The Median Is The Same As The Quartile
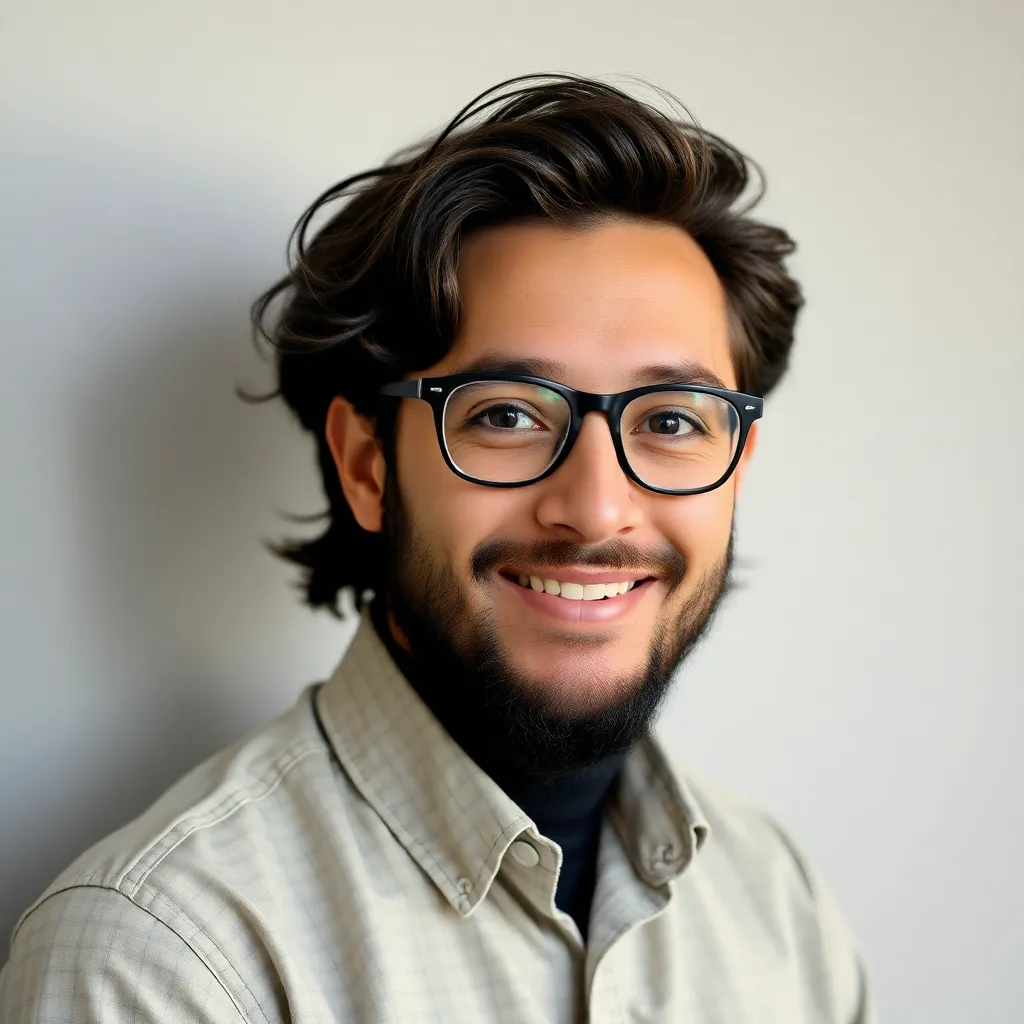
Juapaving
May 12, 2025 · 5 min read
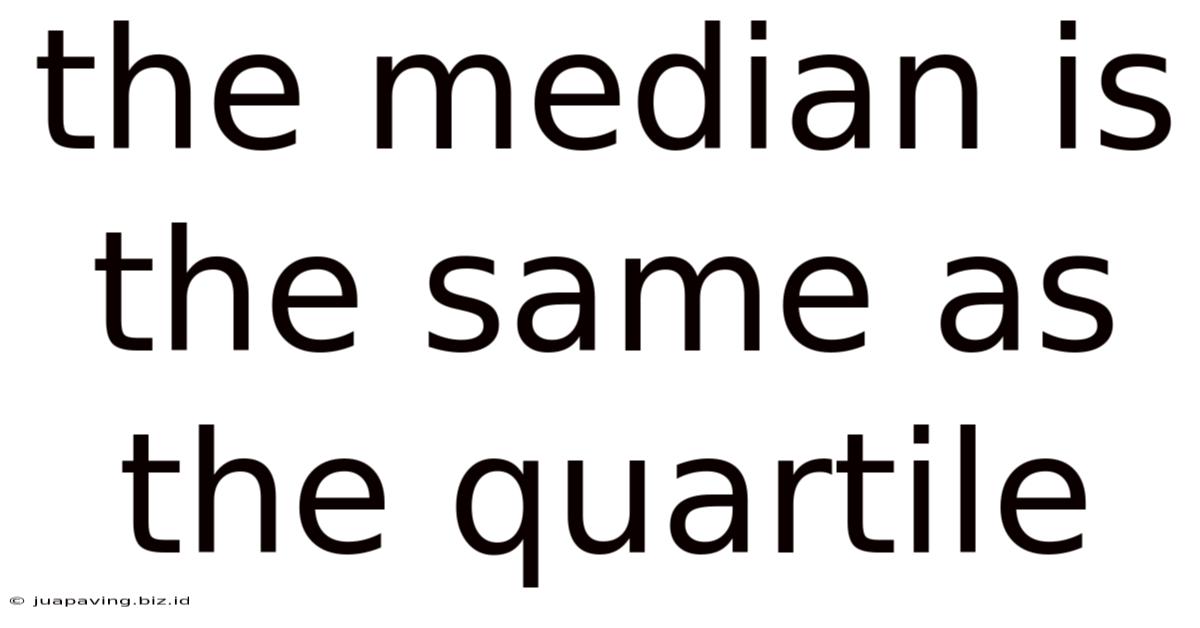
Table of Contents
The Median: A Deep Dive into Quartiles and Their Relationship
The median, a fundamental concept in statistics, often gets intertwined with the discussion of quartiles. While they are closely related, understanding their precise definitions and the nuanced differences between them is crucial for accurate data interpretation and effective data analysis. This in-depth article will explore the median, quartiles, and their relationship, clarifying common misconceptions and providing a comprehensive understanding of their application in various statistical contexts. We will also delve into the scenarios where the median and a quartile might coincide, explaining the conditions that lead to this specific outcome.
Understanding the Median
The median is the middle value in a dataset when the data is ordered from least to greatest. It's a measure of central tendency, providing a representative value of the data's center. For datasets with an odd number of data points, the median is simply the middle value. For datasets with an even number of data points, the median is the average of the two middle values.
Example:
Consider the dataset: {2, 5, 7, 10, 12}. The median is 7.
Now consider: {2, 5, 7, 10}. The median is (5+7)/2 = 6.
The median is less susceptible to the influence of outliers than the mean (average), making it a robust measure of central tendency, particularly when dealing with skewed distributions. It provides a more reliable representation of the "typical" value in the presence of extreme values.
Understanding Quartiles
Quartiles divide a dataset into four equal parts. These divisions are marked by three quartile values:
- Q1 (First Quartile): The value that separates the bottom 25% of the data from the top 75%. It's also known as the lower quartile.
- Q2 (Second Quartile): The value that separates the bottom 50% of the data from the top 50%. This is identical to the median.
- Q3 (Third Quartile): The value that separates the bottom 75% of the data from the top 25%. It's also known as the upper quartile.
The Relationship Between the Median and Quartiles
The key relationship lies in the fact that Q2 (the second quartile) is always equal to the median. This is because the median, by definition, divides the ordered data into two equal halves. The second quartile performs exactly the same function – dividing the data into two equal halves. Therefore, they are mathematically equivalent.
When the Median Might Seem Different From a Quartile (But Isn't)
While the median and Q2 are always identical, misunderstandings can arise due to how data is handled, particularly with small datasets or the presence of duplicate values. Let's examine potential scenarios:
Scenario 1: Even Number of Data Points:
When dealing with an even number of data points, the median is the average of the two middle values. This average might not be an actual value present in the dataset. However, Q2, calculated using the same ordered data, will still yield the same average value. They remain equivalent despite the median not being a direct data point.
Example:
Dataset: {1, 3, 5, 7} Median: (3+5)/2 = 4 Q2: (3+5)/2 = 4
Both the median and Q2 are 4, even though 4 isn't present in the original dataset.
Scenario 2: Duplicate Values:
The presence of multiple instances of the same value in a dataset can sometimes lead to confusion. However, the median and Q2 remain consistent. The calculations still follow the principle of splitting the data into equal halves.
Example:
Dataset: {2, 2, 4, 6, 6, 6} Median: (4+6)/2 = 5 Q2: (4+6)/2 = 5
Again, the median and Q2 are identical, reinforcing their inherent equivalence.
Calculating Quartiles: Methods and Considerations
There are different methods for calculating quartiles, particularly when dealing with datasets containing an even number of data points. The most common methods include:
-
Method 1 (Linear Interpolation): This method uses a formula to calculate the position of each quartile and interpolates between data points when necessary. This method is often preferred for its accuracy and precision.
-
Method 2 (Nearest Rank): This simpler method selects the nearest data point to the calculated quartile position. While easier to compute manually, it can sometimes result in less precise estimations, especially with smaller datasets.
Regardless of the calculation method employed, the second quartile (Q2) will always match the median. The choice of method primarily affects the calculation of Q1 and Q3. The consistency of Q2 with the median remains unaffected by the method chosen.
The Importance of Understanding the Median and Quartiles
The median and quartiles are valuable tools in descriptive statistics. They provide insights into the data's distribution, central tendency, and dispersion. Specifically:
- Median: Provides a robust measure of central tendency, less sensitive to outliers.
- Quartiles: Help determine the spread of the data, identifying potential skewness and the presence of outliers. The interquartile range (IQR = Q3 - Q1) is a key measure of dispersion robust to outliers.
- Box Plots: Quartiles are the foundation for creating box plots, a visual representation of data distribution showing the median, quartiles, and potential outliers.
Understanding the relationship between the median and quartiles empowers you to effectively summarize and interpret data, facilitating better decision-making based on accurate statistical analysis.
Advanced Applications and Statistical Concepts
Beyond basic descriptive statistics, the median and quartiles play crucial roles in more advanced statistical concepts:
- Robust Regression: Methods like median regression use the median to minimize the impact of outliers when fitting regression models.
- Non-parametric Statistics: Quartiles are used in various non-parametric statistical tests, which don't rely on assumptions about the data's distribution.
- Outlier Detection: The interquartile range (IQR) is used to identify potential outliers in a dataset. Values significantly beyond Q1 - 1.5IQR or Q3 + 1.5IQR are often considered outliers.
Conclusion: The Inseparable Link Between Median and Q2
In conclusion, while the terms "median" and "quartile" might seem distinct, their relationship is fundamental and unambiguous: the median is always identical to the second quartile (Q2). Understanding this crucial link is essential for accurate data interpretation and application of various statistical methods. By mastering the concepts of median and quartiles, you enhance your ability to effectively analyze, understand, and communicate data insights across a wide range of applications. The consistency of the median and Q2 underscores their importance as robust and reliable measures of central tendency and data distribution. Remember that choosing the appropriate method for calculating quartiles might slightly alter Q1 and Q3, but the equivalence between the median and Q2 remains steadfast.
Latest Posts
Latest Posts
-
What Is The Freezing Point Of Blood
May 12, 2025
-
In Celsius What Is The Freezing Point Of Water
May 12, 2025
-
Difference Between Internal And External Fertilisation
May 12, 2025
-
What Is The Length Of A Pencil In Cm
May 12, 2025
-
The Largest Lymphatic Organ In The Body
May 12, 2025
Related Post
Thank you for visiting our website which covers about The Median Is The Same As The Quartile . We hope the information provided has been useful to you. Feel free to contact us if you have any questions or need further assistance. See you next time and don't miss to bookmark.