How Do You Analyze A Graph
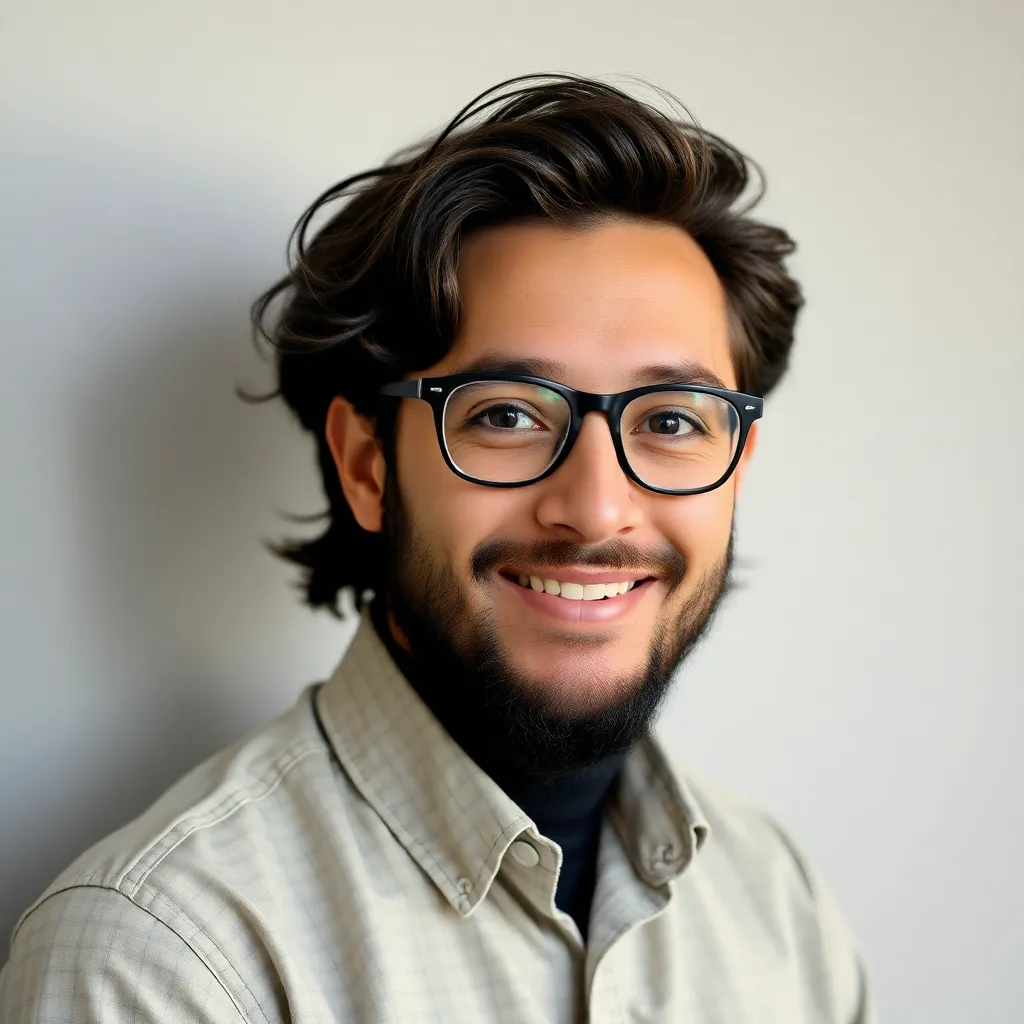
Juapaving
Apr 08, 2025 · 6 min read

Table of Contents
How Do You Analyze a Graph? A Comprehensive Guide
Graphs are ubiquitous. From visualizing stock market trends to depicting social network connections, understanding how to analyze a graph is a crucial skill across numerous fields. This comprehensive guide will delve into the multifaceted process of graph analysis, covering various techniques and considerations, regardless of your experience level.
Understanding the Basics: Types of Graphs and Their Components
Before diving into analytical methods, it's crucial to understand the different types of graphs and their key components.
Types of Graphs
-
Directed Graphs (Digraphs): These graphs represent relationships with directionality. For instance, a graph showing website links would be directed, as Link A pointing to Link B is different from Link B pointing to Link A. Arrows indicate the direction of the relationship.
-
Undirected Graphs: In these graphs, relationships are bidirectional. Think of a social network where friendship is reciprocal; if A is friends with B, B is also friends with A. Edges (lines connecting nodes) have no inherent direction.
-
Weighted Graphs: These graphs assign numerical values (weights) to edges, representing the strength or cost of a relationship. Examples include road networks where weights represent distances or transportation costs.
-
Unweighted Graphs: These graphs simply show the presence or absence of a relationship; edges lack numerical values.
Key Components
-
Nodes (Vertices): These are the individual entities represented within the graph. They can represent anything from people in a social network to cities in a transportation network.
-
Edges (Arcs): These are the connections between nodes. They represent relationships, interactions, or links between the entities.
Essential Graph Analysis Techniques
Numerous techniques exist for analyzing graphs, each offering unique insights. We'll explore some of the most commonly used methods:
1. Degree Centrality: Measuring Node Importance
Degree centrality is a fundamental concept, assessing the importance of a node based on the number of connections it possesses.
-
In-degree (Directed Graphs): The number of incoming edges to a node. High in-degree suggests the node is a popular recipient of connections.
-
Out-degree (Directed Graphs): The number of outgoing edges from a node. High out-degree suggests the node is actively connecting to others.
-
Degree (Undirected Graphs): The total number of edges connected to a node. This is a simple yet effective measure of influence or importance within the network.
Example: In a social network, a node with high degree centrality might be considered an influential individual.
2. Betweenness Centrality: Identifying Bridge Nodes
Betweenness centrality quantifies the influence of a node based on its position within the network. Nodes with high betweenness centrality act as bridges, connecting otherwise disparate parts of the network. Removing these nodes can significantly disrupt the network's connectivity.
Example: In a transportation network, a city with high betweenness centrality serves as a crucial transit hub. Disrupting this hub could significantly impact overall connectivity.
3. Closeness Centrality: Measuring Proximity to Others
Closeness centrality measures the average distance from a node to all other nodes within the network. Nodes with high closeness centrality are easily accessible from other parts of the network. They are centrally located and can quickly reach other nodes.
Example: In a communication network, a node with high closeness centrality can rapidly disseminate information throughout the network.
4. Eigenvector Centrality: Considering the Influence of Connections
Eigenvector centrality goes beyond simply counting connections. It takes into account the importance of a node's neighbors. A node connected to many important nodes itself becomes important. This measure helps identify influential nodes within the network, reflecting the quality of their connections.
Example: In a citation network, a paper cited by many highly cited papers will have a high eigenvector centrality score.
5. Clustering Coefficient: Assessing Local Network Density
The clustering coefficient measures the interconnectedness of a node's immediate neighbors. A high clustering coefficient indicates that a node's neighbors are heavily connected to each other, forming a dense cluster.
Example: In a social network, a high clustering coefficient might indicate the presence of strong social bonds within a group of friends.
6. Pathfinding Algorithms: Finding Shortest Routes
Pathfinding algorithms, such as Dijkstra's algorithm and breadth-first search, are crucial for analyzing networks with a focus on finding the shortest paths between nodes. These algorithms are vital for optimizing transportation, communication, and network routing.
7. Community Detection: Identifying Groups within the Network
Community detection algorithms help identify groups or clusters of densely connected nodes within a larger network. These algorithms are useful for understanding social structures, identifying market segments, and uncovering hidden patterns.
8. Network Visualization: The Power of Graphical Representation
Effective visualization is crucial for understanding complex networks. Different visualization techniques can highlight specific aspects of the network structure, making it easier to identify patterns and insights. Tools like Gephi and Cytoscape are invaluable for creating and analyzing network visualizations.
Advanced Graph Analysis Techniques
Beyond the fundamental methods, advanced techniques offer deeper insights:
1. Graph Embeddings: Representing Nodes as Vectors
Graph embeddings convert nodes into low-dimensional vector representations, capturing their structural and relational context within the graph. These vectors can then be used as input for machine learning models to perform tasks such as node classification, link prediction, and anomaly detection.
2. Graph Neural Networks (GNNs): Leveraging Deep Learning for Graph Data
GNNs are a powerful class of deep learning models specifically designed for processing graph-structured data. They can learn complex patterns and relationships within the network, enabling advanced tasks like graph classification, node classification, and graph generation.
Interpreting the Results and Drawing Conclusions
Analyzing a graph isn't just about calculating metrics; it's about interpreting those metrics in the context of the problem.
-
Context is Key: Always consider the real-world meaning behind the nodes and edges. The interpretation of centrality measures, for example, depends heavily on the nature of the network.
-
Look for Patterns and Anomalies: Analyze the distribution of metrics, looking for nodes or groups of nodes that deviate significantly from the average. These anomalies can reveal crucial insights.
-
Correlation vs. Causation: Remember that correlation doesn't imply causation. Just because two nodes are highly connected doesn't necessarily mean one causes the other.
-
Iterative Analysis: Graph analysis is often an iterative process. Initial findings may lead to new questions and further analysis, refining your understanding of the network.
Tools for Graph Analysis
Numerous software tools and libraries are available to aid in graph analysis, ranging from user-friendly visualization tools to powerful programming libraries:
-
Gephi: A powerful open-source software for visualizing and analyzing large graphs.
-
Cytoscape: Another popular open-source platform for network visualization and analysis.
-
NetworkX (Python): A comprehensive Python library for creating, manipulating, and analyzing graphs.
-
igraph (R, Python): A versatile library available in multiple programming languages for graph analysis.
Conclusion
Analyzing a graph is a multifaceted process requiring a nuanced understanding of the data and the appropriate analytical techniques. By mastering the techniques discussed in this guide and leveraging available tools, you can unlock valuable insights from diverse graph-structured data, leading to better decision-making across numerous fields. Remember that the process is often iterative, with initial analysis leading to further questions and a deeper understanding of the underlying network structure. The journey of graph analysis is one of continuous exploration and discovery.
Latest Posts
Latest Posts
-
What Does 1 1 Ratio Mean
Apr 17, 2025
-
What Are The Least Common Multiples Of 12 And 18
Apr 17, 2025
-
As Altitude Increases What Happens To Air Pressure
Apr 17, 2025
-
Which Rna Has A Clover Leaf Structure
Apr 17, 2025
-
Chart Of Perfect Squares And Cubes
Apr 17, 2025
Related Post
Thank you for visiting our website which covers about How Do You Analyze A Graph . We hope the information provided has been useful to you. Feel free to contact us if you have any questions or need further assistance. See you next time and don't miss to bookmark.