What Is The Difference Between Theoretical And Experimental Probability
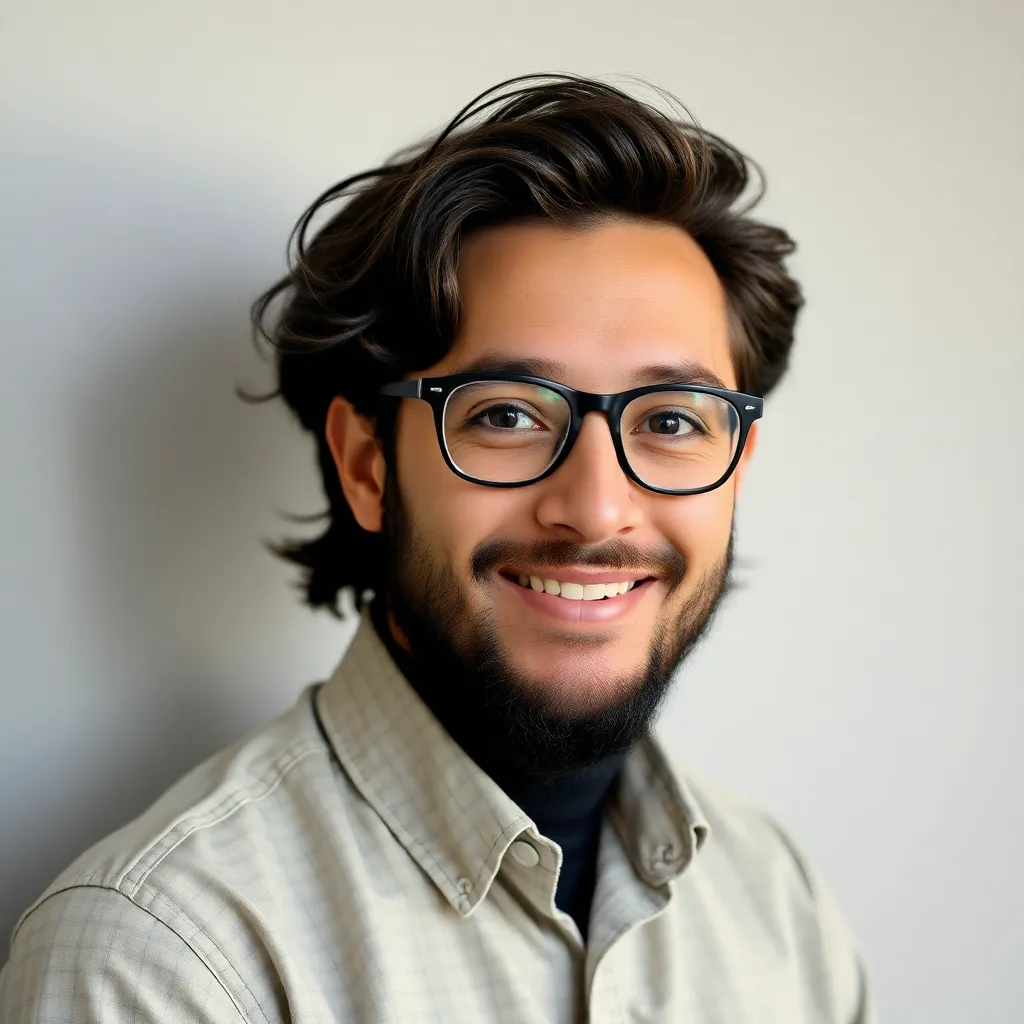
Juapaving
May 10, 2025 · 6 min read
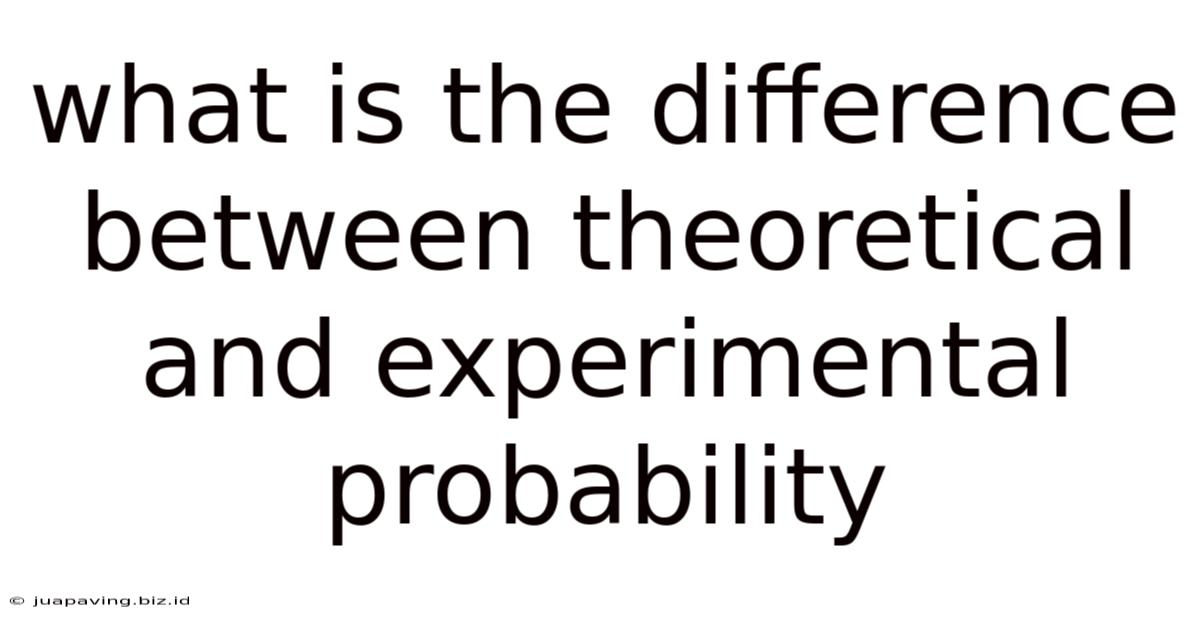
Table of Contents
What's the Difference Between Theoretical and Experimental Probability?
Understanding probability is crucial in many fields, from gambling and insurance to weather forecasting and medical research. However, the concept itself can be nuanced, and a key distinction lies between theoretical and experimental probability. While both aim to quantify the likelihood of an event, their approaches and results can differ significantly. This comprehensive guide will delve into the differences between these two types of probability, highlighting their applications and limitations.
Theoretical Probability: A World of Idealized Outcomes
Theoretical probability, also known as classical probability, relies on logical reasoning and prior knowledge about the event's potential outcomes. It's based on the assumption that all possible outcomes are equally likely. We calculate theoretical probability using the following formula:
P(A) = Number of favorable outcomes / Total number of possible outcomes
Where P(A) represents the probability of event A.
Let's illustrate this with an example. Consider flipping a fair coin. The total number of possible outcomes is two: heads or tails. If we want to determine the theoretical probability of getting heads, we have:
P(Heads) = 1 (favorable outcome: heads) / 2 (total outcomes: heads and tails) = 0.5 or 50%
This tells us that, theoretically, there's a 50% chance of getting heads when flipping a fair coin. This is based on our understanding of a fair coin – that both heads and tails are equally likely.
Advantages of Theoretical Probability:
- Simplicity: It's relatively straightforward to calculate, especially for simple events with clearly defined outcomes.
- Predictability: It provides a clear and concise prediction of the likelihood of an event before any experiments are conducted.
- Basis for Comparison: It provides a benchmark against which experimental results can be compared to assess the accuracy of the experiment or the fairness of the process.
Limitations of Theoretical Probability:
- Assumption of Equal Likelihood: The biggest limitation is its reliance on the assumption that all outcomes are equally likely. In reality, many events don't adhere to this assumption. For instance, predicting the weather isn't as simple as calculating theoretical probabilities.
- Unrealistic for Complex Events: Calculating theoretical probability becomes increasingly complex, even impossible, for events with a large number of possible outcomes or those involving interdependent factors. Imagine trying to calculate the theoretical probability of winning a lottery.
- Ignoring Real-World Factors: Theoretical probability often ignores real-world factors that can influence the outcome, such as bias, external interference, or changing conditions.
Experimental Probability: Learning from Real-World Data
Experimental probability, also known as empirical probability, relies on observing the outcomes of repeated trials of an experiment. It's a data-driven approach that measures the frequency of an event's occurrence over a series of trials. We calculate experimental probability using the following formula:
P(A) = Number of times event A occurred / Total number of trials
Let's revisit the coin flip example. Suppose we flip a coin 100 times and observe that heads appears 48 times. The experimental probability of getting heads would be:
P(Heads) = 48 (number of heads) / 100 (total number of flips) = 0.48 or 48%
This result differs from the theoretical probability of 50%. This discrepancy can be attributed to random variation inherent in any experiment.
Advantages of Experimental Probability:
- Real-World Relevance: It provides a practical estimate of probability based on actual observations, making it particularly useful when theoretical calculations are difficult or impossible.
- Handles Complexities: It can accommodate events with numerous outcomes or interdependent factors that are difficult to model theoretically. Consider determining the probability of a particular disease based on a large dataset of patients.
- Adaptability: Experimental probability can be updated as more data becomes available, refining the probability estimate over time.
Limitations of Experimental Probability:
- Dependence on Sample Size: The accuracy of experimental probability heavily relies on the sample size. A small sample size can lead to a biased or inaccurate probability estimate. Larger sample sizes generally yield more reliable results.
- Random Variation: Experimental probability is always subject to random variation. Repeated experiments with the same conditions might produce slightly different results.
- Time and Resource Intensive: Collecting sufficient data for complex events can be time-consuming and resource-intensive.
Key Differences Summarized:
Feature | Theoretical Probability | Experimental Probability |
---|---|---|
Basis | Logical reasoning and prior knowledge | Observed outcomes of repeated trials |
Calculation | Number of favorable outcomes / Total possible outcomes | Number of times event occurred / Total number of trials |
Assumptions | Equally likely outcomes | None (except for random sampling in good experimental design) |
Accuracy | High for simple events, low for complex events | Dependent on sample size; improves with larger samples |
Applications | Simple events with clearly defined outcomes | Complex events, real-world situations |
Limitations | Assumes equally likely outcomes; struggles with complex events | Requires large sample sizes; susceptible to random variation |
Bridging the Gap: Law of Large Numbers
The Law of Large Numbers states that as the number of trials in an experiment increases, the experimental probability will converge towards the theoretical probability. In other words, with enough trials, the observed frequency of an event will get closer and closer to its true probability. This principle underlines the importance of large sample sizes in experimental probability.
Applications in Different Fields:
1. Medicine: Experimental probability is essential in clinical trials to assess the effectiveness of new drugs or treatments. The results from a large number of patients are analyzed to determine the probability of success or the likelihood of adverse effects. Theoretical probability might be used to design the trial and estimate sample size requirements.
2. Insurance: Insurance companies rely heavily on probability to assess risks and set premiums. They use historical data (experimental probability) to estimate the likelihood of certain events like car accidents or house fires. They may also use theoretical probabilities based on statistical models.
3. Weather Forecasting: Weather forecasting uses a blend of theoretical and experimental probability. Theoretical models based on atmospheric physics predict weather patterns, while experimental data from observations (satellite imagery, ground stations) refine these predictions.
4. Gambling: In games of chance, theoretical probability can calculate the odds of winning. Casinos utilize these calculations to establish house advantages. However, experimental probability is essential to analyze actual game outcomes and detect patterns or deviations.
5. Sports Analytics: Sports analytics uses experimental probability extensively. Data on players' past performance is analyzed to predict future outcomes, such as the probability of a player scoring a goal or a team winning a game. Advanced statistical models may incorporate theoretical probabilities as well.
Conclusion:
Both theoretical and experimental probability are valuable tools for understanding and quantifying likelihood. Theoretical probability provides a foundational understanding for simple events, while experimental probability is crucial for real-world applications involving complex events and limited prior knowledge. A strong understanding of both types of probability, combined with an awareness of their limitations and strengths, is essential for effective decision-making across a wide range of fields. The Law of Large Numbers bridges the gap, illustrating how experimental probability approaches theoretical probability with sufficient data, highlighting the power of large sample sizes in refining our understanding of chance.
Latest Posts
Latest Posts
-
In Which Plant Cell Organelle Does Photosynthesis Take Place
May 10, 2025
-
What Is Prime Factorization Of 360
May 10, 2025
-
The Clear Sky Appears Blue Because
May 10, 2025
-
Multiples Of 6 Up To 200
May 10, 2025
-
Compare And Contrast Alcoholic And Lactic Acid Fermentation
May 10, 2025
Related Post
Thank you for visiting our website which covers about What Is The Difference Between Theoretical And Experimental Probability . We hope the information provided has been useful to you. Feel free to contact us if you have any questions or need further assistance. See you next time and don't miss to bookmark.