What Inference Can Be Drawn From The Graph
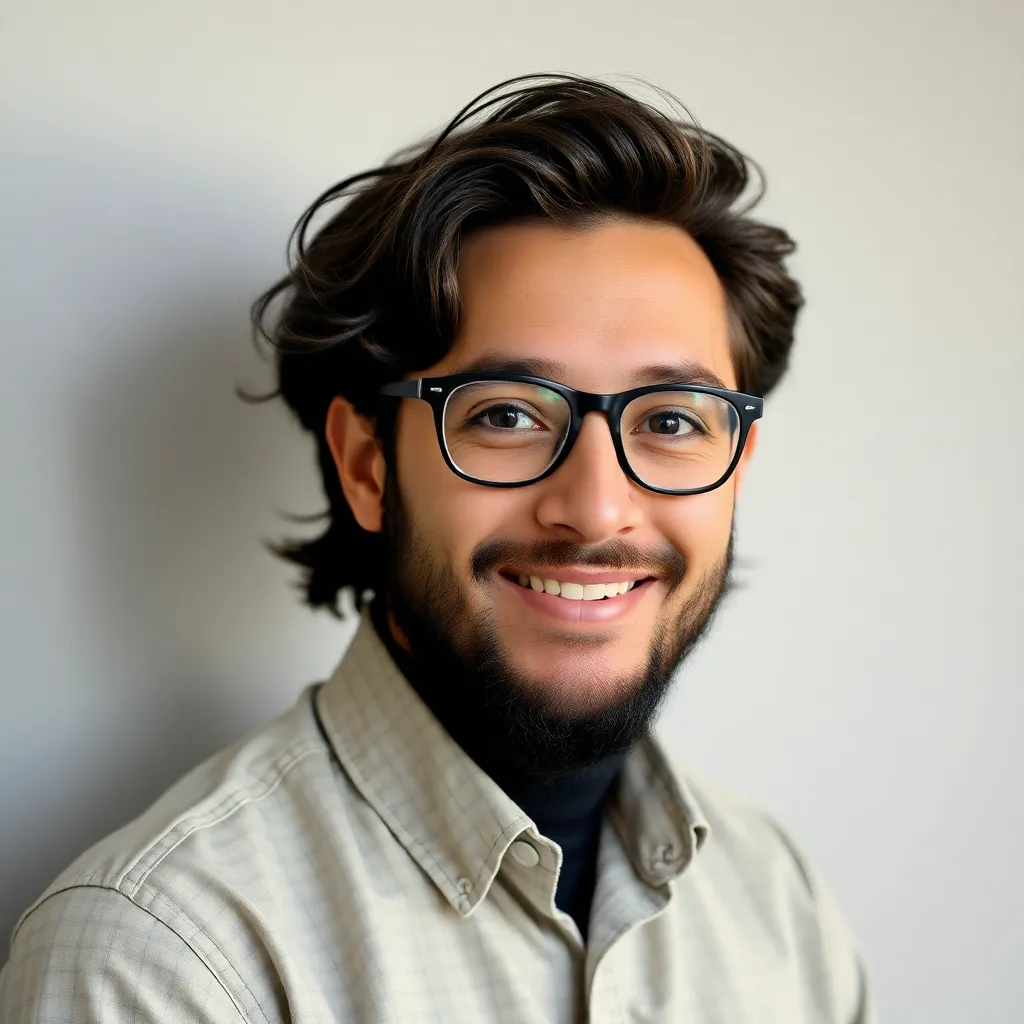
Juapaving
May 12, 2025 · 7 min read
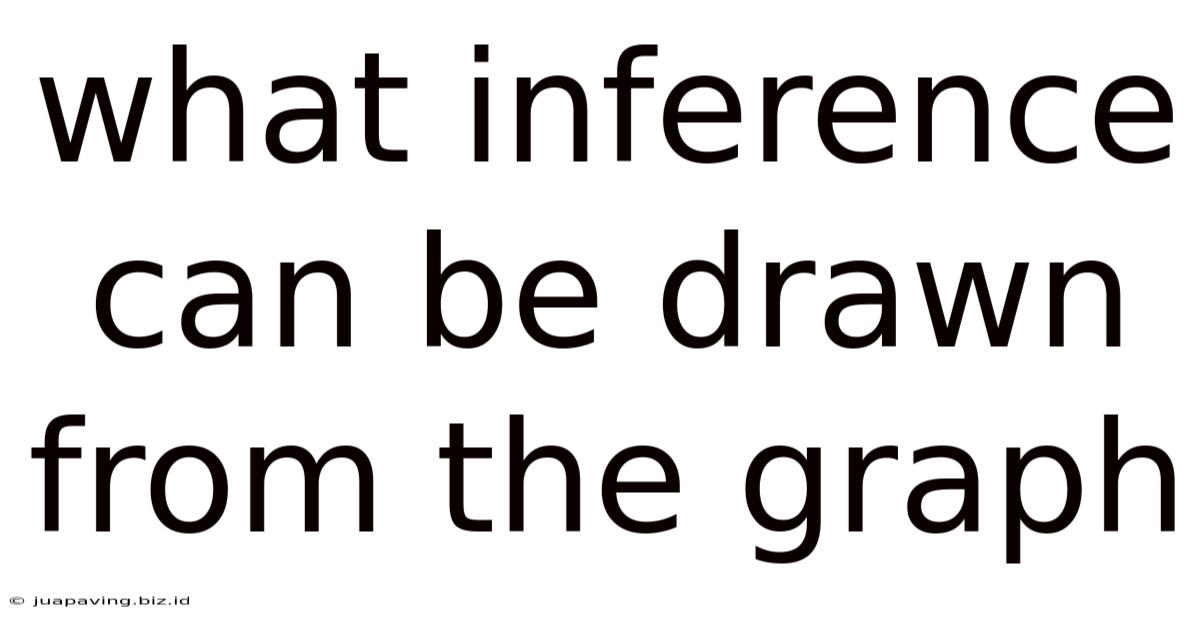
Table of Contents
What Inferences Can Be Drawn From a Graph? A Comprehensive Guide
Graphs are powerful visual tools used to represent data and relationships. They allow us to quickly identify trends, patterns, and outliers that might be missed when looking at raw data alone. However, simply looking at a graph isn't enough; we need to understand how to draw meaningful inferences from the information presented. This article will delve into various aspects of graph interpretation, providing you with the skills to extract valuable insights and communicate your findings effectively.
Understanding Different Graph Types
Before we discuss inference, it’s crucial to understand the various types of graphs and their strengths. Different graph types are best suited for different types of data and relationships. Choosing the right graph is the first step towards accurate interpretation.
1. Line Graphs: Showing Trends Over Time
Line graphs are ideal for displaying data that changes over time. They're excellent for illustrating growth, decline, or cyclical patterns. Key inferences drawn from line graphs often involve:
- Trends: Is the data increasing, decreasing, or remaining relatively stable? Are there periods of rapid change or stagnation?
- Rate of Change: How quickly is the data changing? Is the rate of change constant, accelerating, or decelerating?
- Turning Points: Are there points where the trend changes direction (e.g., peaks and troughs)? What factors might have contributed to these changes?
- Comparisons: Can you compare multiple lines on the same graph to identify differences in trends or rates of change?
Example: A line graph showing website traffic over a year could reveal peak periods (perhaps around holidays) and identify times when marketing campaigns were successful or unsuccessful.
2. Bar Charts: Comparing Categories
Bar charts are effective for comparing different categories of data. The length of each bar represents the value of the category. Key inferences include:
- Magnitude Comparisons: Which category has the highest/lowest value? What is the difference between the highest and lowest values?
- Proportions: What percentage of the total does each category represent?
- Rankings: How do the categories rank relative to each other?
- Outliers: Are there any categories that are significantly different from the others?
Example: A bar chart comparing sales figures for different product lines can reveal which products are most popular and which ones need improvement.
3. Pie Charts: Showing Proportions
Pie charts are designed to show the proportion of each category relative to the whole. The size of each slice represents its proportion. Key inferences often focus on:
- Relative Proportions: What percentage of the total does each category represent? Which categories are dominant, and which are minor?
- Comparisons: How do the proportions of different categories compare to each other?
- Changes Over Time (with Multiple Pie Charts): Comparing multiple pie charts for different time periods can show how proportions have shifted.
Example: A pie chart illustrating the age demographics of a website's visitors can highlight the target audience.
4. Scatter Plots: Identifying Correlations
Scatter plots display the relationship between two variables. Each point represents a data point with coordinates corresponding to the values of the two variables. Key inferences often revolve around:
- Correlation: Is there a positive, negative, or no correlation between the two variables? A positive correlation means that as one variable increases, the other also tends to increase. A negative correlation means that as one variable increases, the other tends to decrease. No correlation implies no relationship.
- Strength of Correlation: How strong is the correlation? A strong correlation means that the points cluster closely around a line, while a weak correlation means that the points are more scattered.
- Outliers: Are there any points that lie far from the general trend? These outliers could represent unusual data points that warrant further investigation.
- Linearity: Does the relationship between the variables appear to be linear (a straight line), or non-linear (a curve)?
Example: A scatter plot showing the relationship between hours of study and exam scores could reveal a positive correlation, suggesting that more study time is associated with higher scores.
5. Histograms: Showing Data Distribution
Histograms show the distribution of a single variable. Data is grouped into bins (intervals), and the height of each bar represents the frequency (number of data points) within that bin. Key inferences include:
- Central Tendency: Where is the center of the data located (mean, median, mode)?
- Spread/Dispersion: How spread out is the data? Measures like range, variance, and standard deviation can quantify this.
- Symmetry/Skewness: Is the distribution symmetrical (bell-shaped), or skewed (leaning to one side)? A skewed distribution might indicate that there are outliers or that the data is not normally distributed.
- Modality: How many peaks (modes) does the distribution have? A unimodal distribution has one peak, while a bimodal distribution has two.
Example: A histogram showing the distribution of student test scores can reveal whether the scores are clustered around the average, or if there's a significant number of high or low scores.
Drawing Inferences: A Step-by-Step Approach
Drawing accurate inferences from a graph requires a systematic approach:
-
Understand the Context: Before analyzing the graph, understand the data it represents and the questions you're trying to answer. What are the variables being measured? What is the time period or population being considered?
-
Identify the Key Features: Note the overall trends, patterns, and any significant data points (outliers). Look for peaks, valleys, clusters, and gaps in the data.
-
Quantify the Observations: Whenever possible, quantify your observations using numerical data. For example, instead of saying "sales increased," say "sales increased by 15%."
-
Consider Potential Biases: Be aware of potential biases in the data or the way the graph is presented. For example, the scale of the axes can influence the perceived trends.
-
Formulate Hypotheses: Based on your observations, formulate hypotheses to explain the patterns you've identified. These hypotheses should be testable and falsifiable.
-
Consider External Factors: Don't just focus on the data presented in the graph. Consider external factors that might have influenced the results. For example, economic conditions, seasonal variations, or policy changes could all affect the data.
-
Draw Conclusions: Based on your analysis, draw conclusions about the data and its implications. Be cautious about making generalizations that go beyond the scope of the data.
-
Communicate Your Findings Clearly: Communicate your findings clearly and concisely using both verbal and visual methods. Use clear and concise language, and support your conclusions with evidence from the graph.
Common Errors in Graph Interpretation
Several common errors can lead to inaccurate inferences:
- Ignoring Context: Failing to understand the context of the data can lead to misinterpretations.
- Misinterpreting Correlations: Correlation doesn't necessarily imply causation. Just because two variables are correlated doesn't mean that one causes the other.
- Overgeneralizing: Making generalizations beyond the scope of the data.
- Ignoring Outliers: Outliers can provide valuable information, and ignoring them can lead to inaccurate conclusions.
- Poorly Designed Graphs: Misleading graphs can distort the data and lead to inaccurate conclusions.
Advanced Techniques for Graph Interpretation
For more complex graphs, several advanced techniques can be used:
- Regression Analysis: This statistical technique can be used to model the relationship between two or more variables and make predictions.
- Time Series Analysis: This technique is used to analyze data collected over time and identify trends, seasonality, and other patterns.
- Multivariate Analysis: This technique is used to analyze data with multiple variables simultaneously.
Conclusion
Drawing accurate inferences from a graph is a crucial skill for anyone working with data. By understanding the different types of graphs, employing a systematic approach, and being aware of potential errors, you can extract valuable insights and communicate your findings effectively. Remember to always consider the context, quantify your observations, and avoid making assumptions that go beyond the data. With practice and a keen eye for detail, you can become a proficient interpreter of graphical data and harness its power to make informed decisions.
Latest Posts
Latest Posts
-
Mass Of A Neutron In Grams
May 12, 2025
-
Which Of The Following Statements About Genetic Diversity Is True
May 12, 2025
-
How Many Zeros Are In 8 Million
May 12, 2025
-
What Is The Difference Between A Woman And A Lady
May 12, 2025
-
Unit Of Second Order Rate Constant
May 12, 2025
Related Post
Thank you for visiting our website which covers about What Inference Can Be Drawn From The Graph . We hope the information provided has been useful to you. Feel free to contact us if you have any questions or need further assistance. See you next time and don't miss to bookmark.