The Standard Deviation Can Be Negative.
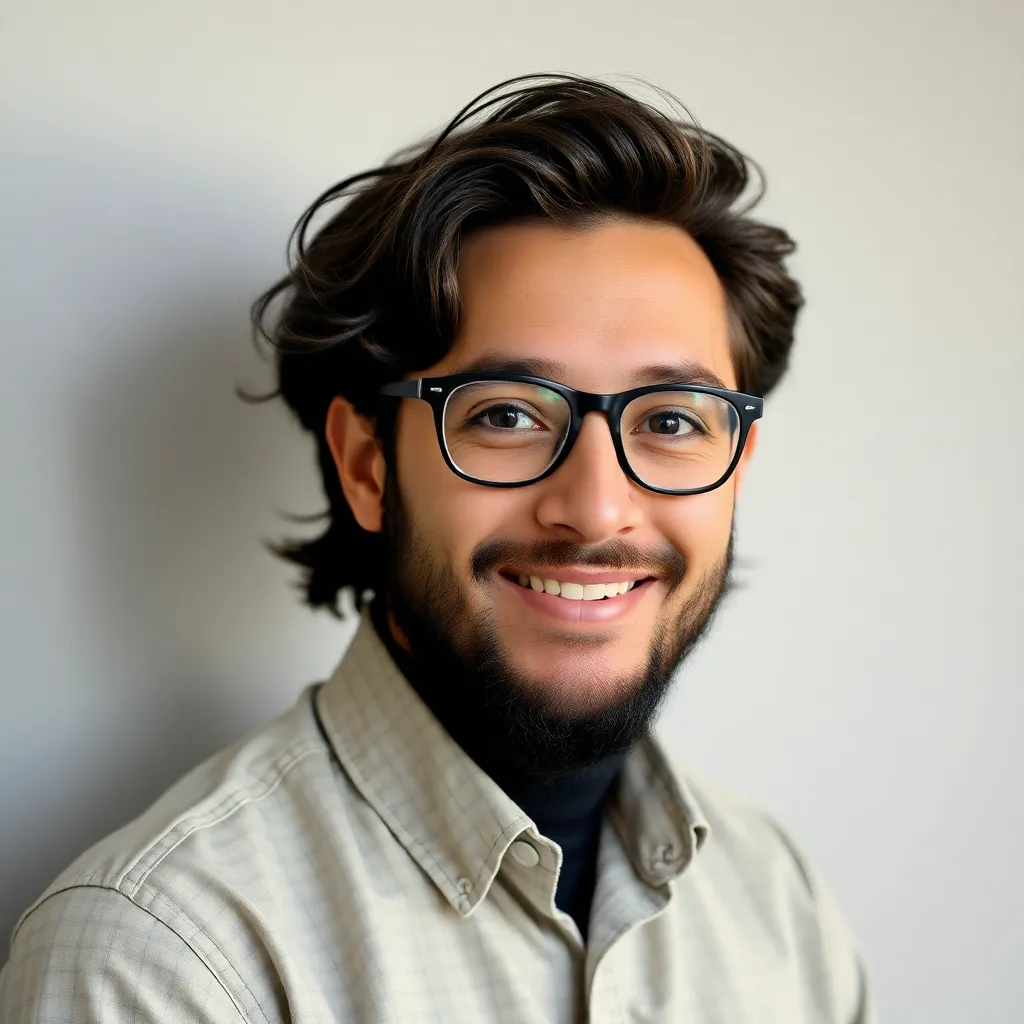
Juapaving
May 10, 2025 · 5 min read
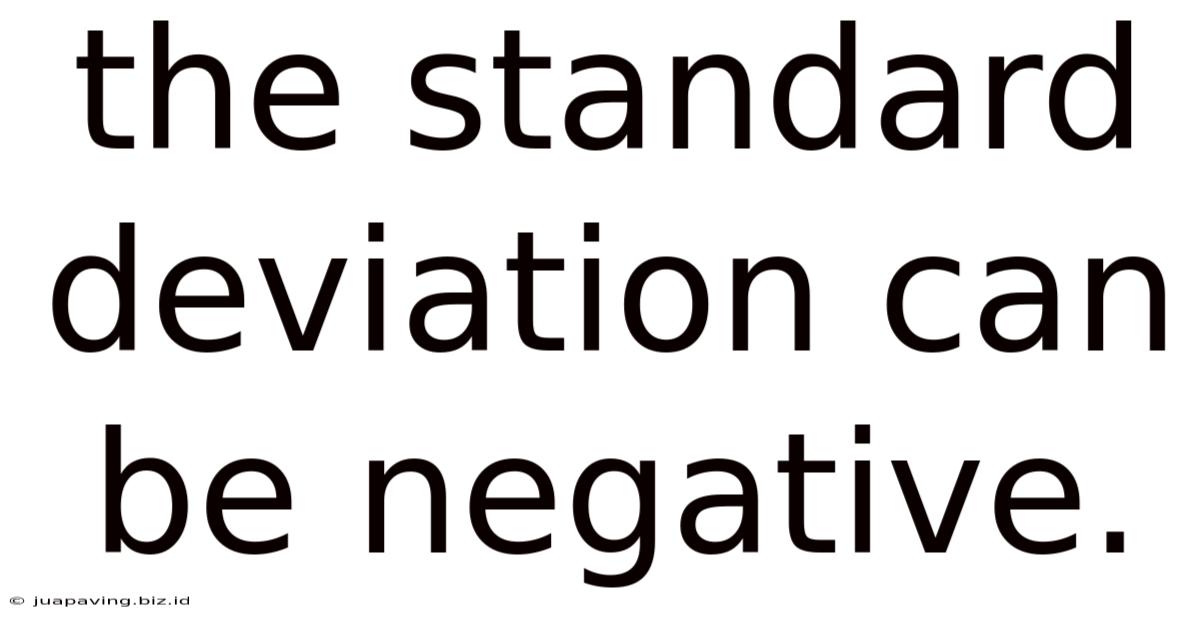
Table of Contents
Can Standard Deviation Be Negative? Understanding the Fundamentals of Statistical Dispersion
The standard deviation, a cornerstone of statistical analysis, quantifies the amount of variation or dispersion within a dataset. It measures how spread out the data points are from the mean (average). A common misconception is that standard deviation can be negative. This article will definitively address this misconception, delve into the mathematical underpinnings of standard deviation, and explore its crucial role in various fields. We'll also discuss related concepts and potential pitfalls in interpretation.
Understanding Standard Deviation: A Mathematical Deep Dive
Standard deviation is calculated by first finding the variance. Variance is the average of the squared differences from the mean. Why square the differences? Because this eliminates the issue of negative differences canceling out positive ones, providing a true measure of dispersion regardless of the direction of deviation from the average.
Here's a step-by-step breakdown of the calculation:
-
Calculate the mean (average) of the dataset. This is a straightforward process: sum all the values and divide by the number of values.
-
Find the difference between each data point and the mean. This reveals how much each data point deviates from the central tendency.
-
Square each of these differences. Squaring ensures all values are positive, preventing cancellation effects.
-
Calculate the average of these squared differences. This is the variance – a measure of the average squared deviation from the mean.
-
Take the square root of the variance. This gives us the standard deviation, restoring the original units of measurement.
The formula for calculating the sample standard deviation (s) is:
s = √[Σ(xi - x̄)² / (n - 1)]
Where:
- xi represents each individual data point.
- x̄ represents the mean of the dataset.
- n represents the number of data points in the sample.
- Σ represents the sum of all values.
The population standard deviation (σ) uses 'n' instead of 'n-1' in the denominator. The use of 'n-1' for sample standard deviation is known as Bessel's correction and provides a less biased estimate of the population standard deviation.
Why Standard Deviation Cannot Be Negative
The square root of a number is always either positive or zero. Since the variance (the number under the square root in the standard deviation formula) is always non-negative (due to the squaring of the differences), the standard deviation will always be non-negative as well. A negative standard deviation is mathematically impossible. Any calculation resulting in a negative standard deviation indicates an error in the calculation process.
Common Misinterpretations and Errors
Several factors can lead to the misconception that standard deviation could be negative:
-
Incorrect formula application: A simple mistake in applying the formula, particularly in calculating the squared differences or the mean, can yield an incorrect, potentially negative, result. Double-checking each step is crucial.
-
Software errors: Statistical software packages, while generally reliable, can occasionally produce errors. It's advisable to verify results using a different method or software if a negative standard deviation is obtained.
-
Misunderstanding of the concept: A lack of understanding of the underlying mathematical principles of standard deviation can lead to misinterpretations. Remembering that it measures dispersion, and that squaring eliminates the sign of the differences is key.
-
Confusing standard deviation with other statistical measures: Standard deviation shouldn't be confused with other metrics like skewness, which can be negative and indicates asymmetry in the data distribution.
Interpreting Standard Deviation: Practical Applications
The standard deviation is not just a theoretical concept; it plays a vital role in numerous fields:
-
Finance: It's used to quantify the risk associated with investments, measuring the volatility of returns. A higher standard deviation indicates greater risk.
-
Quality control: In manufacturing, it helps determine the consistency of a production process. A lower standard deviation suggests higher quality and less variability in the product.
-
Healthcare: It's employed to measure the variability in patient responses to treatments, helping assess treatment efficacy and identify potential side effects.
-
Environmental science: It helps analyze variations in environmental parameters like temperature, precipitation, or pollution levels, providing insights into climate change and environmental impacts.
-
Social sciences: Standard deviation is used to analyze variability in survey data, providing a deeper understanding of population characteristics and attitudes.
Beyond Standard Deviation: Other Measures of Dispersion
While standard deviation is a widely used measure of dispersion, other metrics exist, each with its own strengths and weaknesses:
-
Variance: As already mentioned, variance is the square of the standard deviation. While less intuitive than standard deviation due to its squared units, it plays a crucial role in statistical analysis and more advanced techniques.
-
Range: This is the simplest measure, representing the difference between the maximum and minimum values in a dataset. It's easily calculated but sensitive to outliers.
-
Interquartile range (IQR): The IQR is the difference between the 75th percentile (third quartile) and the 25th percentile (first quartile). It's less sensitive to outliers than the range.
-
Mean absolute deviation (MAD): MAD calculates the average absolute deviation from the mean. It offers a more robust alternative to standard deviation, particularly when dealing with datasets containing outliers.
Advanced Topics: Standard Deviation and Data Distribution
The standard deviation is closely linked to the shape of the data distribution. In a normal distribution (bell curve), approximately 68% of the data falls within one standard deviation of the mean, 95% within two standard deviations, and 99.7% within three standard deviations. This empirical rule is fundamental for interpreting standard deviation within a normally distributed dataset.
However, it's crucial to remember that the standard deviation's interpretation varies depending on the data distribution. In skewed distributions, the empirical rule doesn't hold as accurately. For non-normal distributions, other techniques might be more appropriate for data analysis and interpretation.
Conclusion: A Firm Grasp on Standard Deviation
The standard deviation is a powerful tool in statistical analysis, offering a quantifiable measure of data dispersion. Understanding its calculation, interpretation, and limitations is crucial for anyone working with data. Remember, a negative standard deviation is impossible; any such result points to an error in the calculation or interpretation. By mastering the principles discussed here, you can effectively utilize standard deviation to gain valuable insights from your data, regardless of the field of application. Always double-check your calculations and consider the context of your data before drawing conclusions. This nuanced understanding will enable you to conduct more rigorous and accurate statistical analyses.
Latest Posts
Latest Posts
-
Which Fuel Is Not A Fossil Fuel
May 10, 2025
-
X 2 On A Number Line
May 10, 2025
-
Is Ke Conserved In An Elastic Collision
May 10, 2025
-
Creature Able To Hold Breath Underwater
May 10, 2025
-
Is 14 A Prime Number Or A Composite Number
May 10, 2025
Related Post
Thank you for visiting our website which covers about The Standard Deviation Can Be Negative. . We hope the information provided has been useful to you. Feel free to contact us if you have any questions or need further assistance. See you next time and don't miss to bookmark.