Identify The Model That Represents A Mixture Of Two Compounds
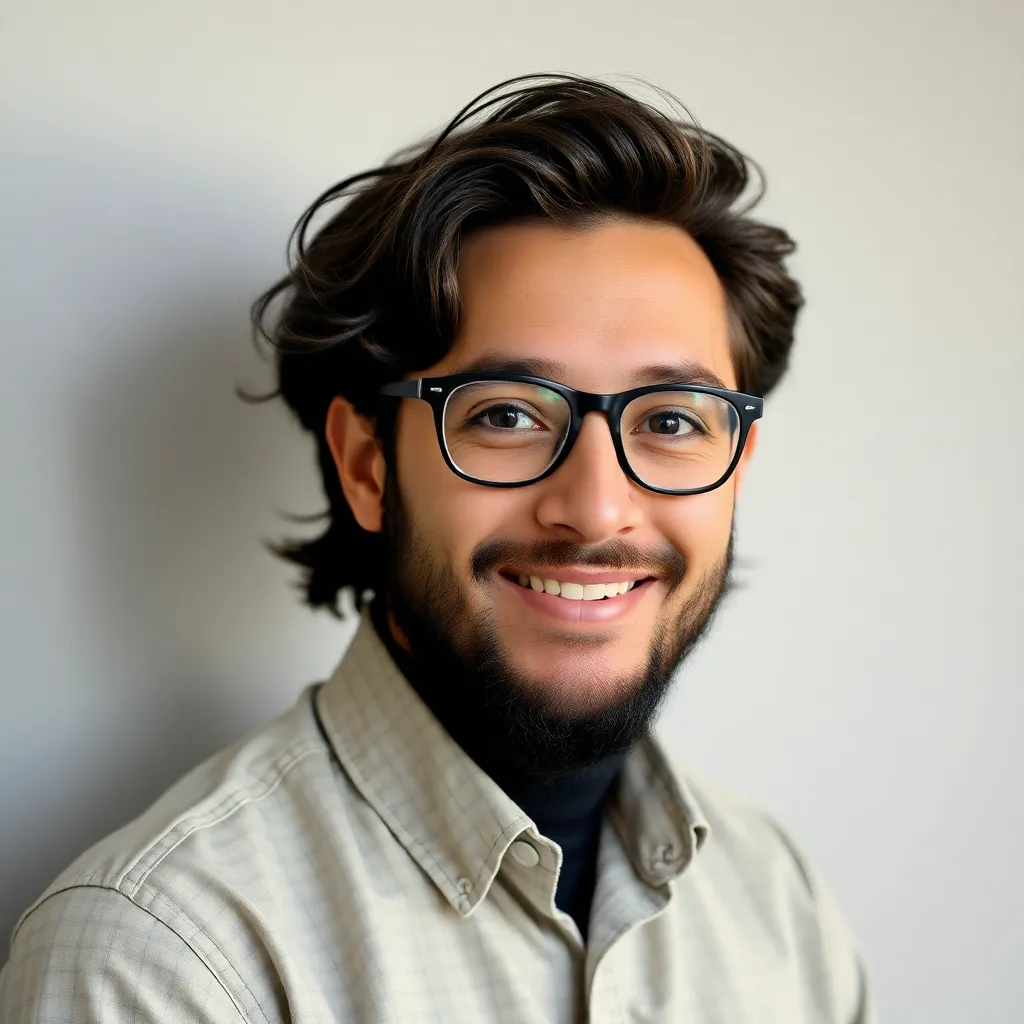
Juapaving
May 09, 2025 · 6 min read
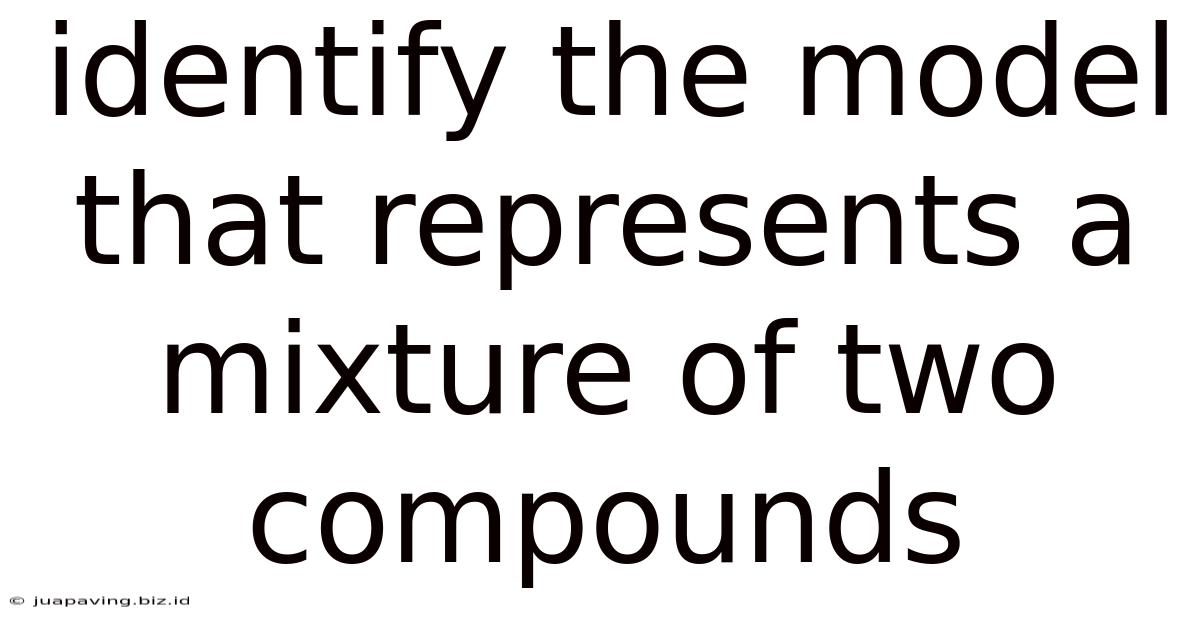
Table of Contents
Identifying the Model Representing a Mixture of Two Compounds
Understanding the composition of mixtures is crucial across various scientific disciplines, from chemistry and materials science to environmental monitoring and pharmaceutical analysis. Often, we encounter mixtures comprising two or more distinct compounds. Accurately identifying the model that best represents such a mixture requires a careful examination of its physical and chemical properties, coupled with appropriate analytical techniques. This article delves into various methods and models used to characterize these mixtures, emphasizing the importance of distinguishing between different types of mixtures and the limitations of each modeling approach.
Types of Mixtures: A Foundation for Model Selection
Before diving into model identification, it's crucial to understand the different types of mixtures. This classification significantly impacts the choice of suitable models. Broadly, mixtures can be categorized as:
1. Homogeneous Mixtures: Solutions
In homogeneous mixtures, also known as solutions, the components are uniformly distributed at a molecular level. The individual components are indistinguishable to the naked eye, and the mixture has a uniform composition throughout. Examples include saltwater, air (a mixture of gases), and many alloys. Modeling homogeneous mixtures often relies on concepts like concentration (e.g., molarity, molality), activity coefficients, and thermodynamic models like the Debye-Hückel theory for electrolyte solutions.
2. Heterogeneous Mixtures: Suspensions and Colloids
Heterogeneous mixtures, conversely, exhibit non-uniform composition. The individual components remain distinct and are visibly separable. This category includes:
- Suspensions: These mixtures contain larger particles that tend to settle out over time (e.g., sand in water).
- Colloids: These mixtures have particles of intermediate size, dispersed throughout the medium, that do not settle out easily (e.g., milk, fog).
Modeling heterogeneous mixtures is more complex and often requires considering factors like particle size distribution, interfacial interactions, and sedimentation behavior. Techniques like image analysis, particle tracking, and sedimentation analysis are often employed.
Analytical Techniques for Mixture Characterization
The selection of an appropriate model for a mixture of two compounds often begins with detailed characterization using analytical techniques. Several methods can provide valuable insights into the mixture's composition:
1. Spectroscopy (UV-Vis, IR, NMR)
Spectroscopic techniques are powerful tools for identifying individual compounds within a mixture. Each compound exhibits a unique spectral fingerprint. By analyzing the spectrum of the mixture and comparing it to known spectra of pure compounds, the constituents can be identified. Furthermore, the relative intensities of the spectral peaks can often be used to quantify the relative amounts of each component.
- UV-Vis Spectroscopy: Useful for identifying compounds with conjugated pi systems.
- IR Spectroscopy: Provides information on functional groups present in the molecules.
- NMR Spectroscopy: Offers detailed structural information about the molecules, including their connectivity and chemical environment.
2. Chromatography (GC, HPLC)
Chromatographic techniques separate the components of a mixture based on their differential interactions with a stationary and a mobile phase. This separation allows for the identification and quantification of individual components.
- Gas Chromatography (GC): Suitable for volatile and thermally stable compounds.
- High-Performance Liquid Chromatography (HPLC): Applicable to a broader range of compounds, including non-volatile and thermally labile ones.
3. Mass Spectrometry (MS)
Mass spectrometry determines the mass-to-charge ratio of ions, providing information on the molecular weight and fragmentation patterns of compounds in a mixture. Coupled with chromatography (e.g., GC-MS, LC-MS), it offers a highly sensitive and specific method for identifying and quantifying components.
4. X-ray Diffraction (XRD)
XRD is particularly useful for characterizing crystalline solid mixtures. The diffraction pattern provides information on the crystal structure and the relative abundance of different crystalline phases.
Models for Representing Mixtures of Two Compounds
The choice of the most appropriate model depends heavily on the nature of the mixture (homogeneous or heterogeneous) and the desired level of detail.
1. Simple Additive Models for Homogeneous Mixtures
For homogeneous mixtures where interactions between components are minimal, a simple additive model might suffice. This model assumes that the properties of the mixture are a linear combination of the properties of its individual components:
Property_mixture = (x_A * Property_A) + (x_B * Property_B)
where:
x_A
andx_B
are the mole fractions or weight fractions of components A and B, respectively.Property_A
andProperty_B
are the properties of pure components A and B.
This model is applicable to properties like density, refractive index, and sometimes even specific heat capacity for ideal mixtures. However, it fails when significant interactions exist between components.
2. Ideal Solution Models
The ideal solution model expands on the simple additive model by incorporating the concept of activity coefficients. It assumes that the interactions between similar molecules (A-A and B-B) are identical to those between dissimilar molecules (A-B). This assumption is rarely perfectly true, but it often provides a reasonable approximation for mixtures of similar molecules. The activity coefficient (γ) accounts for deviations from ideality:
Property_mixture = (x_A * γ_A * Property_A) + (x_B * γ_B * Property_B)
3. Non-Ideal Solution Models
For many real-world mixtures, the ideal solution model is inadequate. Non-ideal solution models account for deviations from ideality caused by significant interactions between the components. These models often employ empirical or semi-empirical equations to describe the activity coefficients, such as:
- Margules equation: A simple two-parameter equation that captures deviations from ideality.
- van Laar equation: Another two-parameter equation that is particularly useful for mixtures with significant deviations from ideality.
- Wilson equation: A more complex model that considers the molecular size and interaction energies of the components.
- NRTL (Non-Random Two-Liquid) equation: A model commonly used for mixtures exhibiting strong non-idealities, particularly those with liquid-liquid phase separation.
- UNIQUAC (Universal Quasi-Chemical) equation: Accounts for both the size and shape of the molecules in the mixture.
The choice among these models often depends on the specific system and the availability of experimental data for parameter estimation.
4. Models for Heterogeneous Mixtures
Modeling heterogeneous mixtures is significantly more challenging. The choice of model often depends on the specific type of heterogeneity. For suspensions, models that account for sedimentation and particle-particle interactions might be necessary. For colloids, models considering interparticle forces (e.g., van der Waals forces, electrostatic interactions) are essential. Techniques like population balance modeling or discrete element method (DEM) simulations can be useful.
Model Validation and Refinement
Once a model is selected, it’s crucial to validate it against experimental data. This often involves comparing predicted properties of the mixture with those measured experimentally. Statistical measures, such as the root-mean-square error (RMSE) or the coefficient of determination (R²), can be used to assess the model's goodness of fit. If the model does not accurately predict the experimental data, it might need refinement or replacement with a more suitable model. This iterative process of model selection, validation, and refinement is crucial for accurate representation of the mixture.
Conclusion: A Holistic Approach to Mixture Modeling
Identifying the model that best represents a mixture of two compounds is a multi-step process requiring careful consideration of the mixture's type, the available analytical data, and the desired accuracy. While simple additive models can suffice for some ideal mixtures, more sophisticated models are necessary for non-ideal systems. The selection of an appropriate model is a crucial step toward understanding the behavior and properties of the mixture and utilizing this information effectively in various applications. Continual refinement and validation against experimental data are vital for ensuring the model's accuracy and reliability. The combination of robust analytical techniques and appropriate modeling approaches is essential for achieving a comprehensive understanding of mixtures and their properties.
Latest Posts
Latest Posts
-
5 Cm In Km Can Be Written As
May 09, 2025
-
In The Diagram Which One Represents A Hypertonic Solution
May 09, 2025
-
How Do You Estimate A Quotient
May 09, 2025
-
How Many Meters In 1 5 Kilometers
May 09, 2025
-
The Vertebral Column Extends From The Skull To The
May 09, 2025
Related Post
Thank you for visiting our website which covers about Identify The Model That Represents A Mixture Of Two Compounds . We hope the information provided has been useful to you. Feel free to contact us if you have any questions or need further assistance. See you next time and don't miss to bookmark.