Z Table For Two Tailed Test
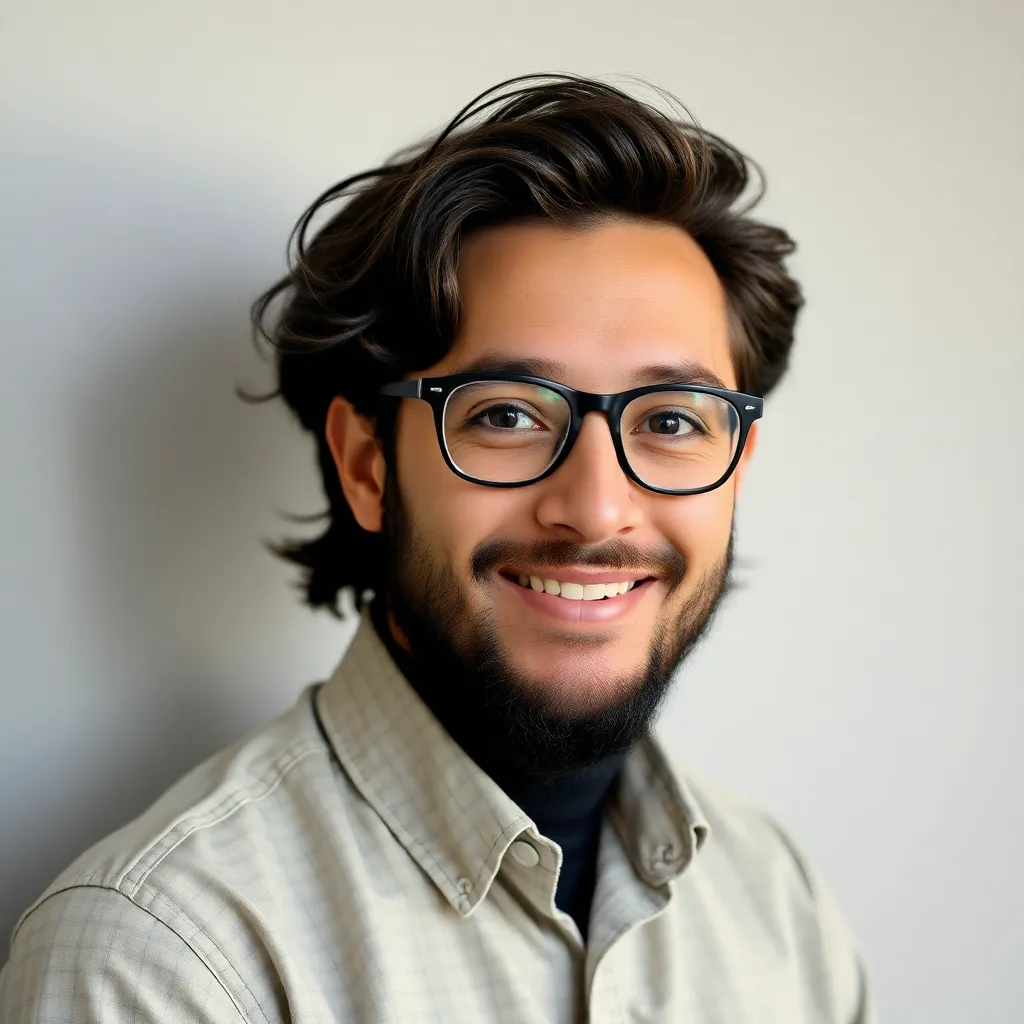
Juapaving
May 11, 2025 · 6 min read

Table of Contents
Demystifying the Z-Table for Two-Tailed Hypothesis Tests
The Z-table, also known as the standard normal distribution table, is a crucial tool in statistical hypothesis testing. It allows us to determine the probability associated with a given Z-score, which is essential for making inferences about a population based on sample data. While many understand its use in one-tailed tests, the application of the Z-table for two-tailed tests often presents a greater challenge. This comprehensive guide will thoroughly explain the Z-table and its application to two-tailed hypothesis testing, demystifying the process step-by-step.
Understanding the Z-Table and Z-Scores
Before diving into two-tailed tests, let's review the fundamentals. The Z-table displays the cumulative probability of a standard normal distribution. A standard normal distribution is a bell-shaped curve with a mean of 0 and a standard deviation of 1. A Z-score represents the number of standard deviations a particular data point is from the mean. A positive Z-score indicates the data point is above the mean, while a negative Z-score indicates it's below the mean.
Interpreting the Z-Table
The Z-table is organized with Z-scores listed in rows (representing the whole number and tenths place) and columns (representing the hundredths place). The cell values represent the cumulative probability from negative infinity up to the Z-score. For example, if you look up a Z-score of 1.96, you'll find a probability of approximately 0.975. This means there's a 97.5% chance that a randomly selected data point from a standard normal distribution will be less than or equal to 1.96 standard deviations from the mean.
Two-Tailed Hypothesis Tests: A Deeper Dive
A two-tailed hypothesis test examines whether a sample mean differs significantly from a population mean in either direction (greater than or less than). Unlike a one-tailed test, which focuses on a single direction, a two-tailed test considers both possibilities. This makes it more conservative but also provides a more comprehensive analysis.
Setting Up the Hypothesis
A two-tailed hypothesis test always involves a null hypothesis (H₀) and an alternative hypothesis (H₁). The null hypothesis typically states there's no significant difference between the sample mean and the population mean. The alternative hypothesis, on the other hand, states there is a significant difference. This is often represented as:
- H₀: μ = μ₀ (There is no significant difference)
- H₁: μ ≠ μ₀ (There is a significant difference)
where:
- μ represents the population mean
- μ₀ represents the hypothesized population mean
Choosing the Significance Level (α)
The significance level (α), also known as the alpha level, is the probability of rejecting the null hypothesis when it's actually true (Type I error). Commonly used significance levels are 0.05 (5%) and 0.01 (1%). This value determines the critical Z-scores that define the rejection regions.
Calculating the Test Statistic (Z-score)
To determine if there is sufficient evidence to reject the null hypothesis, we need to calculate the Z-score using the following formula:
Z = (x̄ - μ₀) / (σ / √n)
Where:
- x̄ represents the sample mean
- μ₀ represents the hypothesized population mean
- σ represents the population standard deviation
- n represents the sample size
Determining the Critical Z-scores
Because it's a two-tailed test, we need to divide the significance level (α) by 2. This gives us the area in each tail of the distribution. We then look up this value (α/2) in the Z-table to find the corresponding critical Z-score. For example, if α = 0.05, α/2 = 0.025. Looking up 0.025 in the Z-table (or its complement, 1 - 0.025 = 0.975), we find a critical Z-score of approximately ±1.96. This means the rejection regions are Z < -1.96 and Z > 1.96.
Making a Decision
Once we have the calculated Z-score and the critical Z-scores, we can make a decision:
- If the calculated Z-score falls within the rejection regions (Z < -1.96 or Z > 1.96 in our example), we reject the null hypothesis. This suggests there's sufficient evidence to conclude a significant difference exists between the sample mean and the hypothesized population mean.
- If the calculated Z-score falls outside the rejection regions (between -1.96 and 1.96 in our example), we fail to reject the null hypothesis. This suggests there's not enough evidence to conclude a significant difference.
Illustrative Example: Applying the Z-Table for a Two-Tailed Test
Let's say a researcher wants to test whether the average height of adult women in a certain city is different from the national average of 64 inches. A random sample of 100 women from the city yields a sample mean height of 65 inches, with a known population standard deviation of 2.5 inches. Let's conduct a two-tailed Z-test with a significance level of α = 0.05.
-
State the Hypotheses:
- H₀: μ = 64 inches
- H₁: μ ≠ 64 inches
-
Calculate the Z-score:
- Z = (65 - 64) / (2.5 / √100) = 4
-
Determine the Critical Z-scores:
- α/2 = 0.05 / 2 = 0.025
- The critical Z-score from the Z-table for 0.025 is approximately ±1.96
-
Make a Decision:
- Our calculated Z-score (4) falls within the rejection region (Z > 1.96).
- Therefore, we reject the null hypothesis. There is sufficient evidence to suggest that the average height of adult women in the city is significantly different from the national average.
Common Pitfalls and Considerations
While the Z-table is a powerful tool, it's essential to be aware of some common pitfalls:
-
Assumption of Normality: The Z-test assumes the underlying population is normally distributed. If this assumption is violated, the results might be unreliable. Consider using non-parametric tests if normality cannot be assumed.
-
Known Population Standard Deviation: The Z-test requires knowledge of the population standard deviation (σ). If this is unknown, the t-test should be used instead.
-
Sample Size: The accuracy of the Z-test improves with larger sample sizes. Smaller sample sizes might lead to less reliable results.
-
Interpretation: Remember that failing to reject the null hypothesis doesn't necessarily prove the null hypothesis is true. It simply means there isn't enough evidence to reject it based on the available data.
Conclusion
Mastering the use of the Z-table for two-tailed hypothesis tests is a cornerstone of statistical analysis. By carefully following the steps outlined in this guide – defining hypotheses, calculating Z-scores, determining critical values, and making informed decisions – researchers can draw meaningful conclusions from their data and contribute to a deeper understanding of the phenomena under investigation. Remember to always consider the assumptions of the test and interpret the results cautiously, taking into account the limitations and potential errors. Understanding these nuances will enable you to use the Z-table effectively and confidently in your statistical analyses.
Latest Posts
Latest Posts
-
Which Quadrilaterals Have Diagonals That Are Perpendicular
May 12, 2025
-
Molar Mass Of Iron Iii Hydroxide
May 12, 2025
-
In Dna Which Nitrogenous Base Pairs With Adenine
May 12, 2025
-
Where Are The Neutrons Located In The Atom
May 12, 2025
-
Awesome Words That Start With X
May 12, 2025
Related Post
Thank you for visiting our website which covers about Z Table For Two Tailed Test . We hope the information provided has been useful to you. Feel free to contact us if you have any questions or need further assistance. See you next time and don't miss to bookmark.