The Assembly And Analysis Of Discharged Patient Records Is Called
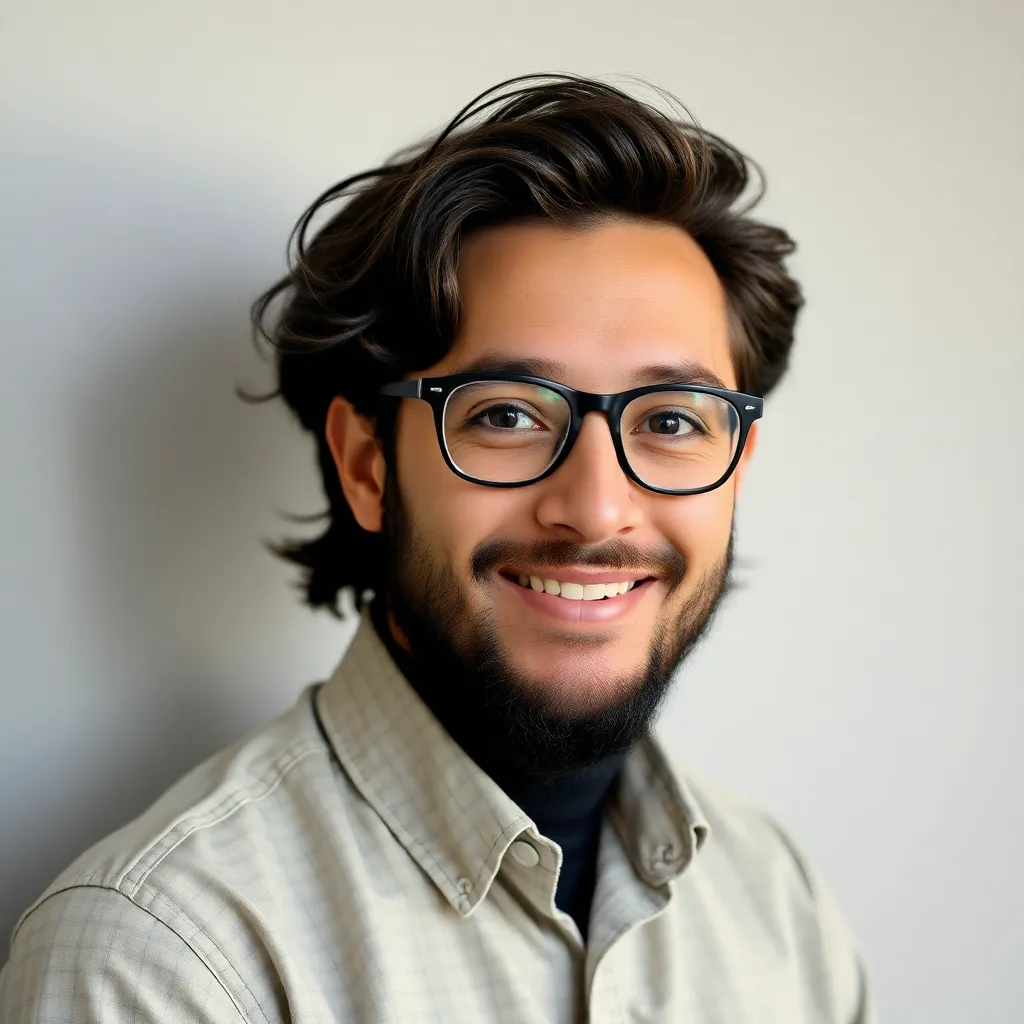
Juapaving
May 25, 2025 · 6 min read
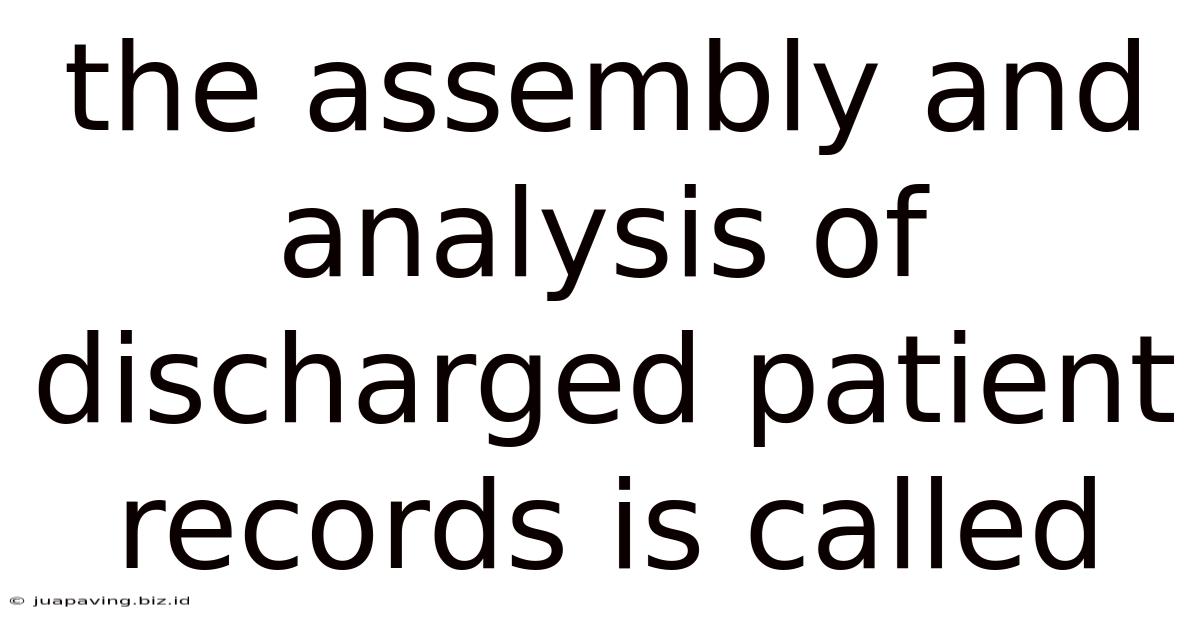
Table of Contents
The Assembly and Analysis of Discharged Patient Records: A Comprehensive Guide to Data Mining in Healthcare
The assembly and analysis of discharged patient records, more formally known as discharge summary analysis, is a crucial process in healthcare. It's not simply about compiling data; it's about extracting valuable insights that can improve patient care, enhance operational efficiency, and drive research advancements. This in-depth guide explores the multifaceted aspects of this process, encompassing its methodology, applications, challenges, and the ethical considerations involved.
Understanding the Scope of Discharged Patient Records
Discharged patient records represent a wealth of information concerning a patient's journey through a healthcare facility. They contain a comprehensive summary of the patient's:
- Demographics: Age, gender, address, contact information.
- Medical History: Past illnesses, surgeries, allergies, family history.
- Reason for Admission: The primary diagnosis and any secondary diagnoses.
- Treatment Received: Medications, therapies, procedures performed.
- Test Results: Laboratory results, imaging studies, etc.
- Progress Notes: Daily documentation of the patient's condition and response to treatment.
- Discharge Summary: A concise overview of the patient's hospitalization, including the final diagnosis, treatment plan, and follow-up instructions.
- Discharge Medications: A detailed list of prescribed medications upon discharge.
The assembly of these records requires a meticulous approach, ensuring data accuracy and completeness. Any inconsistencies or missing information can significantly impact the reliability of subsequent analysis.
The Process of Assembling Discharged Patient Records
The assembly of discharged patient records typically involves several key steps:
1. Data Identification and Retrieval:
This initial stage focuses on identifying and locating the relevant patient records. This might involve searching electronic health record (EHR) systems, retrieving paper-based records, or accessing data from multiple sources. The process must adhere to strict data privacy and security protocols, complying with regulations like HIPAA in the US or GDPR in Europe.
2. Data Cleaning and Standardization:
Once the records are assembled, the next crucial step is data cleaning. This involves correcting errors, handling missing values, and standardizing data formats. Inconsistencies in data entry across different healthcare providers can lead to significant challenges, highlighting the need for standardized terminologies and coding systems such as SNOMED CT and ICD codes.
3. Data Integration:
In many cases, patient data resides across multiple systems and formats. Data integration aims to consolidate this information into a unified and accessible database. This may require employing specialized data integration tools and techniques.
4. Data Validation:
Before any analysis can be undertaken, it's critical to validate the assembled data. This involves verifying the accuracy, completeness, and consistency of the information. Data validation techniques often include cross-checking data against multiple sources and employing data quality checks.
Analyzing Discharged Patient Records: Unlocking Valuable Insights
The analysis of discharged patient records offers a multitude of opportunities for improving healthcare outcomes. The methods employed depend on the specific research question or objective. Some common analytical techniques include:
1. Descriptive Statistics:
This fundamental approach involves summarizing the characteristics of the dataset using measures like mean, median, mode, standard deviation, and frequency distributions. For instance, one might calculate the average length of stay for patients with a particular diagnosis or the prevalence of specific complications.
2. Regression Analysis:
Regression analysis explores the relationships between different variables in the dataset. For example, it might be used to identify risk factors associated with readmissions or to predict the likelihood of developing certain complications based on patient characteristics and treatment received.
3. Cohort Studies:
This longitudinal approach involves tracking a group of patients over time to observe outcomes and identify patterns. By analyzing discharged patient records, researchers can assess the long-term effects of different treatments or interventions.
4. Case-Control Studies:
In these studies, researchers compare a group of patients with a specific condition (cases) to a group of patients without the condition (controls) to identify factors associated with the condition. Discharged patient records can provide the necessary data for this type of analysis.
5. Machine Learning:
Advanced machine learning techniques are increasingly being applied to discharged patient records to identify patterns, predict outcomes, and personalize treatment plans. Algorithms like predictive modeling can help anticipate readmissions, identify patients at high risk of complications, and optimize resource allocation.
6. Natural Language Processing (NLP):
NLP techniques are crucial for extracting meaningful information from unstructured data like clinical notes and physician reports. NLP algorithms can automate the extraction of key medical concepts, facilitating more efficient data analysis.
Applications of Discharged Patient Record Analysis
The insights derived from discharged patient record analysis have far-reaching implications across various aspects of healthcare:
1. Improving Patient Care:
Analysis can reveal patterns and trends in patient outcomes, enabling healthcare providers to refine treatment protocols, identify areas for improvement, and ultimately enhance the quality of care delivered.
2. Enhancing Operational Efficiency:
By identifying bottlenecks and inefficiencies in the healthcare workflow, analysis can inform strategic decisions regarding resource allocation, staffing, and process optimization. For instance, analyzing average length of stay can highlight areas for streamlining care.
3. Reducing Healthcare Costs:
Identifying factors associated with readmissions or complications can lead to preventative strategies that reduce healthcare costs. Targeting high-risk patients with proactive interventions can save resources and improve outcomes.
4. Supporting Clinical Research:
Discharged patient records provide a valuable resource for conducting clinical research, allowing researchers to investigate the effectiveness of different treatments, identify risk factors for various conditions, and advance medical knowledge.
5. Public Health Surveillance:
Analyzing aggregated data from discharged patient records can contribute to public health surveillance by identifying outbreaks of infectious diseases, tracking the prevalence of chronic conditions, and informing public health interventions.
Challenges in the Analysis of Discharged Patient Records
Despite its immense potential, analyzing discharged patient records presents several significant challenges:
1. Data Quality Issues:
Inconsistent data entry, missing values, and errors in documentation can compromise the accuracy and reliability of the analysis. Robust data cleaning and validation procedures are crucial to mitigate these issues.
2. Data Security and Privacy:
Protecting patient confidentiality is paramount. Strict adherence to data privacy regulations and the implementation of strong security measures are essential. Anonymization and de-identification techniques are often used to safeguard patient information.
3. Data Heterogeneity:
The diversity of data formats and terminologies used across different healthcare providers poses a significant challenge. Standardized coding systems and data integration techniques are crucial for overcoming this hurdle.
4. Data Volume and Complexity:
The sheer volume and complexity of healthcare data can make analysis challenging. Sophisticated analytical tools and techniques are necessary to manage and analyze this vast amount of information effectively.
5. Ethical Considerations:
Ethical considerations surrounding data use and interpretation are crucial. Transparency, informed consent, and responsible data management are vital to maintain ethical standards.
Conclusion: The Future of Discharged Patient Record Analysis
The assembly and analysis of discharged patient records is an increasingly important aspect of healthcare. As technology advances and data analytical techniques become more sophisticated, the potential benefits of this process will only grow. By addressing the challenges related to data quality, security, and ethics, healthcare providers and researchers can unlock the immense potential of discharged patient records to improve patient care, enhance operational efficiency, and drive advancements in medical research and public health. The future of this field lies in integrating advanced analytics, AI, and machine learning to unlock even more comprehensive and actionable insights, paving the way for a more personalized and effective healthcare system.
Latest Posts
Latest Posts
-
Deconstruct The Given Diels Alder Adduct
May 25, 2025
-
A Decomposition Reaction Can Be Symbolized By
May 25, 2025
-
What Are The Key Criticisms Of The Interagency Process
May 25, 2025
-
In The Left Footer Section Insert The Sheet Name Field
May 25, 2025
-
Unit 5 Progress Check Frq Ap Biology
May 25, 2025
Related Post
Thank you for visiting our website which covers about The Assembly And Analysis Of Discharged Patient Records Is Called . We hope the information provided has been useful to you. Feel free to contact us if you have any questions or need further assistance. See you next time and don't miss to bookmark.