The Coefficient Of Determination Is Equal To
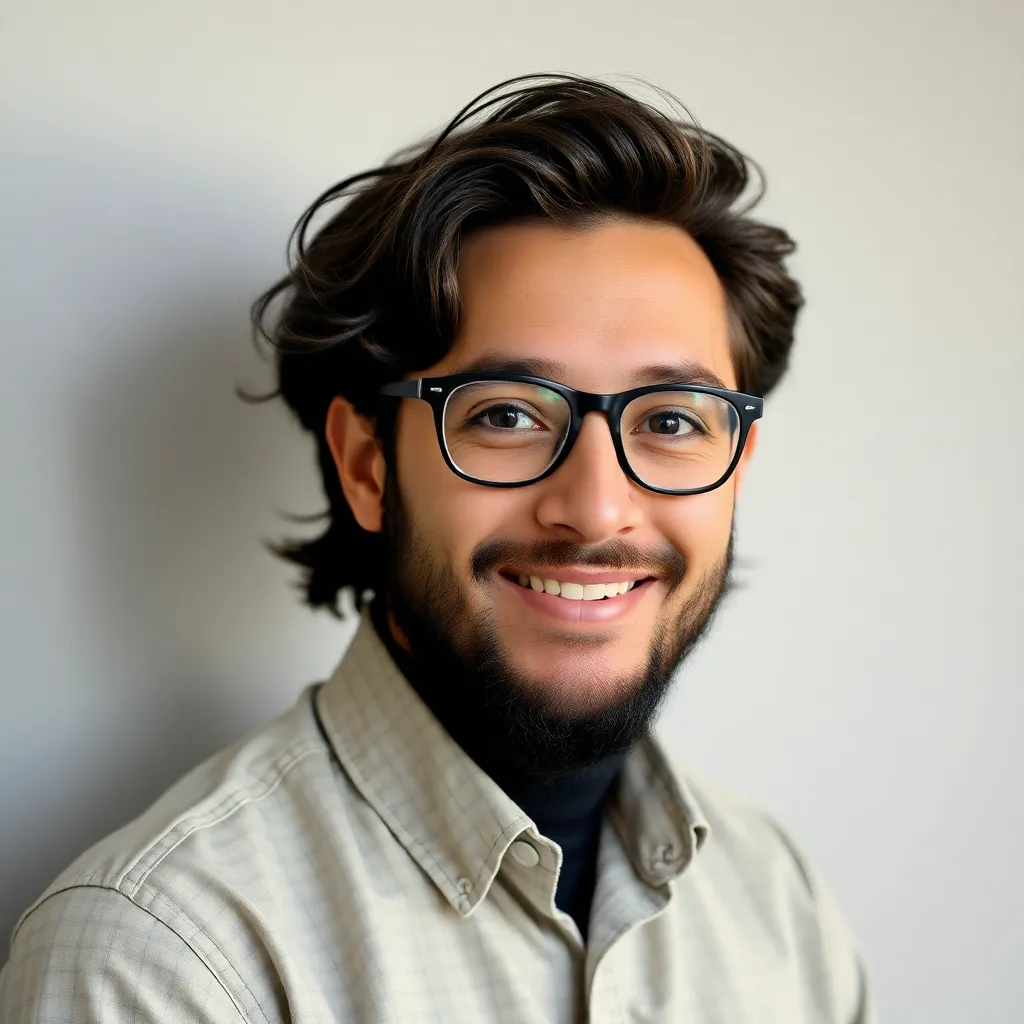
Juapaving
Mar 28, 2025 · 6 min read
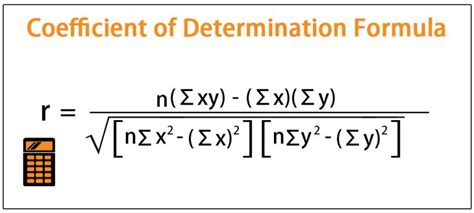
Table of Contents
The Coefficient of Determination: What it Is and What it Equals
The coefficient of determination, denoted as R², is a crucial statistical measure used in regression analysis. It quantifies the proportion of the variance in a dependent variable that's predictable from the independent variable(s). In simpler terms, it tells us how well a regression model fits the observed data. Understanding what R² equals and its implications is vital for interpreting regression results accurately. This comprehensive guide will delve deep into the meaning of R², exploring its calculation, interpretation, and limitations.
What Does R² Actually Represent?
At its core, R² represents the goodness of fit of a regression model. A higher R² value indicates a better fit, meaning the model explains a larger portion of the variability in the dependent variable. Conversely, a lower R² suggests a poorer fit, with the model explaining only a small fraction of the variation.
Imagine this: You're trying to predict house prices (dependent variable) based on their size (independent variable). A high R² would suggest that house size is a strong predictor of price, with the model accurately capturing a significant portion of the price variations. A low R², however, would imply that house size alone isn't a sufficient predictor, and other factors significantly influence the price.
R² is always expressed as a value between 0 and 1, or equivalently, as a percentage between 0% and 100%.
- R² = 0: The model explains none of the variability in the dependent variable. The independent variable(s) offer no predictive power.
- R² = 1: The model explains all the variability in the dependent variable. The independent variable(s) perfectly predict the dependent variable.
- 0 < R² < 1: The model explains some, but not all, of the variability in the dependent variable. The value of R² indicates the strength of the relationship.
Calculating R²: The Mathematical Underpinnings
R² is mathematically defined as the ratio of the explained variance to the total variance. Let's break down the calculation:
1. Total Sum of Squares (SST): This represents the total variability in the dependent variable. It measures the sum of the squared differences between each observed value and the mean of the dependent variable.
Formula: SST = Σ(yᵢ - ȳ)² where:
- yᵢ represents the individual observed values of the dependent variable.
- ȳ represents the mean of the dependent variable.
2. Regression Sum of Squares (SSR): This represents the variability in the dependent variable explained by the regression model. It's the sum of the squared differences between the predicted values (from the regression model) and the mean of the dependent variable.
Formula: SSR = Σ(ŷᵢ - ȳ)² where:
- ŷᵢ represents the predicted values of the dependent variable from the regression model.
3. Residual Sum of Squares (SSE): This represents the unexplained variability in the dependent variable – the variability that the model fails to capture. It's the sum of the squared differences between the observed values and the predicted values.
Formula: SSE = Σ(yᵢ - ŷᵢ)²
The Relationship: These three sums of squares are related by the following equation:
SST = SSR + SSE
Finally, the coefficient of determination is calculated as:
R² = SSR / SST = 1 - (SSE / SST)
This equation shows that R² can be calculated either by dividing the explained variance (SSR) by the total variance (SST) or by subtracting the ratio of unexplained variance (SSE) to total variance (SST) from 1.
Interpreting R²: Practical Considerations
While a high R² generally indicates a good fit, its interpretation needs careful consideration. Several factors influence its value and can lead to misinterpretations.
1. The Number of Independent Variables:
Adding more independent variables to a regression model always increases R², even if those variables are irrelevant. This is because the model has more parameters to fit the data, thus potentially reducing the SSE. This phenomenon highlights the importance of considering the adjusted R².
2. Adjusted R²: Penalizing for Complexity
The adjusted R² (R²adj) addresses the issue of adding irrelevant variables by penalizing the model's complexity. It adjusts the R² value based on the number of independent variables and the sample size. A higher adjusted R² indicates a better fit, even when considering the number of predictors.
Formula: R²adj = 1 - [(1 - R²) * (n - 1) / (n - k - 1)] where:
- n is the sample size.
- k is the number of independent variables.
3. The Context of the Data:
The interpretation of R² should always be within the context of the specific application and data. A high R² might be expected in some fields (e.g., physics), while a lower R² might be acceptable in others (e.g., social sciences). The practical significance of the explained variance is crucial.
4. Causation vs. Correlation:
It's critical to remember that a high R² doesn't imply causation. While a high R² suggests a strong relationship between the independent and dependent variables, it doesn't prove that changes in the independent variable cause changes in the dependent variable. Other factors could be influencing the relationship.
Limitations of R²
Despite its usefulness, R² has certain limitations:
- Doesn't assess model adequacy: A high R² doesn't guarantee that the model is a good representation of the underlying data-generating process. Other diagnostic tests are needed to assess assumptions like linearity, homoscedasticity, and normality of residuals.
- Sensitive to outliers: Outliers can significantly inflate the R² value, giving a misleading impression of the model's goodness of fit.
- Not suitable for all models: R² is primarily used for linear regression models. Its interpretation can be more complex for non-linear models.
- Can be misleading with small sample sizes: In small samples, R² can be artificially inflated, leading to overestimation of the model's predictive ability.
R² in Different Regression Models
While the basic concept of R² remains consistent, its calculation and interpretation might vary slightly across different regression models.
1. Linear Regression:
In simple and multiple linear regression, R² directly measures the proportion of variance explained by the linear relationship between the independent and dependent variables.
2. Logistic Regression:
In logistic regression (used for binary outcome variables), a direct equivalent of R² doesn't exist. Pseudo-R² measures, such as McFadden's R² or Cox and Snell's R², are often used to assess the model's goodness of fit, but they lack the straightforward interpretation of R² in linear regression.
Conclusion: R² as a Powerful, Yet Limited, Tool
The coefficient of determination, R², is a cornerstone of regression analysis. It offers a concise summary of the model's ability to explain the variance in the dependent variable. However, its interpretation requires careful consideration of the context, adjusted R², and potential limitations. A high R² is desirable, but it shouldn't be the sole criterion for model selection. Researchers must consider other diagnostic tests and the practical significance of the results before drawing conclusions. Remember that correlation doesn't equal causation, and a good model goes beyond simply explaining a high percentage of variance. It also needs to be accurate, reliable, and interpretable within the given context. By understanding both the strengths and limitations of R², researchers can utilize it effectively as a valuable tool in their statistical analyses.
Latest Posts
Latest Posts
-
What Is The Least Common Denominator Of 8 And 12
Mar 31, 2025
-
5 Letter Words With Er In The End
Mar 31, 2025
-
What Are The Common Factors Of 28 And 36
Mar 31, 2025
-
What Is 6 10 As A Percentage
Mar 31, 2025
-
Cual Es La Diferencia Entre Sent Y Send
Mar 31, 2025
Related Post
Thank you for visiting our website which covers about The Coefficient Of Determination Is Equal To . We hope the information provided has been useful to you. Feel free to contact us if you have any questions or need further assistance. See you next time and don't miss to bookmark.