Measuring The Average Sentiment Expressed About A Company
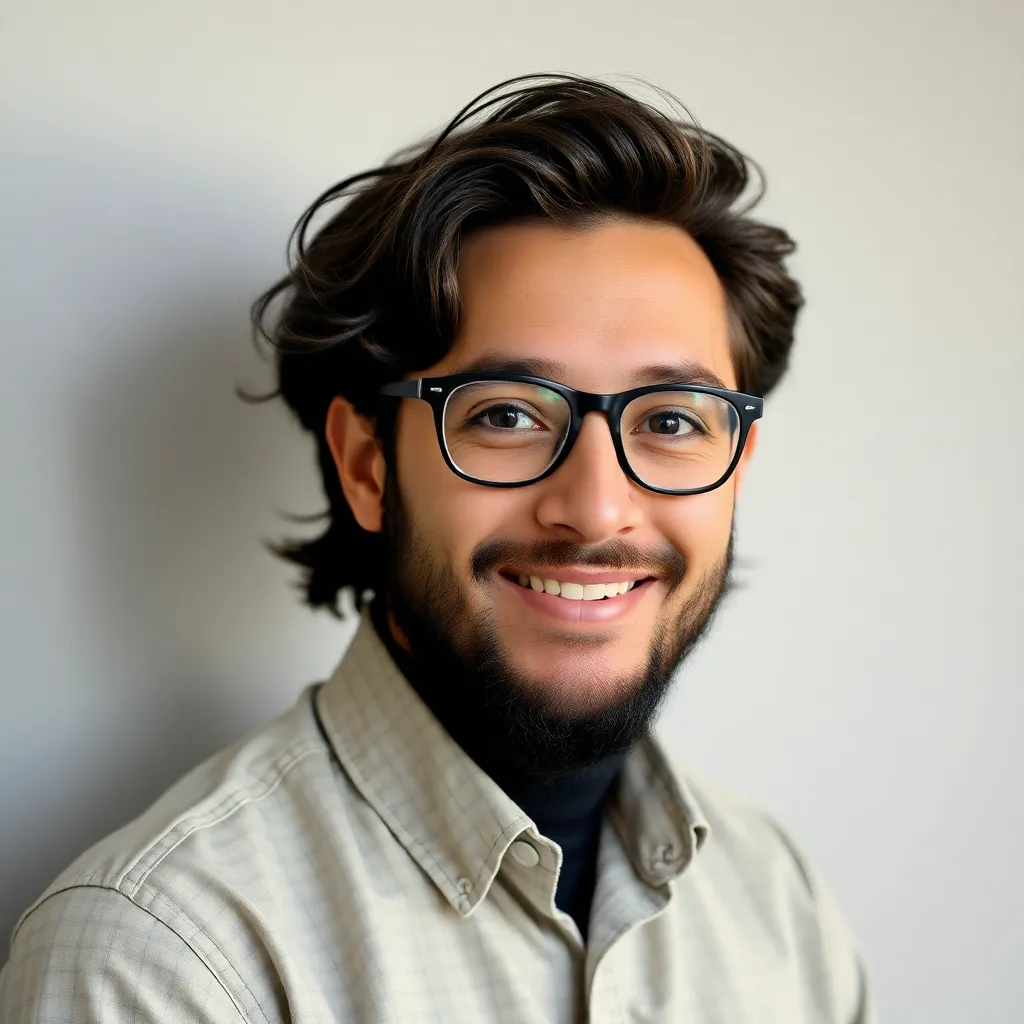
Juapaving
May 24, 2025 · 6 min read
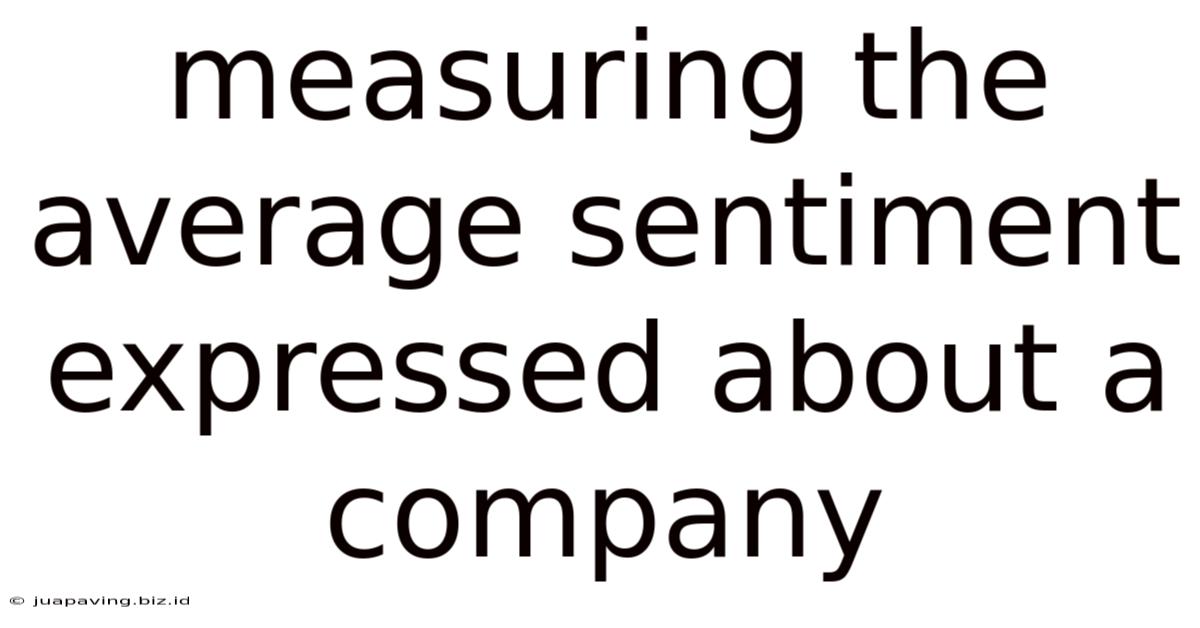
Table of Contents
Measuring the Average Sentiment Expressed About a Company: A Comprehensive Guide
Understanding public sentiment towards your company is crucial for success in today's competitive landscape. Positive sentiment translates to increased brand loyalty, stronger customer relationships, and ultimately, higher profitability. Negative sentiment, on the other hand, can severely damage your reputation, leading to lost sales and even legal issues. This comprehensive guide explores the various methods of measuring the average sentiment expressed about a company, from manual analysis to sophisticated AI-powered tools.
Why Measuring Sentiment is Critical for Businesses
Before diving into the how, let's examine the why. Understanding public sentiment provides invaluable insights into several key areas:
1. Brand Reputation Management:
Negative sentiment can spread like wildfire online. By actively monitoring and analyzing sentiment, you can identify potential PR crises early on and mitigate their impact. This proactive approach is far more effective than reactive damage control. Early detection allows for swift responses, demonstrating accountability and commitment to customer satisfaction.
2. Product Development and Improvement:
Customer feedback, often expressed through sentiment, offers valuable insights into product strengths and weaknesses. Negative sentiment surrounding a specific product feature, for example, might highlight areas requiring improvement or redesign. Positive sentiment, conversely, can indicate successful product features and areas to leverage in future marketing campaigns.
3. Marketing Campaign Effectiveness:
Measuring sentiment before, during, and after a marketing campaign allows for objective evaluation of its success. Is your messaging resonating with your target audience? Are customers responding positively to your new product launch? Sentiment analysis provides data-driven answers to these crucial questions.
4. Competitive Analysis:
Analyzing sentiment towards your competitors can reveal their strengths and weaknesses in the eyes of the consumer. This comparative analysis can inform your own marketing and product development strategies, helping you gain a competitive edge.
5. Customer Service Enhancement:
Negative sentiment expressed on social media or review platforms often indicates areas where customer service needs improvement. By analyzing these expressions, you can identify recurring issues and develop strategies to enhance the customer experience.
Methods for Measuring Average Sentiment
Several methods exist for measuring the average sentiment expressed about a company. These range from simple manual analysis to sophisticated AI-powered tools.
1. Manual Sentiment Analysis:
This traditional approach involves manually reading and classifying comments, reviews, and social media posts as positive, negative, or neutral. While this method provides a deep understanding of the nuances in language, it's extremely time-consuming and impractical for large datasets. It's suitable for smaller-scale projects or as a supplementary method to validate automated analysis.
Advantages:
- High Accuracy (for smaller datasets): Allows for careful consideration of context and nuances.
- In-depth Understanding: Provides qualitative insights beyond simple sentiment scores.
Disadvantages:
- Extremely Time-Consuming: Inefficient for large volumes of data.
- Prone to Subjectivity: Analyst bias can influence the classification.
- Scalability Issues: Difficult to maintain consistency across multiple analysts.
2. Automated Sentiment Analysis using Natural Language Processing (NLP):
NLP is a branch of artificial intelligence that enables computers to understand and process human language. Automated sentiment analysis tools leverage NLP techniques to analyze large volumes of text data and determine the overall sentiment. These tools typically assign a sentiment score (e.g., -1 to +1, where -1 is highly negative and +1 is highly positive) to each piece of text.
Advantages:
- High Speed and Efficiency: Processes large datasets quickly.
- Scalability: Handles massive volumes of data with ease.
- Objectivity: Minimizes human bias in classification.
Disadvantages:
- Dependence on Data Quality: Accuracy relies heavily on the quality and quantity of training data.
- Contextual Challenges: Sarcasm, irony, and other nuanced language can be misinterpreted.
- Cost: Advanced NLP tools can be expensive.
3. Lexicon-Based Approach:
This method uses a pre-defined lexicon or dictionary of words and their associated sentiment scores. The tool counts the occurrences of these words in the text and calculates an overall sentiment score based on their weights. This approach is simpler and less computationally intensive than sophisticated machine learning models.
Advantages:
- Simplicity and Ease of Implementation: Relatively straightforward to set up and use.
- Computational Efficiency: Faster and less resource-intensive than other methods.
Disadvantages:
- Limited Accuracy: May not capture the subtleties of language and context.
- Contextual Dependence: The same word can have different sentiments depending on the context.
4. Machine Learning-Based Approach:
This advanced technique utilizes machine learning algorithms to train models on large datasets of labeled text data. These models learn to identify patterns and relationships between words and their associated sentiments, leading to more accurate and nuanced sentiment analysis. Different algorithms, such as Naive Bayes, Support Vector Machines (SVM), and deep learning models, can be employed.
Advantages:
- High Accuracy: Typically provides more accurate results than lexicon-based approaches.
- Adaptability: Can be trained on specific industry or domain-specific data.
- Contextual Understanding: Better handles contextual nuances and complexities.
Disadvantages:
- Data Dependence: Requires large, high-quality datasets for training.
- Computational Cost: Training and deploying machine learning models can be resource-intensive.
- Expertise Required: Requires specialized knowledge in machine learning and data science.
Data Sources for Sentiment Analysis
The accuracy and comprehensiveness of your sentiment analysis depend heavily on the quality and diversity of your data sources. Consider the following:
- Social Media Platforms: Twitter, Facebook, Instagram, and Reddit offer vast amounts of user-generated content that reflects public sentiment.
- Online Reviews: Sites like Yelp, TripAdvisor, and Amazon provide valuable customer feedback on products and services.
- News Articles and Blogs: Media outlets often report on company news and public opinion, providing valuable contextual information.
- Forums and Online Communities: Dedicated forums and online communities related to your industry can offer insightful discussions and opinions.
- Customer Surveys and Feedback Forms: Directly solicit feedback from your customers through surveys and feedback forms.
Analyzing and Interpreting the Results
Once you've collected and analyzed your data, the next step is to interpret the results. Consider these factors:
- Average Sentiment Score: Calculate the overall average sentiment score across all data points. This provides a general indication of the prevailing sentiment.
- Sentiment Distribution: Analyze the distribution of sentiment scores to understand the range of opinions. Are most people expressing strongly positive or negative views, or is the sentiment more evenly distributed?
- Trend Analysis: Track changes in sentiment over time to identify emerging trends and patterns. This can reveal the impact of specific events or marketing campaigns.
- Topic Modeling: Identify the key topics or themes associated with positive and negative sentiment. This can reveal areas of strength and weakness.
- Qualitative Analysis: Supplement quantitative analysis with qualitative insights from manual review of selected comments and reviews. This helps understand the why behind the sentiment scores.
Best Practices for Effective Sentiment Analysis
To maximize the effectiveness of your sentiment analysis, follow these best practices:
- Define Clear Objectives: Clearly define what you hope to achieve with your sentiment analysis before you begin.
- Choose Appropriate Methods: Select the most appropriate methods based on your data volume, resources, and desired level of accuracy.
- Ensure Data Quality: Clean and pre-process your data to remove noise and irrelevant information.
- Validate Your Results: Compare automated results with manual analysis to ensure accuracy and identify potential biases.
- Regular Monitoring: Regularly monitor sentiment to track changes and respond to emerging trends.
- Contextualize Your Findings: Consider external factors that might influence sentiment.
Conclusion
Measuring the average sentiment expressed about a company is a crucial aspect of modern business intelligence. By utilizing a combination of manual and automated methods, analyzing data from diverse sources, and carefully interpreting the results, businesses can gain valuable insights into public perception, enhance their brand reputation, and make data-driven decisions that lead to success. Remember that effective sentiment analysis is an ongoing process that requires continuous monitoring, adaptation, and refinement. The journey to understanding and improving your brand's sentiment is a dynamic and essential component of long-term success.
Latest Posts
Latest Posts
-
Kitchener Model Of Ethical Decision Making
May 24, 2025
-
Analysis Of I Hear America Singing
May 24, 2025
-
How Are Jalousie Windows Normally Opened And Closed
May 24, 2025
-
Match Each Term To Its Description
May 24, 2025
-
Analysis The Gift Of The Magi
May 24, 2025
Related Post
Thank you for visiting our website which covers about Measuring The Average Sentiment Expressed About A Company . We hope the information provided has been useful to you. Feel free to contact us if you have any questions or need further assistance. See you next time and don't miss to bookmark.