How To Calculate Mad In Forecasting
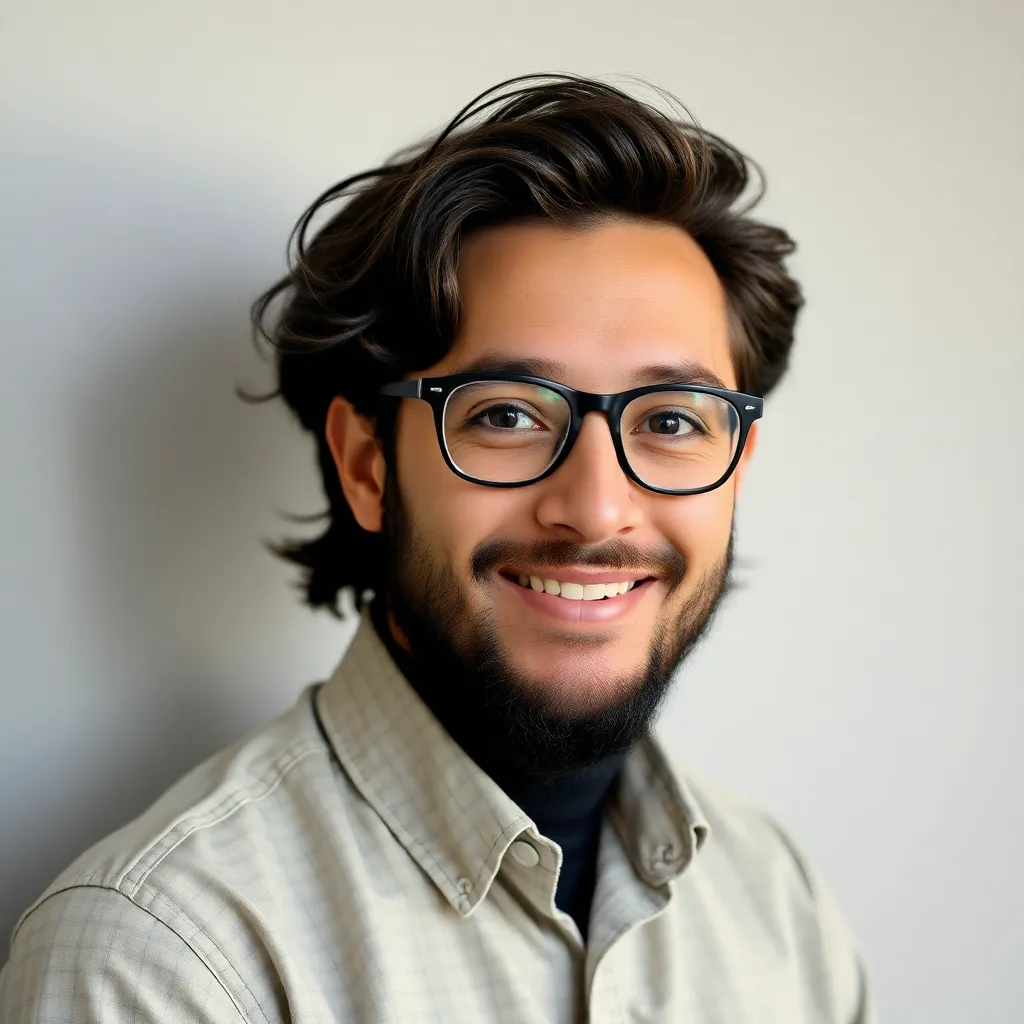
Juapaving
Apr 13, 2025 · 6 min read

Table of Contents
How to Calculate MAD in Forecasting: A Comprehensive Guide
Forecasting accuracy is crucial in various fields, from business and finance to weather prediction and supply chain management. A key metric used to assess the accuracy of a forecasting model is the Mean Absolute Deviation (MAD). Understanding how to calculate and interpret MAD is essential for improving forecasting processes and making better data-driven decisions. This comprehensive guide will delve into the intricacies of MAD calculation, its advantages and limitations, and provide practical examples to solidify your understanding.
What is Mean Absolute Deviation (MAD)?
The Mean Absolute Deviation (MAD) is a simple yet powerful measure of the average absolute difference between the forecasted values and the actual values. In simpler terms, it quantifies the average forecast error. A lower MAD indicates a more accurate forecasting model, suggesting that the predictions are, on average, closer to the actual outcomes. Conversely, a higher MAD signifies larger forecast errors and less reliable predictions.
Why Use MAD?
MAD offers several advantages:
- Simplicity and Ease of Calculation: MAD is relatively straightforward to calculate, making it accessible even without advanced statistical knowledge.
- Intuitive Interpretation: The result of MAD is easily interpretable; it represents the average absolute error in the same units as the data being forecasted. This makes it easier to communicate the accuracy of forecasts to both technical and non-technical audiences.
- Robustness to Outliers: While sensitive to outliers to some degree, MAD is less sensitive than other measures like the standard deviation, as it uses absolute values rather than squared differences.
When is MAD Most Useful?
MAD shines when:
- Simplicity is Paramount: When a quick and easily understood accuracy measure is needed.
- Outlier Sensitivity is a Concern: While not completely immune, MAD is less affected by extreme values than some alternative measures.
- Understanding Average Forecast Error is Crucial: MAD directly addresses the average magnitude of forecast errors, providing a clear picture of forecast accuracy.
How to Calculate MAD: A Step-by-Step Guide
Calculating MAD involves these steps:
-
Gather your data: You need two sets of data: the actual values and the forecasted values for the same period. Ensure both datasets have the same number of data points.
-
Calculate the forecast errors: For each time period, subtract the forecasted value from the actual value. This results in a series of forecast errors (also known as residuals). The formula is:
Error = Actual Value - Forecasted Value
-
Calculate the absolute errors: Take the absolute value of each forecast error. This removes the negative signs, ensuring that both positive and negative errors contribute equally to the MAD calculation.
-
Sum the absolute errors: Add up all the absolute errors calculated in the previous step.
-
Calculate the mean absolute deviation: Divide the sum of absolute errors by the number of data points (n). The formula for MAD is:
MAD = (Σ |Actual Value - Forecasted Value|) / n
Where:
- Σ represents the summation
- | | represents the absolute value
- n is the number of data points
Example Calculation of MAD
Let's illustrate with a simple example. Suppose we're forecasting monthly sales for a product. The following table shows the actual sales and the forecasted sales for the past six months:
Month | Actual Sales | Forecasted Sales | Error (Actual - Forecasted) | Absolute Error |
---|---|---|---|---|
January | 100 | 90 | 10 | 10 |
February | 110 | 105 | 5 | 5 |
March | 120 | 115 | 5 | 5 |
April | 130 | 135 | -5 | 5 |
May | 140 | 140 | 0 | 0 |
June | 150 | 145 | 5 | 5 |
Calculation:
-
Sum of Absolute Errors: 10 + 5 + 5 + 5 + 0 + 5 = 30
-
Number of Data Points (n): 6
-
MAD: 30 / 6 = 5
Therefore, the MAD for this forecast is 5 units. This indicates that, on average, the forecast deviates from the actual sales by 5 units.
Interpreting MAD Results
The interpretation of MAD depends on the context and the scale of the data. A MAD of 5 in the above example might be considered good or bad depending on the typical sales volume. A MAD of 5 for a product with average monthly sales of 1000 would be excellent, while the same MAD for a product with average monthly sales of 20 would be quite high, indicating a significant forecasting inaccuracy.
It's crucial to compare the MAD of different forecasting models to determine which model provides the most accurate predictions. A lower MAD signifies better forecasting accuracy. Furthermore, it's helpful to track MAD over time to monitor the performance of the forecasting model and identify potential areas for improvement.
Limitations of MAD
While MAD is a valuable tool, it has limitations:
- Sensitivity to Scale: MAD's value depends on the scale of the data. Comparing MAD across datasets with different scales can be misleading.
- Lack of Statistical Properties: Unlike the standard deviation, MAD does not have well-defined statistical properties, making it less suitable for certain statistical analyses.
- Doesn't Differentiate between Positive and Negative Errors: While taking the absolute value addresses the problem of cancellation between positive and negative errors, it loses information about the direction of the errors. Understanding whether the forecast consistently overestimates or underestimates is often valuable.
MAD vs. Other Forecasting Accuracy Measures
MAD is often compared with other accuracy measures, each with its strengths and weaknesses:
- Mean Squared Error (MSE): MSE squares the errors before averaging them, giving greater weight to larger errors. This makes MSE more sensitive to outliers.
- Root Mean Squared Error (RMSE): RMSE is the square root of MSE, returning the error in the same units as the data. It's also more sensitive to outliers than MAD.
- Mean Absolute Percentage Error (MAPE): MAPE expresses errors as percentages, making it easier to compare accuracy across datasets with different scales. However, MAPE can be undefined or misleading when actual values are close to zero.
The choice of the most appropriate accuracy measure depends on the specific needs and context of the forecasting problem.
Improving Forecasting Accuracy using MAD
MAD can be used to improve forecasting accuracy through iterative processes:
-
Model Selection: Compare MAD values across different forecasting models (e.g., simple moving average, exponential smoothing, ARIMA) to identify the model with the lowest MAD.
-
Parameter Tuning: For models with adjustable parameters (like exponential smoothing), adjust the parameters to minimize the MAD.
-
Data Preprocessing: Investigate potential data issues like outliers or seasonality that might be affecting the accuracy of the forecasts and make necessary adjustments to the data before modeling.
-
Feature Engineering: Consider adding relevant predictor variables to improve forecast accuracy.
-
Model Refinement: Based on the MAD values and error analysis, refine the chosen forecasting model to improve its predictive capabilities.
Conclusion
The Mean Absolute Deviation (MAD) is a valuable tool for evaluating the accuracy of forecasting models. Its simplicity, ease of calculation, and intuitive interpretation make it a widely used metric in various fields. While it has limitations, understanding how to calculate and interpret MAD is essential for developing and improving forecasting processes, leading to better decision-making and improved performance. By comparing MAD across different models and consistently monitoring its value, you can refine your forecasting techniques and achieve greater predictive accuracy. Remember to consider the context and limitations of MAD and potentially explore other accuracy metrics to gain a comprehensive understanding of your forecast's performance.
Latest Posts
Latest Posts
-
Which Of The Following Is Incorrectly
Apr 15, 2025
-
How Do You Separate Sugar And Water
Apr 15, 2025
-
How Many Neutrons Does Mercury Have
Apr 15, 2025
-
4062 Divided By 81 With Remainder
Apr 15, 2025
-
What Is The Difference Between Multiple Alleles And Polygenic Traits
Apr 15, 2025
Related Post
Thank you for visiting our website which covers about How To Calculate Mad In Forecasting . We hope the information provided has been useful to you. Feel free to contact us if you have any questions or need further assistance. See you next time and don't miss to bookmark.