scales, but it's not a direct measure of absolute dispersion like standard deviation or range. Similarly, skewness and kurtosis, while descriptive of data distribution shape, do not directly measure dispersion. Skewness measures asymmetry, and kurtosis measures the "tailedness" of the distribution.
Identifying Non-Dispersion Measures in Multiple Choice Questions
In multiple choice questions, carefully examine the options provided. Look for terms associated with central tendency (mean, median, mode) or data shape (skewness, kurtosis). These are typically not direct measures of dispersion. Understanding the underlying concept and formulas for common dispersion measures will help you confidently select the correct answer. Remember, a measure of dispersion should directly quantify the spread or variability of the data.
Practical Applications of Measures of Dispersion
Understanding and applying measures of dispersion is crucial across diverse fields:
- Finance: Analyzing the risk associated with investments. Higher standard deviation in stock returns implies higher risk.
- Quality Control: Monitoring the consistency of a manufacturing process. Lower standard deviation signifies less variation in product quality.
- Healthcare: Assessing the variability in patient outcomes or disease prevalence.
- Sports Analytics: Evaluating the consistency of an athlete's performance.
- Environmental Science: Studying the variability of environmental parameters like temperature or rainfall.
The choice of appropriate dispersion measure depends on the specific context and characteristics of the data. Outliers significantly impact the range, making the IQR a more robust alternative. Variance and standard deviation are widely applicable and provide comprehensive measures of spread. MAD offers a simpler and more robust alternative to standard deviation in the presence of outliers. Choosing the right measure will provide a more accurate and informative interpretation of data variability.
Conclusion: Mastering Dispersion for Data Analysis
Understanding measures of dispersion is paramount for comprehensive data analysis. Being able to distinguish between measures of dispersion and other descriptive statistics, particularly measures of central tendency, is crucial for correctly interpreting data and drawing meaningful conclusions. By understanding the strengths and weaknesses of each dispersion measure, one can select the most appropriate tool for a given analysis. This ability to effectively interpret data variability translates to improved decision-making across diverse fields, highlighting the importance of mastering this fundamental statistical concept. Remember to consider the context, data distribution, and presence of outliers when choosing a measure of dispersion to ensure you're getting the most accurate picture of your data's variability.
Latest Posts
Latest Posts
-
How Do You Estimate A Quotient
May 09, 2025
-
How Many Meters In 1 5 Kilometers
May 09, 2025
-
The Vertebral Column Extends From The Skull To The
May 09, 2025
-
The Physical Expression Of An Organisms Genes
May 09, 2025
-
Which Of The Following Is Derived Unit
May 09, 2025
Related Post
Thank you for visiting our website which covers about Which Of The Following Is Not A Measure Of Dispersion . We hope the information provided has been useful to you. Feel free to contact us if you have any questions or need further assistance. See you next time and don't miss to bookmark.