Using The Count Data And Observational Data
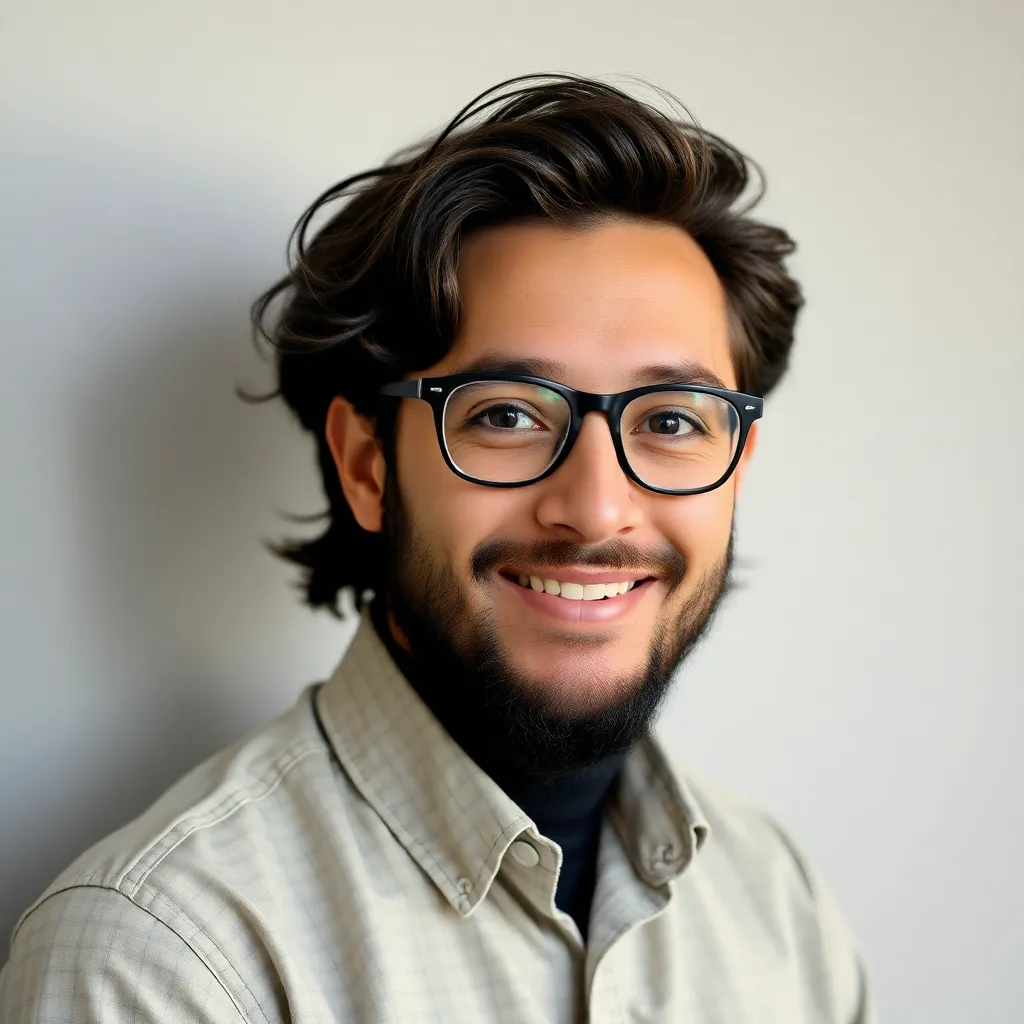
Juapaving
May 24, 2025 · 6 min read

Table of Contents
Delving into the Depths: Utilizing Count Data and Observational Data in Research
Analyzing data is the bedrock of research, forming the foundation upon which conclusions are built and hypotheses tested. Two crucial data types often encountered in research are count data and observational data. Understanding their unique characteristics and appropriate analytical techniques is vital for drawing accurate and meaningful inferences. This article will delve into the intricacies of both, exploring their applications, potential pitfalls, and best practices for analysis.
Understanding Count Data: More Than Just Numbers
Count data represents the number of times an event occurs within a given timeframe or specific context. It's inherently discrete, meaning it can only take on whole number values (0, 1, 2, 3, and so on). Unlike continuous data (e.g., height, weight), count data can't be meaningfully subdivided. Examples abound across numerous fields:
- Epidemiology: Number of new COVID-19 cases per day in a city.
- Ecology: Number of bird nests found in a particular forest area.
- Marketing: Number of website clicks generated by an advertising campaign.
- Finance: Number of defaults on loans within a specific portfolio.
The unique characteristic of count data is its often non-normal distribution. Standard statistical tests that assume normality (like t-tests or ANOVA) are inappropriate. Instead, specialized techniques are required to analyze count data effectively.
Common Distributions for Count Data:
Several probability distributions are commonly used to model count data, depending on the specific characteristics of the data:
-
Poisson Distribution: This distribution is suitable when the events are independent and occur at a constant average rate. It's characterized by a single parameter, λ (lambda), representing the average rate of occurrence. The Poisson distribution is often a good starting point for analyzing count data.
-
Negative Binomial Distribution: This distribution is a generalization of the Poisson distribution, accounting for overdispersion—where the variance is greater than the mean. Overdispersion frequently occurs in real-world count data due to unobserved heterogeneity or clustering. The negative binomial distribution incorporates an additional parameter to capture this extra variability.
-
Zero-Inflated Poisson (ZIP) and Zero-Inflated Negative Binomial (ZINB) Distributions: These distributions are used when there's an excess of zeros in the count data compared to what a standard Poisson or negative binomial distribution would predict. This excess of zeros might be due to structural zeros (e.g., individuals who are inherently incapable of experiencing the event) or sampling zeros (e.g., sampling error leading to zero counts).
Analyzing Count Data: Techniques and Considerations:
Appropriate statistical methods for count data include:
-
Poisson Regression: This model predicts the expected count of events based on predictor variables. It's a valuable tool for exploring relationships between explanatory variables and the count outcome.
-
Negative Binomial Regression: Similar to Poisson regression but specifically designed for count data exhibiting overdispersion.
-
Zero-Inflated Regression Models: These models explicitly account for excess zeros in the data. Choosing between ZIP and ZINB depends on the presence of overdispersion.
-
Generalized Estimating Equations (GEE): GEE is particularly useful when dealing with clustered or correlated count data (e.g., multiple observations from the same individual).
Important Considerations:
-
Data Transformation: Transforming count data (e.g., taking the square root or log) is generally discouraged because it can distort the underlying probability distribution and lead to misleading inferences.
-
Model Selection: Careful model selection is crucial. Start with a simpler model (e.g., Poisson regression) and check for model assumptions (e.g., overdispersion). If assumptions are violated, consider a more complex model (e.g., negative binomial regression).
-
Interpretation: Interpreting the results of count data analysis requires careful consideration of the model parameters and their statistical significance. Focus on the practical implications of the findings.
Understanding Observational Data: Capturing Reality as It Is
Observational data is collected by observing subjects without manipulating any variables. Unlike experimental data, where researchers actively intervene, observational data reflects the natural occurrence of events. This type of data is prevalent in many research fields, particularly in situations where controlled experiments are unethical or impossible.
Examples of observational data include:
- Epidemiology: Observing the prevalence of a disease in different populations.
- Sociology: Studying the relationship between social media usage and mental health.
- Economics: Analyzing the impact of government policies on economic growth.
- Environmental Science: Monitoring changes in wildlife populations over time.
Types of Observational Studies:
Observational studies can be broadly categorized into:
-
Cross-sectional studies: Data is collected at a single point in time, providing a snapshot of the variables of interest.
-
Cohort studies: A group of individuals (the cohort) is followed over time, observing the occurrence of events of interest.
-
Case-control studies: Individuals with a particular outcome (cases) are compared to a control group without the outcome, investigating potential risk factors.
Challenges and Biases in Observational Data:
Observational data is prone to several biases, including:
-
Selection bias: This occurs when the sample is not representative of the population of interest.
-
Confounding bias: This arises when a third variable influences both the exposure and the outcome, obscuring the true relationship.
-
Information bias: This occurs when there are inaccuracies or inconsistencies in the data collection process.
Analyzing Observational Data: Methods and Strategies:
Analyzing observational data often involves statistical techniques designed to address potential biases and confounding factors:
-
Regression analysis: This is a powerful tool for exploring relationships between variables, controlling for confounders. Multiple regression can accommodate multiple predictor variables.
-
Propensity score matching: This technique aims to reduce selection bias by matching individuals with similar characteristics but differing exposure status.
-
Instrumental variables: This approach is used to address confounding bias when a direct causal relationship cannot be easily established.
-
Causal inference methods: Techniques like directed acyclic graphs (DAGs) and causal diagrams can help researchers map out potential causal relationships and account for confounding.
Important Considerations:
-
Careful Study Design: A well-designed observational study is crucial to minimizing bias and improving the validity of the conclusions. Careful consideration of sampling methods, data collection protocols, and potential confounding factors is paramount.
-
Robust Statistical Methods: Selecting appropriate statistical methods is essential to address potential biases and draw valid inferences.
-
Interpretation of Results: Interpreting findings from observational studies requires caution. Observational data does not establish causality; it only reveals associations. Carefully consider the limitations of the study and avoid overgeneralizing the results. Clearly state that correlation does not equal causation.
Combining Count Data and Observational Data: A Powerful Synergy
Many research questions involve both count data and observational data. For instance, researchers might observe the number of hospital readmissions (count data) in a group of patients with various characteristics (observational data) to determine factors influencing readmission rates. In this scenario, combining both data types allows for a richer and more nuanced analysis.
A common approach is to integrate count data into a broader observational study design. For example, a cohort study could track the number of events (count data) experienced by individuals over time, while also collecting information about their demographics, lifestyle, and other relevant variables (observational data). Analyzing this combined data allows for a comprehensive understanding of the factors contributing to the observed event counts.
Conclusion: Harnessing the Power of Data
Understanding the distinct characteristics of count data and observational data, along with their appropriate analytical techniques, is paramount for conducting robust research. While both data types offer valuable insights, they also present unique challenges. Careful study design, appropriate statistical methods, and cautious interpretation are crucial for obtaining meaningful and reliable conclusions. By mastering these approaches, researchers can effectively leverage both count data and observational data to unravel complex research questions and contribute meaningfully to their respective fields. Remember to always prioritize rigorous methodology and responsible interpretation to ensure the integrity and impact of your research.
Latest Posts
Latest Posts
-
The Highlighted Openings Are A Component Of Which Bones
May 24, 2025
-
Twelfth Night Act 3 Scene 2
May 24, 2025
-
Chapter 23 The Catcher In The Rye
May 24, 2025
-
Pal Cadaver Axial Skeleton Vertebral Column Lab Practical Question 9
May 24, 2025
-
Theme For Of Mice And Men
May 24, 2025
Related Post
Thank you for visiting our website which covers about Using The Count Data And Observational Data . We hope the information provided has been useful to you. Feel free to contact us if you have any questions or need further assistance. See you next time and don't miss to bookmark.