Which Correlation Is Most Likely A Causation
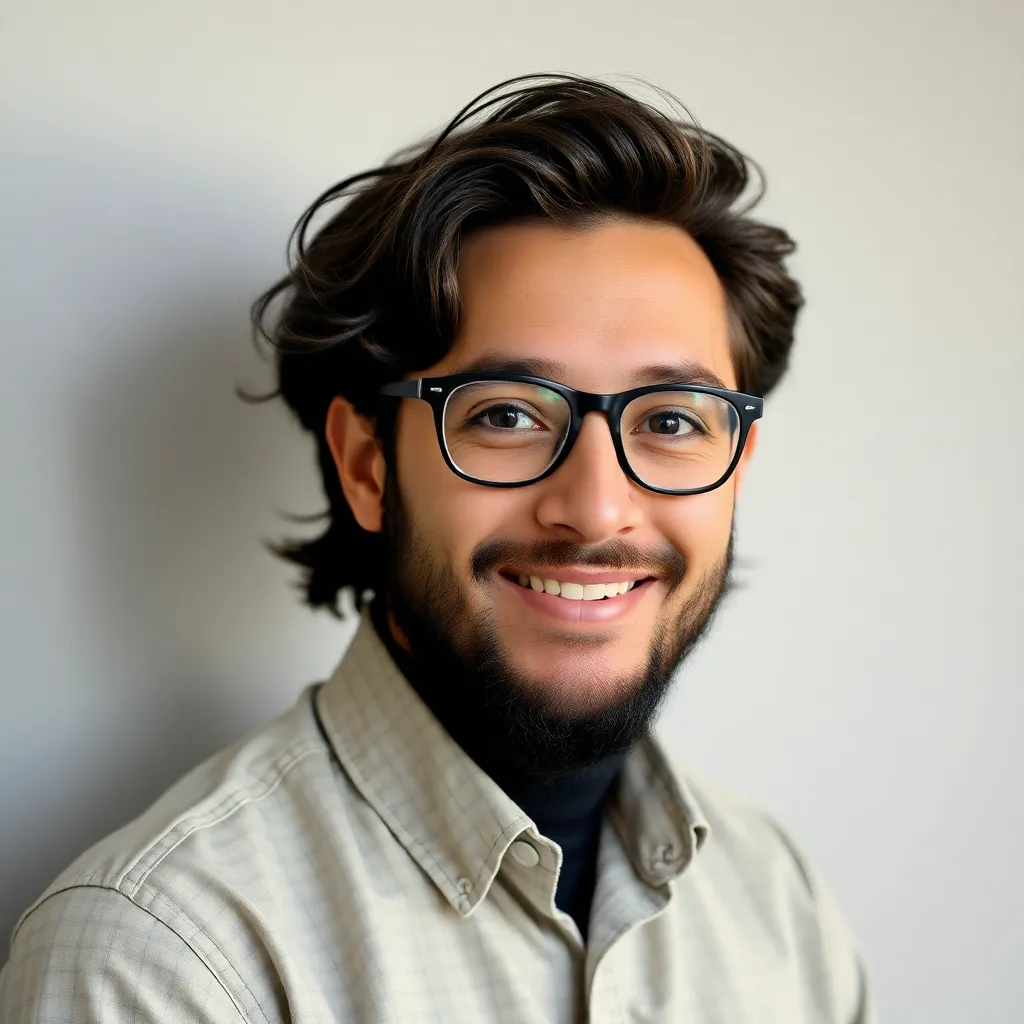
Juapaving
May 23, 2025 · 6 min read
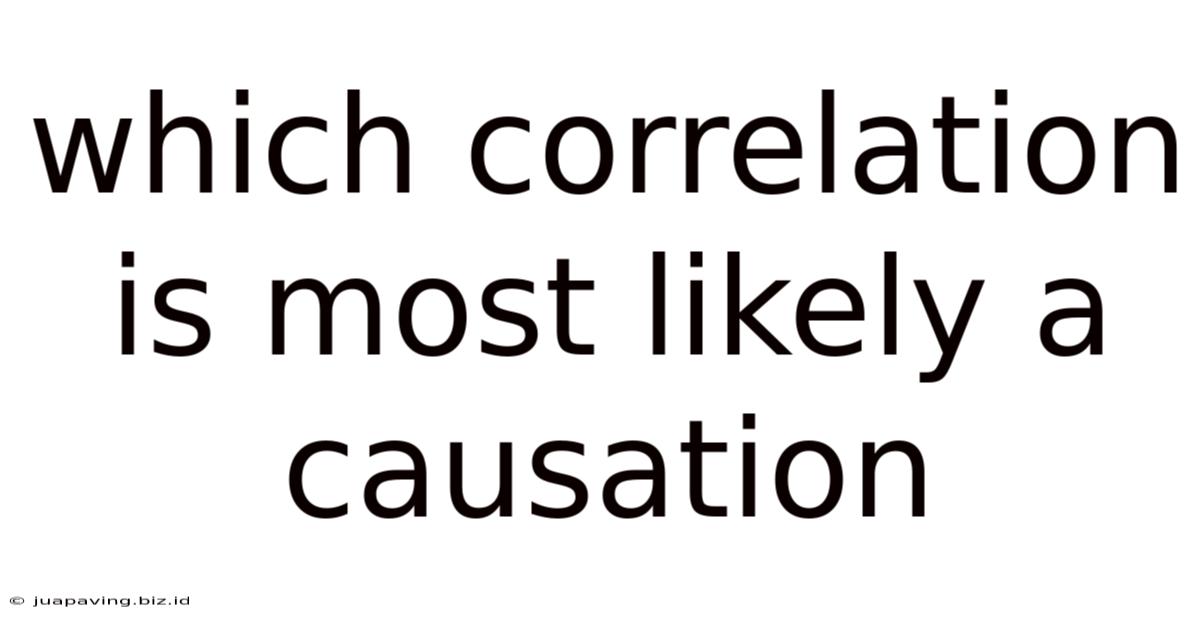
Table of Contents
Which Correlation is Most Likely a Causation? Unraveling the Mysteries of Correlation vs. Causation
Understanding the difference between correlation and causation is crucial for critical thinking and effective decision-making, especially in fields like data science, epidemiology, and social sciences. While a correlation indicates a relationship between two variables, it doesn't automatically imply that one variable causes the other. Many factors can create a correlation without a causal link. This article delves into the nuances of correlation and causation, providing strategies to identify which correlations are most likely to represent true causal relationships.
Correlation vs. Causation: A Fundamental Distinction
A correlation describes a statistical association between two or more variables. When a correlation exists, changes in one variable tend to be accompanied by changes in the other. This relationship can be positive (both variables increase together), negative (one variable increases while the other decreases), or zero (no relationship).
Causation, on the other hand, implies a direct cause-and-effect relationship. One variable directly influences the other; a change in the causal variable leads to a change in the effect variable. It's important to remember that correlation does not equal causation. A strong correlation might exist without a causal link.
Why Correlation Doesn't Equal Causation
Several scenarios illustrate why a correlation doesn't automatically imply causation:
1. Confounding Variables: The Hidden Player
A confounding variable is a third, often unobserved, factor that influences both variables being studied, creating a spurious correlation. For example, ice cream sales and drowning incidents are often positively correlated. However, the underlying cause is likely the summer heat (the confounding variable), which leads to increased ice cream consumption and more swimming, resulting in more drownings. Neither ice cream consumption causes drowning nor vice versa.
2. Reverse Causation: The Chicken or the Egg Dilemma
Sometimes, the causal direction is reversed. The variable initially thought to be the effect might actually be the cause. Consider the relationship between self-esteem and academic achievement. High self-esteem might lead to better academic performance, but equally, academic success could boost self-esteem. The direction of causality isn't clear from the correlation alone.
3. Coincidence: Purely Random Chance
Sometimes, a correlation emerges purely by chance, especially with smaller datasets. Statistical significance testing helps mitigate this, but even statistically significant correlations might not represent true causal relationships, particularly in complex systems.
Identifying Likely Causal Relationships: A Multifaceted Approach
While definitively proving causation often requires rigorous experimentation (e.g., randomized controlled trials), several strategies can help assess the likelihood of a causal relationship based on correlational data:
1. Temporal Precedence: Cause Before Effect
A fundamental criterion for causality is temporal precedence. The presumed cause must precede the effect in time. If variable A is claimed to cause variable B, then A must occur before B. This seems obvious, but it's frequently overlooked. For instance, if you're studying the effect of a marketing campaign (A) on sales (B), the campaign must have launched before the observed increase in sales.
2. Strength of the Correlation: A Stronger Signal
A stronger correlation suggests a higher probability of a causal relationship, though it's not definitive proof. A correlation coefficient closer to +1 (positive) or -1 (negative) indicates a stronger association. However, a strong correlation can still be spurious due to confounding variables.
3. Consistency: Replication Across Studies
If a correlation is consistently observed across multiple independent studies using different methodologies and populations, it strengthens the case for a causal link. Replication bolsters the reliability and generalizability of the findings, reducing the likelihood of random chance or methodological artifacts.
4. Dose-Response Relationship: A Graded Effect
A dose-response relationship provides further evidence of causation. If the magnitude of the effect increases with the intensity of the cause, it suggests a stronger causal link. For instance, if smoking more cigarettes (cause) leads to a greater risk of lung cancer (effect), it strengthens the argument for a causal relationship.
5. Biological Plausibility: Making Sense of the Mechanism
Evaluating the biological or theoretical plausibility of a causal mechanism adds significant weight to the argument. If the proposed causal link aligns with established biological or theoretical understanding, it enhances the credibility of the relationship. For example, understanding the mechanisms by which smoking damages lung tissue supports the causal link between smoking and lung cancer.
6. Consideration of Alternative Explanations: Ruling Out Other Possibilities
Thoroughly examining and ruling out alternative explanations for the observed correlation is vital. This involves identifying and controlling for potential confounding variables and considering reverse causation. Rigorous analysis aims to eliminate competing hypotheses.
7. Mechanism Identification: Unveiling the "How"
Understanding the underlying mechanism linking the cause and effect strengthens the causal argument significantly. For example, demonstrating how a specific gene mutation (cause) leads to a particular disease (effect) through a series of biochemical processes provides strong evidence of causation.
8. Experimental Evidence: The Gold Standard
The most compelling evidence for causation comes from well-designed experiments, particularly randomized controlled trials (RCTs). RCTs involve randomly assigning participants to different groups (e.g., treatment and control) and measuring the effect of the intervention. Randomization helps control for confounding variables, making it more likely that any observed difference between groups is due to the intervention.
Examples Illustrating the Application of These Principles
Let's examine a few examples to see how these principles can be applied in practice:
Example 1: Vaccination and Disease Prevention
The correlation between vaccination and reduced incidence of infectious diseases is extremely strong and consistent across numerous studies. Temporal precedence is clear (vaccination precedes disease reduction), there's a dose-response relationship (higher vaccination rates lead to lower disease rates), the mechanism is well understood (vaccination induces immunity), and numerous randomized controlled trials have demonstrated effectiveness. These factors strongly suggest a causal relationship.
Example 2: Exercise and Cardiovascular Health
Observational studies reveal a strong negative correlation between regular exercise and the risk of cardiovascular disease. While not definitive proof of causation, the temporal precedence is evident, many studies support the correlation, a plausible mechanism exists (exercise improves cardiovascular fitness), and a dose-response relationship (more exercise leads to lower risk) is observed. These points strongly suggest a causal relationship, further strengthened by experimental studies showing the benefits of exercise interventions.
Example 3: Coffee Consumption and Longevity
Some studies show a correlation between coffee consumption and increased longevity. However, while intriguing, this correlation is weaker than the previous examples. Confounding variables, such as lifestyle choices often associated with coffee consumption (e.g., healthier diet, more social interaction), could contribute to this association. Further research is needed to determine the extent to which this correlation reflects a true causal relationship, and more research on underlying mechanisms are needed to strengthen the causal argument.
Conclusion: The Ongoing Pursuit of Causal Understanding
Determining whether a correlation indicates causation is a complex process that requires careful consideration of multiple factors. While a single correlation rarely provides conclusive proof of causation, a combination of strong correlation, temporal precedence, a plausible mechanism, consistent findings across studies, and, ideally, experimental evidence significantly strengthens the case. By applying these principles judiciously, we can move beyond simple correlations towards a more profound understanding of cause-and-effect relationships in the world around us. Remember, critical thinking and a rigorous approach are crucial when evaluating causal claims, especially those based solely on correlational data.
Latest Posts
Latest Posts
-
Chapter 3 Of The Scarlet Letter
May 25, 2025
-
What Is An Interrogative Word In Spanish
May 25, 2025
-
Chapter 1 The Things They Carried
May 25, 2025
-
Summary Of Chapter 12 Catcher In The Rye
May 25, 2025
-
Which Of The Following Is An Example Of Confirmation Bias
May 25, 2025
Related Post
Thank you for visiting our website which covers about Which Correlation Is Most Likely A Causation . We hope the information provided has been useful to you. Feel free to contact us if you have any questions or need further assistance. See you next time and don't miss to bookmark.