Time Series Data May Exhibit Which Of The Following Behaviors
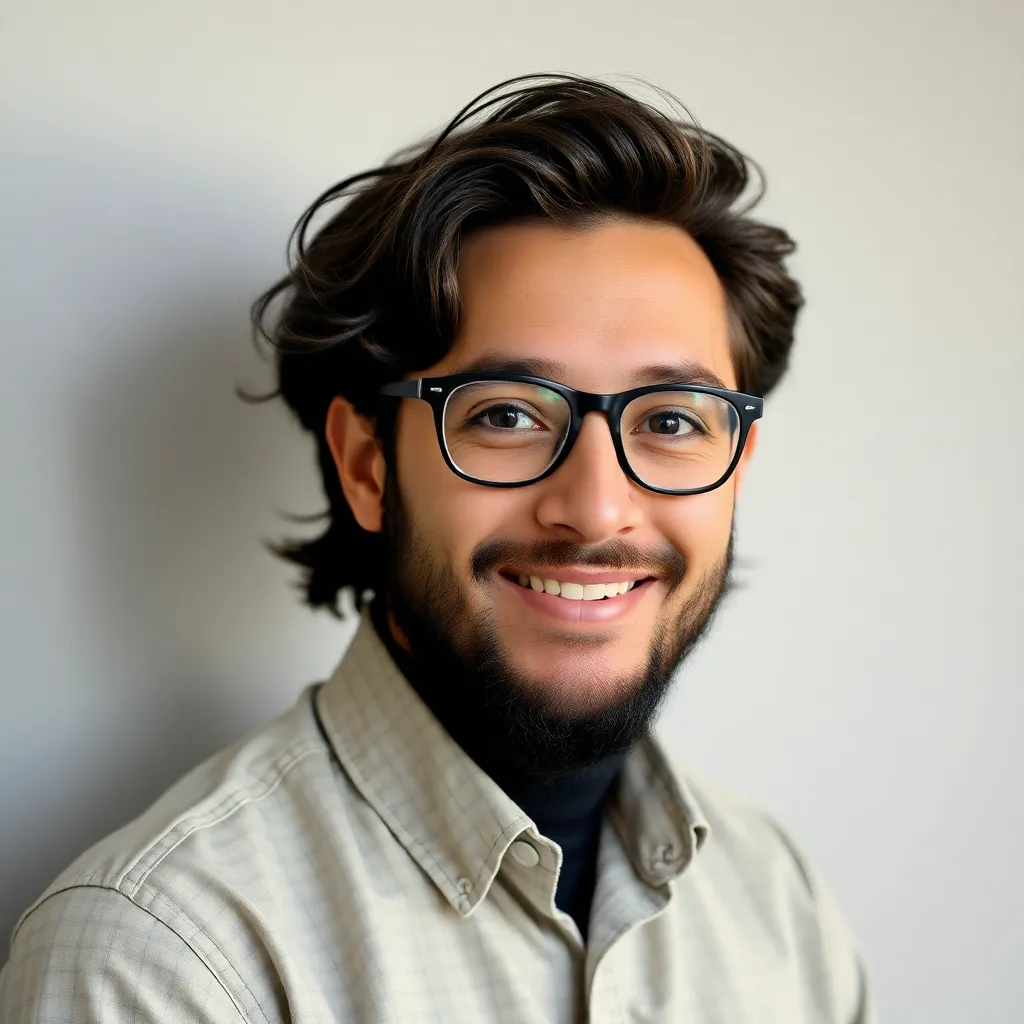
Juapaving
May 24, 2025 · 6 min read
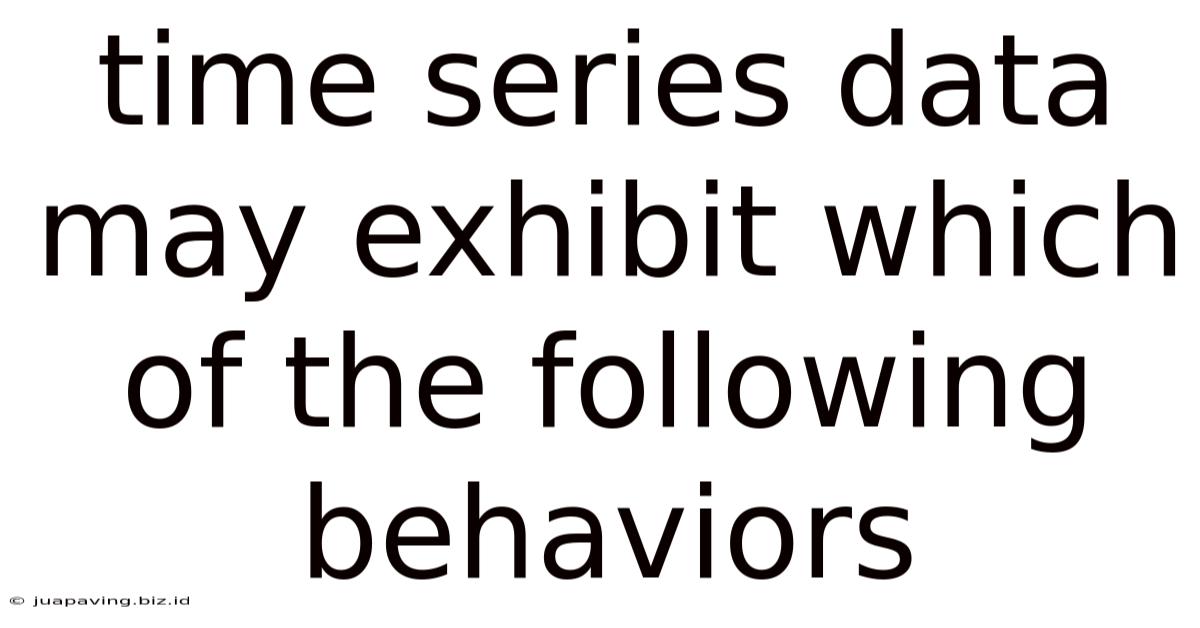
Table of Contents
Time Series Data: Unmasking its Behavioral Quirks
Time series data, a sequential collection of data points indexed in time order, is ubiquitous across numerous fields. From financial markets charting stock prices to climate science tracking temperatures, understanding the inherent behaviors of time series data is crucial for accurate analysis and forecasting. This comprehensive guide delves into the diverse characteristics that define time series data, providing a deep understanding of the patterns and anomalies you might encounter.
Key Behaviors of Time Series Data
Time series data, unlike cross-sectional data, possesses a unique temporal dependency. This dependency introduces several characteristic behaviors which must be carefully considered during analysis. Let's explore some of the most prevalent:
1. Trends
A trend represents a long-term pattern of increase or decrease in the data. This gradual shift can be linear, indicating a constant rate of change, or non-linear, showcasing an accelerating or decelerating trend. Identifying trends is crucial for understanding the underlying forces driving the time series. For example, a steadily increasing trend in global temperatures strongly suggests a long-term climate change pattern.
- Linear Trend: A straight line best represents the overall direction of the data. Imagine the steady growth of a company's revenue over many years.
- Non-linear Trend: The relationship isn't easily represented by a straight line. Consider exponential growth in technology adoption, or the S-curve representing the lifecycle of a product.
- Identifying Trends: Techniques like linear regression, moving averages, and exponential smoothing can effectively identify and quantify trends within time series data.
2. Seasonality
Seasonality refers to repetitive patterns that occur at fixed intervals within a time series. These patterns often reflect cyclical influences like weather patterns, holiday sales, or daily or weekly routines. Understanding seasonality is critical for accurate forecasting, as ignoring it can lead to significant errors.
- Identifying Seasonality: Techniques like autocorrelation and spectral analysis are valuable tools for identifying seasonal components. Visual inspection of time series plots often reveals repeating patterns. Decomposition methods separate seasonal components from the overall trend.
- Examples of Seasonality: The peak demand for ice cream during summer months, higher retail sales during the holiday season, or increased website traffic during weekdays are all examples of seasonality.
3. Cyclicity
While similar to seasonality, cyclicity refers to longer-term, less predictable patterns that don't follow a fixed period. Unlike seasonal fluctuations which are consistent, cyclical variations are irregular and often more challenging to predict.
- Examples of Cyclicity: Economic cycles (e.g., business cycles of expansion and recession), long-term climate oscillations (e.g., El Niño-Southern Oscillation), or even sunspot cycles showcase cyclic behaviors.
- Identifying Cyclicity: Advanced techniques like wavelet transforms and spectral analysis are often needed to identify and model cyclical components in time series data. These methods can reveal hidden periodicities and oscillations.
4. Randomness/Noise
Randomness or noise represents unpredictable fluctuations in the data. It's the portion of the time series that cannot be explained by trends, seasonality, or cyclical patterns. Noise can stem from measurement errors, unforeseen events, or inherent stochasticity in the system being measured.
- Dealing with Noise: Smoothing techniques like moving averages help reduce the impact of noise, revealing underlying patterns more clearly. However, excessive smoothing can obscure important details.
- The Importance of Noise Analysis: Understanding the nature and level of noise is crucial for assessing the accuracy of forecasting models and for understanding the limitations of any predictions.
5. Autocorrelation
Autocorrelation, also known as serial correlation, measures the relationship between a data point and its lagged values. A high positive autocorrelation suggests that values closer in time tend to be more similar, while a negative autocorrelation indicates alternating patterns.
- Understanding Autocorrelation: Autocorrelation is crucial for understanding the temporal dependency within the time series. It influences the choice of appropriate modeling techniques.
- Measuring Autocorrelation: The autocorrelation function (ACF) and partial autocorrelation function (PACF) are statistical tools used to quantify and visualize autocorrelation at different lags.
6. Heteroscedasticity
Heteroscedasticity refers to the non-constant variance of the time series. In simpler terms, the variability of the data changes over time. This variation in volatility can influence forecasting accuracy and model selection.
- Consequences of Heteroscedasticity: Models that assume constant variance (homoscedasticity) may produce inaccurate forecasts if the data exhibits heteroscedasticity.
- Addressing Heteroscedasticity: Transformations like logarithmic transformations or weighted least squares regression can often mitigate the effects of heteroscedasticity.
7. Structural Breaks
Structural breaks or regime shifts represent sudden and significant changes in the statistical properties of a time series. These shifts can be caused by unforeseen events, policy changes, or other external factors that fundamentally alter the underlying data-generating process.
- Detecting Structural Breaks: Techniques like Chow tests or CUSUM tests are commonly used to detect the presence and timing of structural breaks. Visual inspection can also be helpful in identifying abrupt changes.
- Importance of Identifying Structural Breaks: Ignoring structural breaks can lead to inaccurate forecasting models that fail to adapt to the new regime.
8. Non-stationarity
Non-stationarity is a crucial characteristic indicating that the statistical properties of the time series (mean, variance, or autocorrelation) change over time. Many statistical models assume stationarity, and non-stationary data often requires pre-processing before analysis.
- Consequences of Non-stationarity: Applying stationary models to non-stationary data can lead to misleading results and inaccurate forecasts.
- Addressing Non-stationarity: Techniques like differencing (taking the difference between consecutive data points) or other transformations can help stabilize the time series and make it stationary.
Advanced Behavioral Aspects:
Beyond the fundamental behaviors outlined above, consider these more nuanced characteristics:
9. Jumps and Spikes
Jumps and spikes represent sudden, large deviations from the typical behavior of the time series. These are often caused by external shocks or outliers. Identifying and addressing these anomalies is vital for building robust forecasting models.
- Handling Jumps and Spikes: These often require specialized techniques like robust regression or outlier detection methods. Simply removing them might erase valuable information.
10. Regime Switching
Regime switching describes time series that transition between different states or regimes, each characterized by unique statistical properties. This behavior is common in economic and financial data, where transitions between boom and recession periods occur.
- Modeling Regime Switching: Hidden Markov models (HMMs) and other advanced techniques are used to model and forecast regime-switching behavior.
Implications for Time Series Analysis
Understanding these behavioral quirks is paramount for choosing the correct analytical techniques and building effective forecasting models. Ignoring these characteristics can lead to inaccurate forecasts and misinterpretations of the underlying data-generating process.
- Model Selection: The presence or absence of trends, seasonality, and other characteristics guides the selection of appropriate time series models (e.g., ARIMA, SARIMA, exponential smoothing).
- Data Pre-processing: Techniques like differencing, transformations, and outlier treatment are often necessary to prepare the data for analysis.
- Forecasting Accuracy: A thorough understanding of the data's behavior enhances forecasting accuracy and reliability.
Conclusion
The analysis of time series data is a rich and complex field. By understanding the various behaviors exhibited by time series, including trends, seasonality, cyclicity, randomness, autocorrelation, heteroscedasticity, structural breaks, non-stationarity, jumps, spikes, and regime switching, analysts can extract meaningful insights and build powerful forecasting models. This knowledge is fundamental to effective decision-making across diverse disciplines, from finance and economics to meteorology and epidemiology. The exploration of these characteristics forms the bedrock of accurate and insightful analysis, empowering professionals to navigate the intricate world of temporal data.
Latest Posts
Latest Posts
-
Interior Dimensions Of A 53 Trailer
May 24, 2025
-
How Many Chapters Are In A Wrinkle In Time
May 24, 2025
-
Chapter 11 All Quiet On The Western Front
May 24, 2025
-
Act 2 Scene 1 The Tempest Summary
May 24, 2025
-
Summary Of Julius Caesar Act 3 Scene 2
May 24, 2025
Related Post
Thank you for visiting our website which covers about Time Series Data May Exhibit Which Of The Following Behaviors . We hope the information provided has been useful to you. Feel free to contact us if you have any questions or need further assistance. See you next time and don't miss to bookmark.