Identify The Following Examples As Either Small-n Or Large-n Designs.
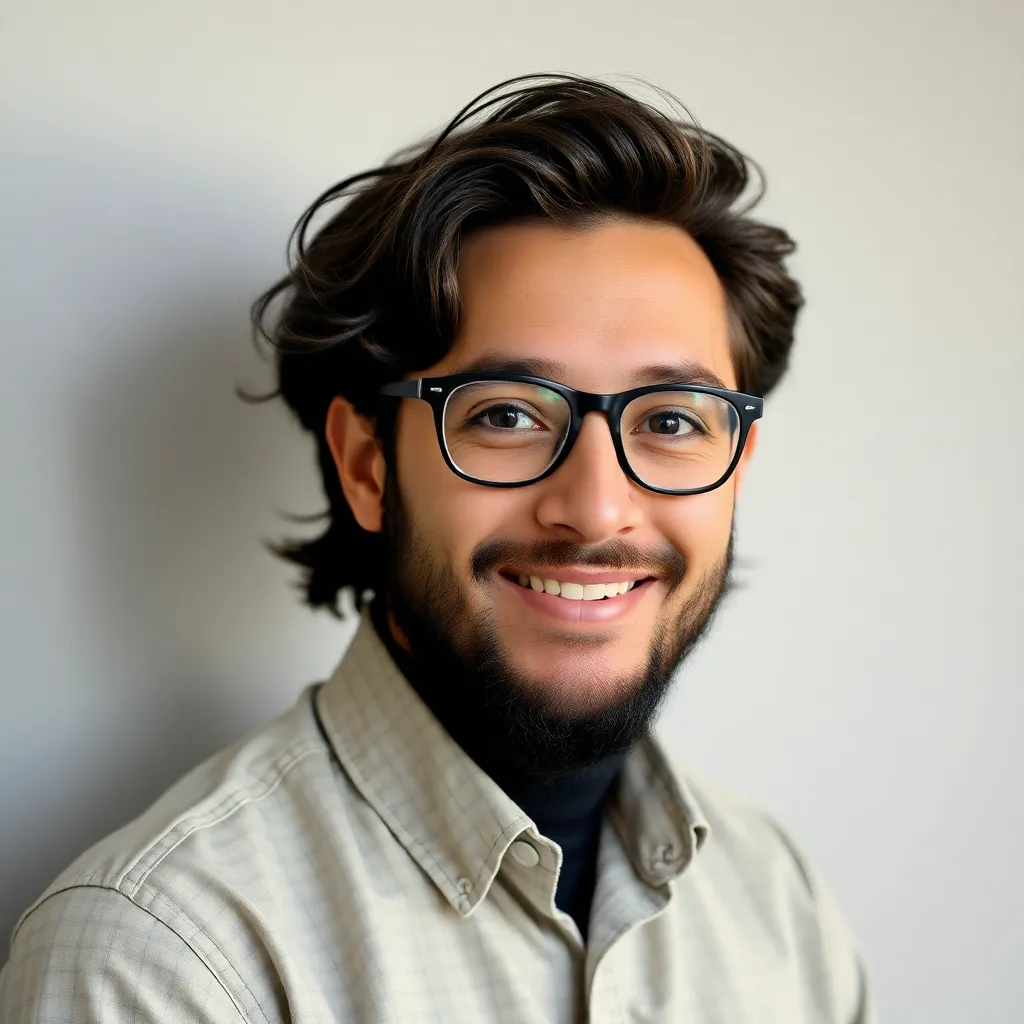
Juapaving
May 25, 2025 · 7 min read
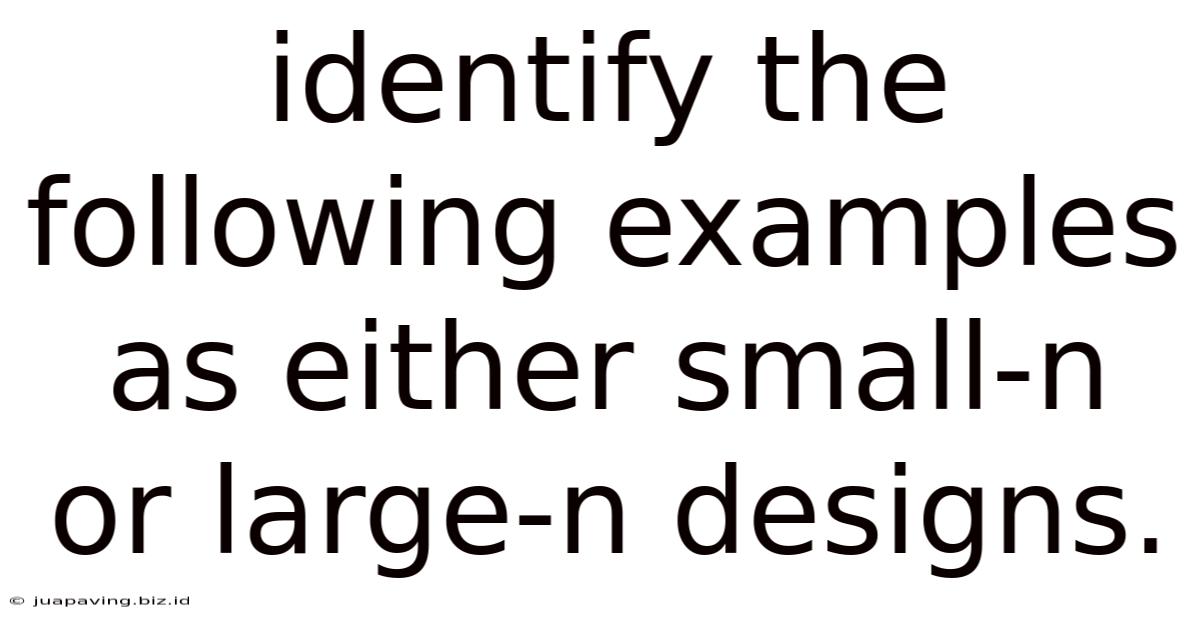
Table of Contents
Identifying Small-N and Large-N Designs in Research
Understanding the distinction between small-n and large-n designs is crucial for researchers across various disciplines. The choice between these approaches significantly impacts the research methods employed, the types of analyses conducted, and the conclusions that can be drawn. This article delves into the characteristics of small-n and large-n designs, providing clear examples to solidify your understanding. We'll explore their strengths and weaknesses, highlighting situations where each design is most appropriate.
What are Small-N and Large-N Designs?
The core difference lies in the number of participants or data points used in the study.
Large-N designs, also known as between-subjects designs, typically involve a large number of participants (often exceeding 30, but this number is context-dependent). The focus is on establishing generalizable findings that apply to a broader population. Data analysis often relies on inferential statistics to test hypotheses about population parameters. The goal is to identify average effects and establish statistically significant relationships between variables. Individual participant data is often less important than the overall pattern across the group.
Small-N designs, also known as within-subjects designs or single-case experimental designs (SCEDs), focus on a limited number of participants (typically one to five). The emphasis is on understanding the behavior of individuals in detail. Rather than seeking to generalize to a broader population, the aim is to demonstrate a clear cause-and-effect relationship within the individual(s) studied. Data analysis focuses on visual inspection of changes in behavior over time and may involve less complex statistical procedures. The individual participant's data is paramount.
Key Differences Summarized:
Feature | Large-N Design | Small-N Design |
---|---|---|
Number of Participants | Many (often 30+) | Few (1-5) |
Focus | Generalizable findings, population effects | Individual behavior, causal relationships |
Data Analysis | Inferential statistics, group comparisons | Visual inspection of trends, simple statistics |
Goal | Identify average effects, test hypotheses | Demonstrate cause-and-effect within individuals |
Generalizability | High (potentially) | Low (typically) |
Internal Validity | Can be high, depending on design | Can be very high with careful experimental control |
External Validity | Can be lower, dependent on sampling | Can be low, generalization limited to individuals |
Examples of Small-N and Large-N Designs:
Let's analyze several research scenarios, classifying them as either small-n or large-n designs and explaining why.
Examples of Large-N Designs:
-
A study investigating the effectiveness of a new antidepressant medication: This study would likely involve hundreds or even thousands of participants, randomly assigned to either a treatment group (receiving the new medication) or a control group (receiving a placebo). The primary outcome measure would be a change in depression scores, analyzed using statistical tests like t-tests or ANOVA to compare the means between groups. This is a classic large-n design focusing on establishing the overall effectiveness of the drug for a population.
-
An experiment examining the relationship between sleep deprivation and cognitive performance: Researchers might recruit a large sample of participants, randomly assigning them to different sleep deprivation conditions (e.g., 4 hours, 6 hours, 8 hours of sleep). Cognitive tests would be administered, and statistical analyses would be used to determine if there is a significant relationship between sleep duration and cognitive performance. Again, the emphasis is on generalizable findings across a population.
-
A survey exploring attitudes toward climate change: Thousands of individuals might be surveyed to determine their views on climate change, the potential causes, and their willingness to adopt environmentally friendly practices. Statistical analysis would focus on identifying trends and correlations in attitudes based on demographic variables (age, location, education, etc.). The interest lies in understanding the distribution of attitudes within a large population.
-
A randomized controlled trial (RCT) evaluating a new teaching method: A large number of students would be randomly assigned to different classrooms using either the traditional method or the new method. Their academic performance would be measured and statistically compared to see if the new method produces better results on average.
Examples of Small-N Designs:
-
A case study of a child with autism spectrum disorder: Researchers might conduct an intensive, in-depth analysis of a single child's behavior, utilizing multiple assessment methods and interventions to understand the child's unique challenges and responses to treatment. The focus is on understanding the individual child, not generalizing to all children with autism.
-
A study examining the effects of a behavioral intervention on a single patient with obsessive-compulsive disorder (OCD): This might involve carefully monitoring the patient's compulsive behaviors before, during, and after the intervention. The goal is to demonstrate a clear cause-and-effect relationship between the intervention and a reduction in compulsive behaviors within that specific individual.
-
Research examining the impact of a new prosthetic device on a single amputee's motor function: Detailed measurements of the amputee's motor skills would be taken before and after using the prosthetic. The goal isn't to generalize to all amputees but to evaluate the device's efficacy for this particular individual.
-
An A-B-A-B reversal design investigating the effectiveness of a reward system on a child's disruptive classroom behavior: The child's disruptive behavior is measured during a baseline phase (A), then during a treatment phase (B) where the reward system is implemented, then back to a baseline phase (A), and finally back to a treatment phase (B). This design, a classic SCED, allows researchers to visually assess the impact of the reward system on the child's behavior by comparing the "A" and "B" phases.
Strengths and Weaknesses:
Large-N Design Strengths:
- High generalizability: Findings can often be generalized to a larger population.
- Statistical power: Large sample sizes provide more statistical power to detect significant effects.
- Objective analysis: Data analysis relies on objective statistical methods, minimizing researcher bias.
Large-N Design Weaknesses:
- Loss of individual detail: Individual participant data may be lost in the focus on group averages.
- Artificiality: The controlled settings needed for experiments might not accurately reflect real-world situations.
- Cost and time: Large-N studies can be expensive and time-consuming to conduct.
Small-N Design Strengths:
- Detailed individual data: Provides rich, in-depth understanding of individual behavior.
- High internal validity: Careful experimental control can establish strong causal inferences.
- Flexibility: Allows for tailoring interventions to individual needs and responses.
Small-N Design Weaknesses:
- Limited generalizability: Findings may not generalize to other individuals or populations.
- Low statistical power: Small sample sizes can make it difficult to detect statistically significant effects.
- Potential for researcher bias: Subjective interpretation of data can be a concern.
Choosing Between Small-N and Large-N Designs:
The choice between small-n and large-n designs depends on the research question and the resources available.
-
Large-N designs are best suited for questions aimed at understanding general population effects and establishing broad relationships between variables. They require substantial resources but offer strong generalizability.
-
Small-N designs are ideal when detailed understanding of individual behavior is paramount and when resources are limited. They offer exceptional internal validity but sacrifice generalizability. They're perfect for situations requiring tailored interventions or exploring rare phenomena.
Conclusion:
Both small-n and large-n designs have valuable contributions to make to scientific knowledge. The choice between them is not a matter of one being superior to the other, but rather a decision based on the specific research goals, the nature of the phenomenon under investigation, and the available resources. Understanding the strengths and limitations of each approach is critical for researchers to select the most appropriate methodology for their studies. By carefully considering the research question and the characteristics of each design, researchers can ensure their findings are both meaningful and reliable.
Latest Posts
Latest Posts
-
What Other Emergency Vehicle Types Require The Same Response
May 25, 2025
-
Aristotle Nicomachean Ethics Book 2 Summary
May 25, 2025
-
Mice And Men Chapter 4 Summary
May 25, 2025
-
Chapter 9 Of Into The Wild
May 25, 2025
-
Quran And Bible Similarities And Differences
May 25, 2025
Related Post
Thank you for visiting our website which covers about Identify The Following Examples As Either Small-n Or Large-n Designs. . We hope the information provided has been useful to you. Feel free to contact us if you have any questions or need further assistance. See you next time and don't miss to bookmark.