The Frequency Distribution Shown Is Constructed Incorrectly
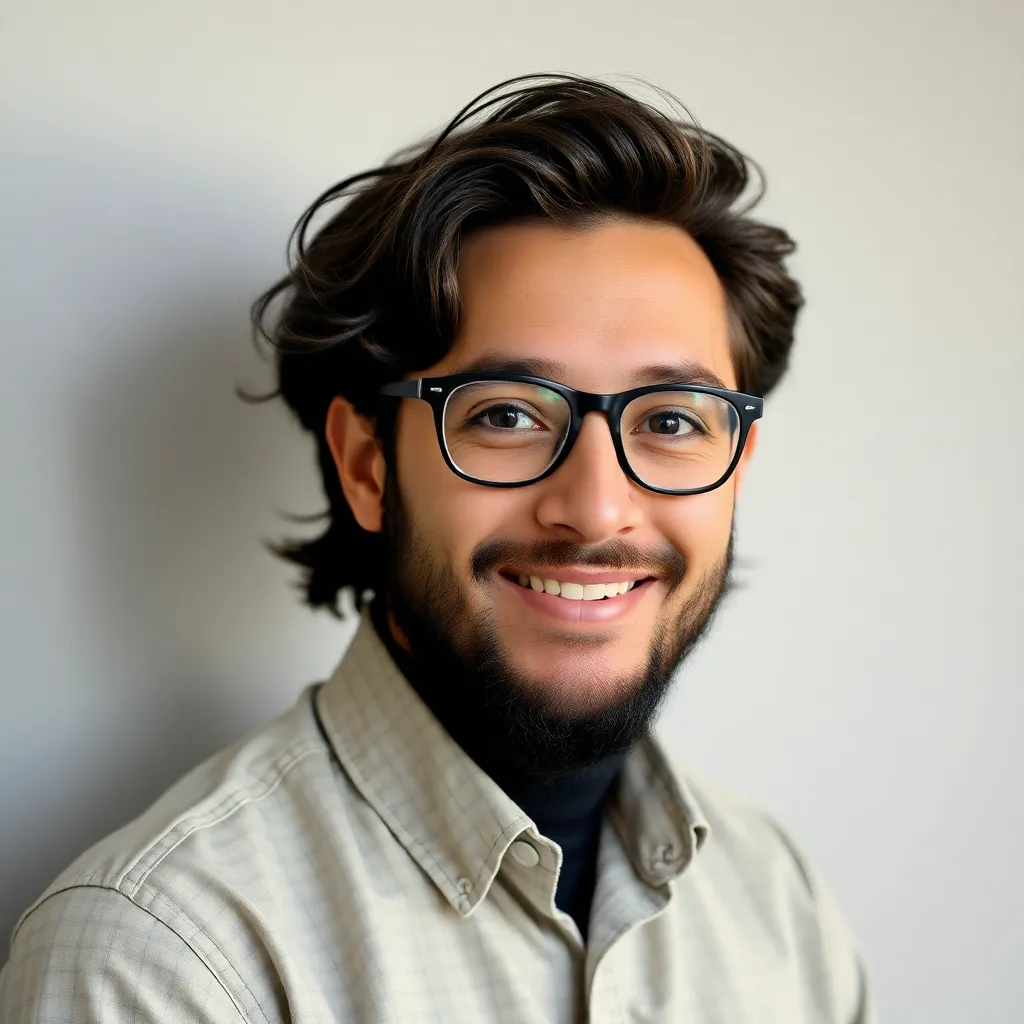
Juapaving
May 25, 2025 · 6 min read
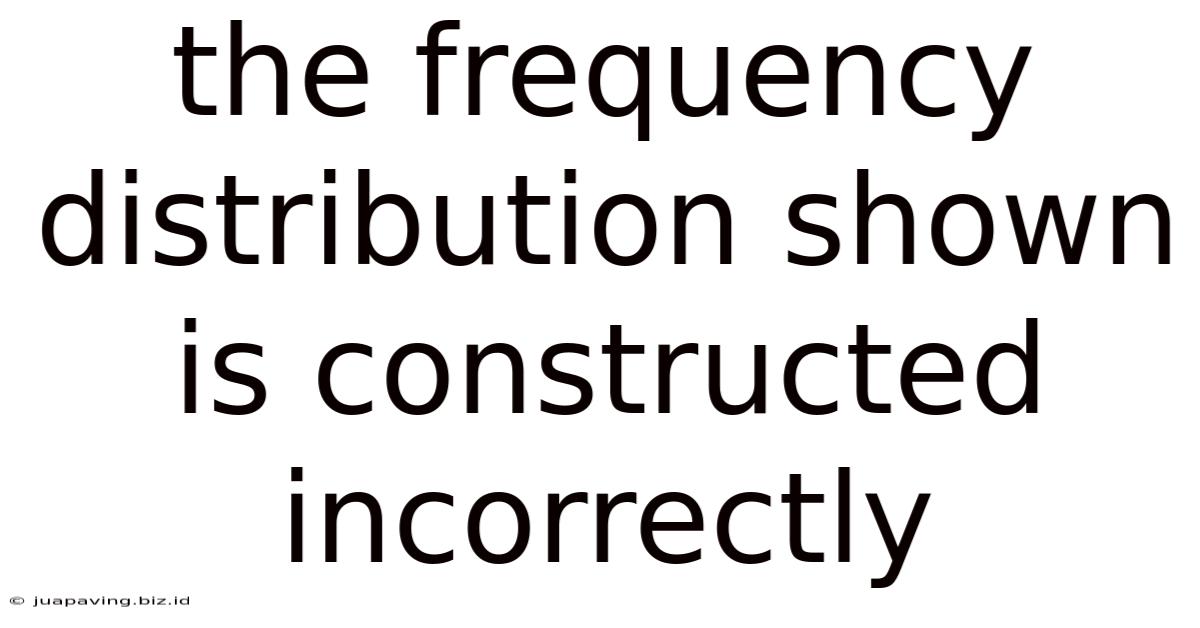
Table of Contents
The Frequency Distribution Shown is Constructed Incorrectly: A Comprehensive Guide to Identifying and Correcting Errors
Frequency distributions are fundamental tools in statistics, providing a concise summary of data by grouping values into classes and showing their frequencies. However, constructing a correct and informative frequency distribution requires careful attention to detail. A poorly constructed frequency distribution can lead to misleading interpretations and inaccurate conclusions. This article delves into the common errors encountered when constructing frequency distributions, providing practical guidance on identifying and correcting them. We will explore various aspects, from choosing appropriate class intervals to handling outliers and ensuring accurate frequency counts.
Common Errors in Frequency Distribution Construction
Several pitfalls can lead to an incorrectly constructed frequency distribution. Let's examine the most frequent mistakes:
1. Overlapping or Unequal Class Intervals: A cardinal rule is that class intervals must be mutually exclusive and of equal width. Overlapping intervals lead to ambiguous data allocation, while unequal intervals distort the visual representation and make comparisons difficult. For example, intervals such as 10-20, 20-30 are incorrect because the value 20 is counted twice. Correct intervals would be 10-19, 20-29, etc. Unequal intervals, while sometimes necessary (e.g., when dealing with skewed data), require careful consideration and should be clearly justified.
2. Incorrect Frequency Counts: Simple counting errors are surprisingly common. Careless counting or misinterpretation of data points can significantly skew the frequency distribution. Independent verification of frequency counts is crucial, particularly when dealing with large datasets. Using spreadsheet software or statistical packages with built-in frequency counting functions can minimize this type of error.
3. Missing or Inaccurate Class Boundaries: Clearly defined class boundaries are essential. Vague or imprecise boundaries lead to confusion regarding data allocation. For example, if the interval is "20-30," it should be specified whether it includes 20 and 30 or excludes them. Using precise boundaries (e.g., 19.5-29.5) eliminates ambiguity.
4. Poor Choice of Number of Classes: The number of classes significantly impacts the visual clarity and interpretability of the frequency distribution. Too few classes obscure important details, while too many classes create a cluttered and uninformative presentation. While there are various rules of thumb (e.g., Sturges' rule, Scott's rule), the optimal number of classes often depends on the specific dataset and the intended analysis.
5. Improper Handling of Outliers: Extreme values, or outliers, can significantly influence the appearance and interpretation of a frequency distribution. Ignoring outliers can lead to a biased representation of the data. However, simply removing outliers without justification is also inappropriate. A better approach is to consider the potential causes of outliers, and if they are genuine data points, they should be included and their impact acknowledged. One might choose to present the distribution with and without the outliers for a comprehensive analysis.
6. Inconsistent Data Representation: The way data is represented within the frequency distribution needs to be consistent. For example, if decimal places are used for one class, the same level of precision should be maintained throughout. Inconsistent data representation creates confusion and undermines the credibility of the distribution.
Identifying Errors in a Given Frequency Distribution
Let's consider a hypothetical example of an incorrectly constructed frequency distribution:
Class Interval | Frequency |
---|---|
10-20 | 5 |
20-30 | 12 |
30-40 | 8 |
40-50 | 3 |
50-60 | 2 |
This frequency distribution might seem reasonable at first glance, but it suffers from a critical error: overlapping class intervals. The value 20 is included in both the 10-20 and 20-30 intervals. This ambiguity makes the data allocation unclear and the frequency counts unreliable.
To correct this error, we need to redefine the class intervals to be mutually exclusive. A corrected version could be:
Class Interval | Frequency |
---|---|
10-19 | 5 |
20-29 | 12 |
30-39 | 8 |
40-49 | 3 |
50-59 | 2 |
This corrected version addresses the overlapping interval issue, leading to a more accurate and reliable frequency distribution.
Techniques for Constructing Accurate Frequency Distributions
The following steps ensure the creation of a correct and informative frequency distribution:
-
Determine the Range: Find the difference between the maximum and minimum values in your dataset.
-
Choose the Number of Classes: Use a rule of thumb or judgment based on data distribution and intended analysis.
-
Calculate the Class Width: Divide the range by the number of classes. Round up to a convenient value for ease of interpretation.
-
Define Class Boundaries: Create mutually exclusive class intervals with clear and precise boundaries.
-
Tally the Frequencies: Carefully count the number of data points that fall into each class interval.
-
Verify Frequency Counts: Independently check the frequency counts to minimize counting errors.
-
Present the Distribution: Clearly present the frequency distribution in a table or histogram. Include all relevant information, such as class intervals, frequencies, and any relevant notes on data handling.
-
Consider Data Visualization: Beyond a simple table, visualize the data with a histogram or frequency polygon for a better understanding of the data's distribution.
Advanced Considerations: Handling Skewed Data and Outliers
When dealing with skewed data or outliers, constructing a frequency distribution requires careful attention.
-
Skewed Data: If the data is highly skewed, unequal class intervals might be necessary to capture the important features of the distribution without losing too much detail. However, clearly indicate the unequal intervals in the presentation.
-
Outliers: If outliers are genuine data points, it is essential to include them in the frequency distribution. However, the presentation should acknowledge their presence and potential influence. Consider displaying the distribution both with and without the outliers for a complete picture. Investigate the cause of the outlier, as it could highlight a problem with data collection or processing.
Conclusion: Ensuring Accuracy and Reliability
Constructing a correct frequency distribution is crucial for accurate data analysis and informed decision-making. By carefully attending to details, avoiding common errors, and employing appropriate techniques, you can create reliable and insightful frequency distributions that accurately represent your data. Remember, a poorly constructed frequency distribution can lead to misleading conclusions, highlighting the importance of meticulous attention to detail throughout the process. Always review and verify your work to ensure accuracy and reliability. Utilizing statistical software can significantly aid in this process, reducing the likelihood of manual errors. Finally, remember that clear communication of your methodology and any limitations of your distribution is paramount for transparency and responsible data analysis.
Latest Posts
Latest Posts
-
2 3 Exponential Functions Practice Answer Key
May 25, 2025
-
A Tree Grows In Brooklyn Characters
May 25, 2025
-
Representation Of Disney Princesses In The Media
May 25, 2025
-
Taking A Holistic Approach To Toughness Includes Using
May 25, 2025
-
Physioex 9 0 Exercise 9 Activity 6
May 25, 2025
Related Post
Thank you for visiting our website which covers about The Frequency Distribution Shown Is Constructed Incorrectly . We hope the information provided has been useful to you. Feel free to contact us if you have any questions or need further assistance. See you next time and don't miss to bookmark.