2 Variances F Hypothesis Test Calculator
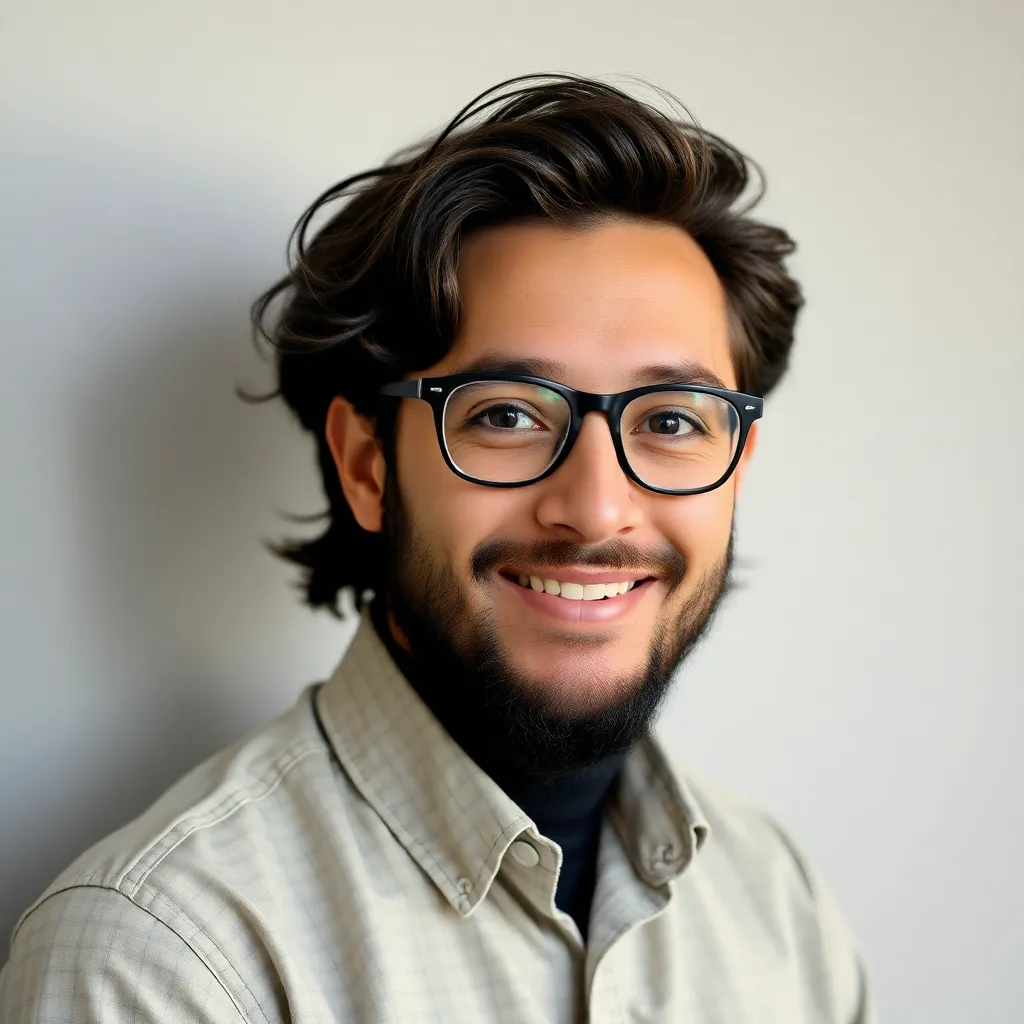
Juapaving
Apr 02, 2025 · 6 min read
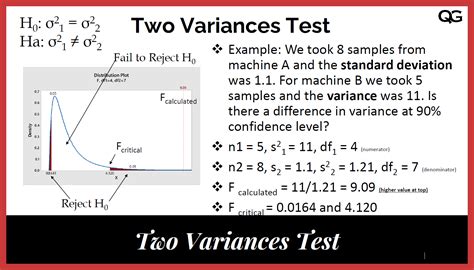
Table of Contents
2 Variances F-Test Calculator: A Comprehensive Guide
Understanding statistical significance is crucial for drawing reliable conclusions from data. When comparing the variances of two populations, the F-test provides a powerful tool. This comprehensive guide explores the two-variances F-test, its applications, assumptions, interpretations, and how to use an F-test calculator effectively.
What is the Two-Variances F-Test?
The two-variances F-test, also known as the F-test for equality of variances, is a statistical test used to determine if two population variances are significantly different. It compares the ratio of the variances of two independent samples, utilizing the F-statistic. This test is particularly useful in various fields, including:
- Quality Control: Assessing the consistency of manufacturing processes.
- Experimental Design: Comparing the variability of treatment groups.
- Finance: Analyzing the volatility of different investment portfolios.
- Healthcare: Evaluating the variability of patient outcomes across different treatments.
Understanding the F-Statistic
The F-statistic is calculated as the ratio of the larger sample variance to the smaller sample variance. This ratio follows an F-distribution, which is defined by two degrees of freedom:
- Degrees of freedom for the numerator (df1): This is calculated as n1 - 1, where n1 is the sample size of the group with the larger variance.
- Degrees of freedom for the denominator (df2): This is calculated as n2 - 1, where n2 is the sample size of the group with the smaller variance.
A higher F-statistic suggests a greater difference in variances, potentially indicating that the population variances are not equal. The critical value from the F-distribution is used to determine if this difference is statistically significant.
Assumptions of the Two-Variances F-Test
The accuracy and reliability of the F-test hinge on certain assumptions about the data:
- Independence: The two samples must be independent of each other. Observations in one sample should not influence the observations in the other.
- Normality: Both populations from which the samples are drawn should be approximately normally distributed. While the F-test is somewhat robust to deviations from normality, especially with larger sample sizes, significant departures can affect the accuracy of the results. Consider using alternative non-parametric tests if normality assumptions are severely violated.
- Random Sampling: Samples should be randomly selected from their respective populations to ensure they are representative.
Steps to Perform a Two-Variances F-Test using a Calculator
While statistical software packages offer robust F-test functionalities, dedicated online calculators simplify the process. A typical two-variances F-test calculator requires the following inputs:
- Sample Variance 1 (s1²): The variance of the first sample.
- Sample Variance 2 (s2²): The variance of the second sample.
- Sample Size 1 (n1): The number of observations in the first sample.
- Sample Size 2 (n2): The number of observations in the second sample.
- Significance Level (α): The probability of rejecting the null hypothesis when it is actually true (typically 0.05 or 5%). This is also known as the Type I error rate.
The calculator then performs the following computations:
- Calculates the F-statistic: This is computed as the ratio of the larger sample variance to the smaller sample variance.
- Determines the critical F-value: The calculator uses the degrees of freedom (df1 and df2) and the significance level (α) to find the critical F-value from the F-distribution table.
- Compares the calculated F-statistic to the critical F-value:
- If the calculated F-statistic is greater than the critical F-value: The null hypothesis (that the variances are equal) is rejected. This suggests a statistically significant difference between the two population variances.
- If the calculated F-statistic is less than or equal to the critical F-value: The null hypothesis is not rejected. There is insufficient evidence to conclude a significant difference between the variances.
- Provides the p-value: The p-value represents the probability of observing the obtained results (or more extreme results) if the null hypothesis is true. A p-value less than the significance level (α) leads to the rejection of the null hypothesis.
Interpreting the Results
The output of the F-test calculator usually includes the calculated F-statistic, the p-value, and the critical F-value. The interpretation depends on comparing the calculated F-statistic to the critical F-value or by evaluating the p-value:
-
P-value Approach: If the p-value is less than the significance level (e.g., 0.05), the null hypothesis is rejected, indicating a statistically significant difference between the two variances. Otherwise, the null hypothesis is not rejected.
-
Critical Value Approach: If the calculated F-statistic exceeds the critical F-value, the null hypothesis is rejected. This signifies a statistically significant difference in variances.
It's crucial to remember that statistical significance does not automatically imply practical significance. A statistically significant difference might be small and inconsequential in the real-world context. Always consider the magnitude of the difference in variances alongside the statistical significance.
Choosing Between One-tailed and Two-tailed Tests
The choice between a one-tailed and a two-tailed F-test depends on the research question:
- Two-tailed test: This is used when you are interested in detecting a difference in either direction (i.e., whether the variance of one population is either greater than or less than the variance of the other population). This is the most common approach.
- One-tailed test: This is used when you have a specific directional hypothesis (e.g., you hypothesize that the variance of population 1 is greater than the variance of population 2). A one-tailed test is more powerful if the direction of the difference is known beforehand. However, it is less flexible than a two-tailed test. Most online calculators default to a two-tailed test, but some offer the option to specify a one-tailed test.
Limitations and Alternatives to the F-Test
While the F-test is a valuable tool, it has limitations:
- Sensitivity to Non-Normality: As previously mentioned, severe deviations from normality can affect the accuracy of the F-test.
- Unequal Sample Sizes: The F-test is more sensitive to deviations from normality when sample sizes are unequal.
- Outliers: Outliers can heavily influence the variance calculations, thus impacting the results of the F-test.
In cases where the assumptions of the F-test are violated or when dealing with non-normal data, consider using non-parametric alternatives such as:
- Levene's test: This test is more robust to non-normality than the F-test.
- Brown-Forsythe test: A modified version of Levene's test that is less sensitive to outliers.
Conclusion: Empowering Data Analysis with the F-Test Calculator
The two-variances F-test is a vital statistical tool for comparing population variances. Using a user-friendly F-test calculator streamlines the analysis process, allowing researchers and analysts to focus on interpreting the results and drawing meaningful conclusions. However, it is crucial to understand the underlying assumptions of the test and consider alternative methods if these assumptions are not met. Remember to always interpret the results in the context of the research question and consider both statistical and practical significance. By utilizing these tools and understanding their limitations, you can make more informed decisions based on your data. Properly understanding and employing the F-test can significantly enhance the rigor and reliability of your statistical analyses. Remember to always critically evaluate your results and consider the limitations of the test in the context of your data.
Latest Posts
Latest Posts
-
What Is The Lowest Common Multiple Of 10 And 15
Apr 03, 2025
-
Which Planets Do Not Have Moons
Apr 03, 2025
-
What Is The Perimeter Of A Parallelogram
Apr 03, 2025
-
Can A Pure Substance Be Separated By Physical Means
Apr 03, 2025
-
Does A Ribosome Have A Membrane
Apr 03, 2025
Related Post
Thank you for visiting our website which covers about 2 Variances F Hypothesis Test Calculator . We hope the information provided has been useful to you. Feel free to contact us if you have any questions or need further assistance. See you next time and don't miss to bookmark.