Putting Forecast Errors Into Perspective Is Best Done Using
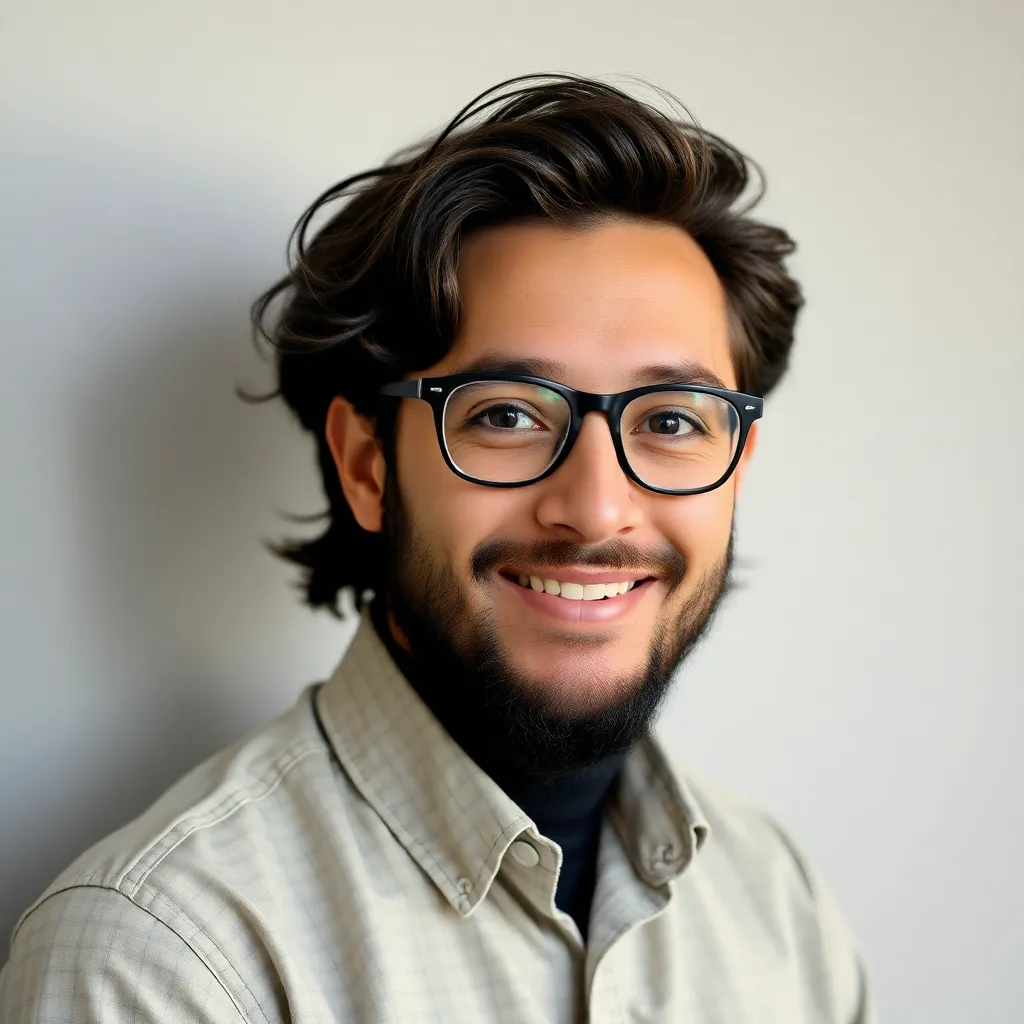
Juapaving
May 25, 2025 · 6 min read
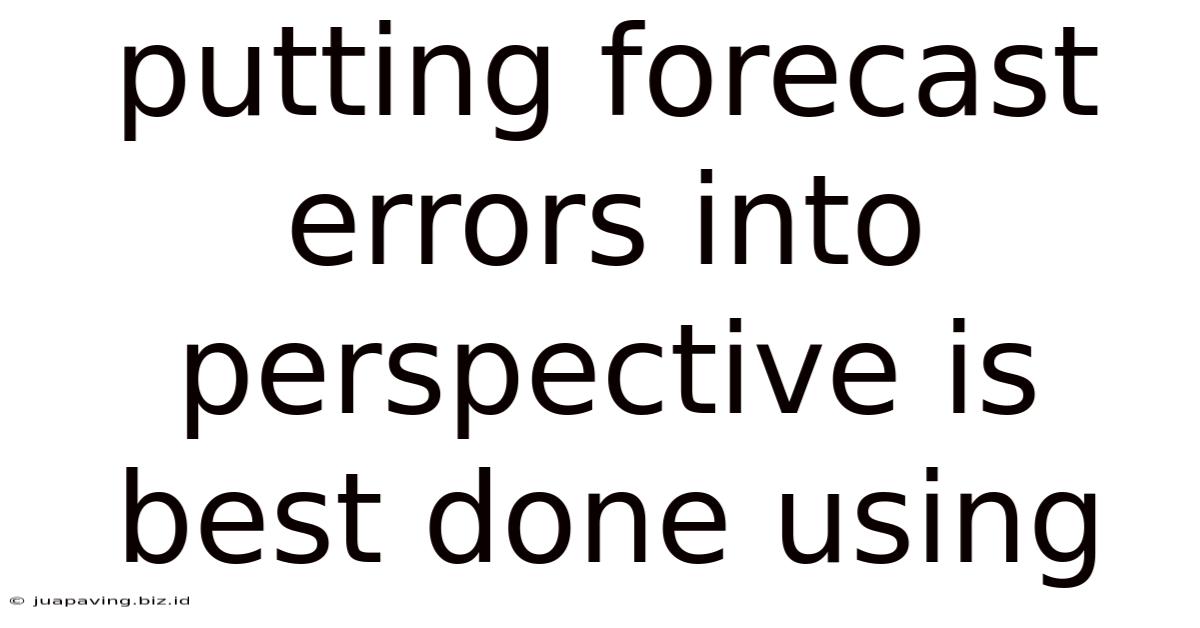
Table of Contents
Putting Forecast Errors into Perspective: The Power of Distributional Methods
Forecasting is an essential tool across numerous fields, from predicting stock prices to estimating weather patterns. However, no forecasting method is perfect. Errors are inevitable. The crucial element isn't avoiding errors entirely – that's impossible – but understanding and effectively managing them. This article delves into the best approach to putting forecast errors into perspective: using distributional forecasting methods. Instead of focusing solely on point forecasts (a single predicted value), we'll explore the advantages of understanding the entire probability distribution of potential future outcomes.
Why Point Forecasts Fall Short
Traditional forecasting methods often focus on providing a single point estimate – a best guess for the future value. While seemingly straightforward, this approach has significant limitations:
-
Ignoring Uncertainty: A single point forecast fails to quantify the uncertainty inherent in future events. The true value could be significantly different from the prediction, and a point forecast offers no indication of this variability.
-
Limited Decision-Making Support: A single number offers limited insight for decision-making. Knowing only the predicted value doesn't help assess the risks associated with different choices or determine appropriate contingency plans.
-
Difficulty in Evaluating Performance: Assessing forecast accuracy based solely on point estimates can be misleading. Two forecasts with the same average error might have drastically different levels of uncertainty.
The Power of Distributional Forecasting
Distributional forecasting provides a more comprehensive picture by producing the entire probability distribution of possible future values. This means instead of a single number, you get a range of possible outcomes, each with an associated probability. This allows for:
-
Quantifying Uncertainty: The distribution visually represents the uncertainty inherent in the forecast. Wider distributions indicate higher uncertainty, while narrower distributions suggest greater confidence.
-
Informed Decision-Making: By understanding the full range of potential outcomes and their probabilities, decision-makers can make more informed choices, considering the risks and rewards associated with different options.
-
Robust Performance Evaluation: Evaluating forecast performance becomes more nuanced. Metrics can go beyond simple average errors to include measures of dispersion and quantile accuracy, providing a more holistic assessment.
Key Distributional Methods
Several methods enable the creation of distributional forecasts. Here are some prominent examples:
1. Quantile Regression
Quantile regression focuses on estimating specific quantiles of the predictive distribution, such as the median, 25th percentile, and 75th percentile. This provides a range of likely outcomes, offering a clearer picture of uncertainty than a single point forecast. It's particularly useful when dealing with skewed data or when the focus is on specific parts of the distribution (e.g., the tail risks).
2. Bayesian Methods
Bayesian methods incorporate prior knowledge and beliefs into the forecasting process. They provide a posterior distribution, reflecting the updated belief about the future outcome after incorporating new data. This approach handles uncertainty elegantly and allows for the quantification of uncertainty at each step of the process.
3. Ensemble Methods
Ensemble methods combine multiple forecasting models to create a more robust and accurate prediction. The distribution of predictions from the ensemble provides a natural estimate of the forecast uncertainty. Methods like bagging and boosting can be adapted to generate distributional forecasts.
4. Simulation-Based Approaches
Simulation methods, like Monte Carlo simulations, can generate numerous possible future scenarios based on the underlying assumptions and model parameters. The resulting distribution of simulated outcomes provides a valuable representation of forecast uncertainty. This is particularly powerful when dealing with complex systems and interacting variables.
Interpreting Distributional Forecasts
Understanding how to interpret a distributional forecast is crucial for effective use. The key elements are:
-
The Mean/Median: This represents the central tendency of the predicted distribution – the most likely outcome.
-
The Variance/Standard Deviation: These metrics quantify the spread or dispersion of the distribution, indicating the uncertainty in the forecast. A larger variance suggests greater uncertainty.
-
Credible Intervals/Prediction Intervals: These intervals provide a range of outcomes within which the true value is likely to fall with a specified probability (e.g., a 95% credible interval suggests there is a 95% probability that the true value lies within that range).
Practical Applications and Examples
The benefits of distributional forecasting extend to various domains:
-
Finance: Predicting stock prices with distributional forecasts allows investors to manage risk more effectively, tailoring their portfolios to different risk tolerances. Understanding the potential downside risk is crucial for informed investment decisions.
-
Weather Forecasting: Weather forecasts expressed as probability distributions are much more informative than single-point predictions. Knowing the probability of different levels of rainfall allows for better planning and resource allocation.
-
Supply Chain Management: Forecasting demand with distributional methods helps companies better manage inventory levels, minimizing stockouts and overstocking. Understanding the variability in demand ensures optimal resource allocation.
-
Public Health: Predicting the spread of infectious diseases using distributional forecasting assists in planning for healthcare resource allocation and public health interventions. Quantifying the uncertainty in the prediction allows for more robust planning and contingency measures.
-
Energy Management: Accurate distributional forecasts of energy consumption help in optimizing energy production and distribution, leading to increased efficiency and reduced costs.
For instance, instead of predicting that tomorrow's temperature will be 20°C, a distributional forecast might state: "There is a 60% probability that tomorrow's temperature will be between 18°C and 22°C, a 90% probability it will be between 15°C and 25°C, and a 5% probability it will be below 15°C or above 25°C." This nuanced forecast offers a much more comprehensive picture for decision-making.
Beyond the Numbers: Communicating Uncertainty Effectively
Presenting distributional forecasts effectively is as important as generating them. Avoid overwhelming the audience with complex statistical jargon. Instead, use clear visuals:
-
Histograms or Density Plots: These provide a visual representation of the probability distribution, showing the range of possible outcomes and their associated probabilities.
-
Box Plots: Useful for summarizing the key features of the distribution (median, quartiles, outliers).
-
Interactive Dashboards: Allow users to explore the distribution dynamically, changing parameters and observing the impact on the forecast.
When communicating forecast uncertainty, always emphasize the probability associated with different outcomes, and clearly define the level of confidence associated with the prediction intervals.
Conclusion: Embracing Uncertainty for Better Decisions
In conclusion, shifting from point forecasts to distributional forecasting offers a significant advancement in forecasting practice. While point forecasts offer simplicity, they sacrifice valuable information about uncertainty. By embracing the uncertainty inherent in forecasting and utilizing distributional methods, we gain a clearer picture of possible future outcomes, facilitating more informed decision-making across a wide range of applications. The added complexity is far outweighed by the benefits of more robust, insightful, and ultimately, more useful forecasts. The future of forecasting lies in understanding and effectively communicating uncertainty, enabling better preparedness and strategic decision-making in an inherently uncertain world.
Latest Posts
Latest Posts
-
A Race Car Rounds One End Of A Speedway
May 25, 2025
-
Bread And The Power Of Imagination Answer Key
May 25, 2025
-
The Litmus Test Can Be Used To Help You
May 25, 2025
-
When Performing An American Bikini Wax
May 25, 2025
-
All Quiet On The Western Front Chapter 11
May 25, 2025
Related Post
Thank you for visiting our website which covers about Putting Forecast Errors Into Perspective Is Best Done Using . We hope the information provided has been useful to you. Feel free to contact us if you have any questions or need further assistance. See you next time and don't miss to bookmark.