Find Ta Df From The Following Information
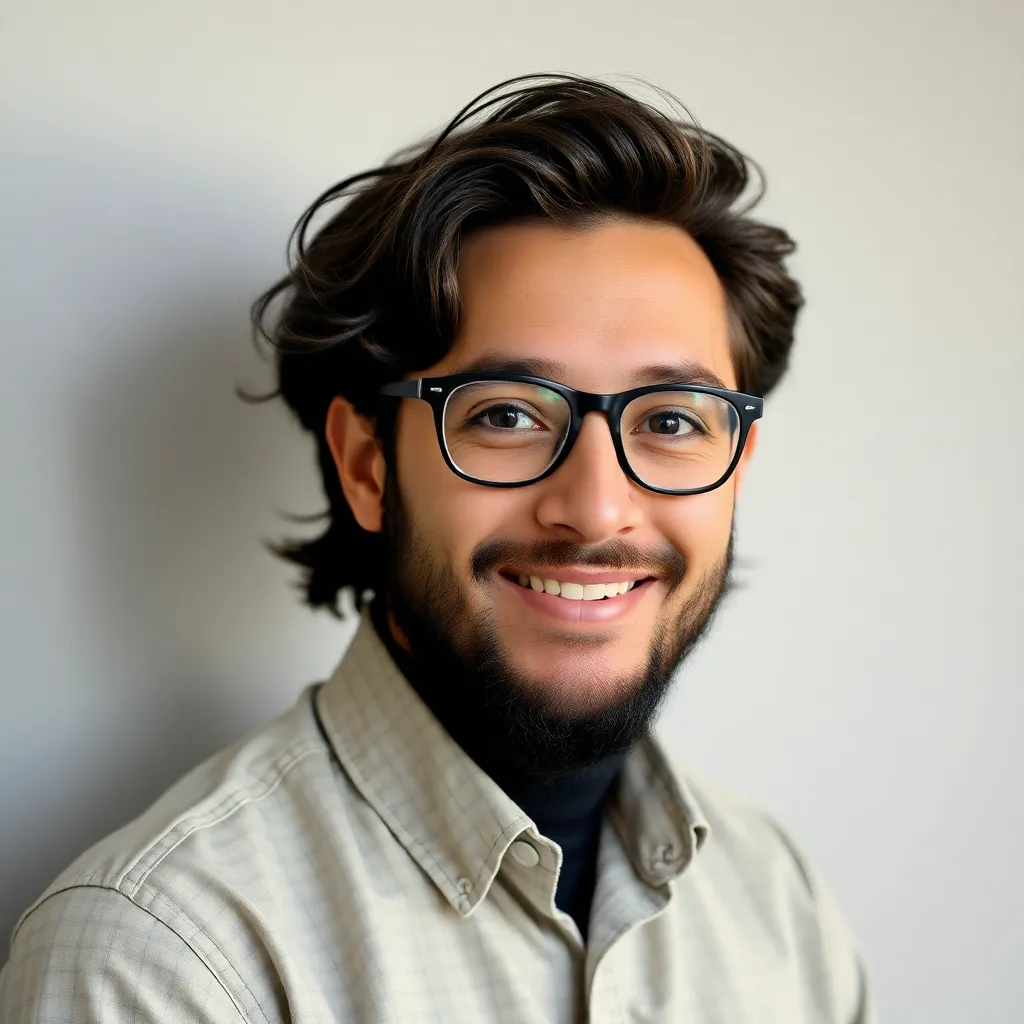
Juapaving
May 27, 2025 · 6 min read
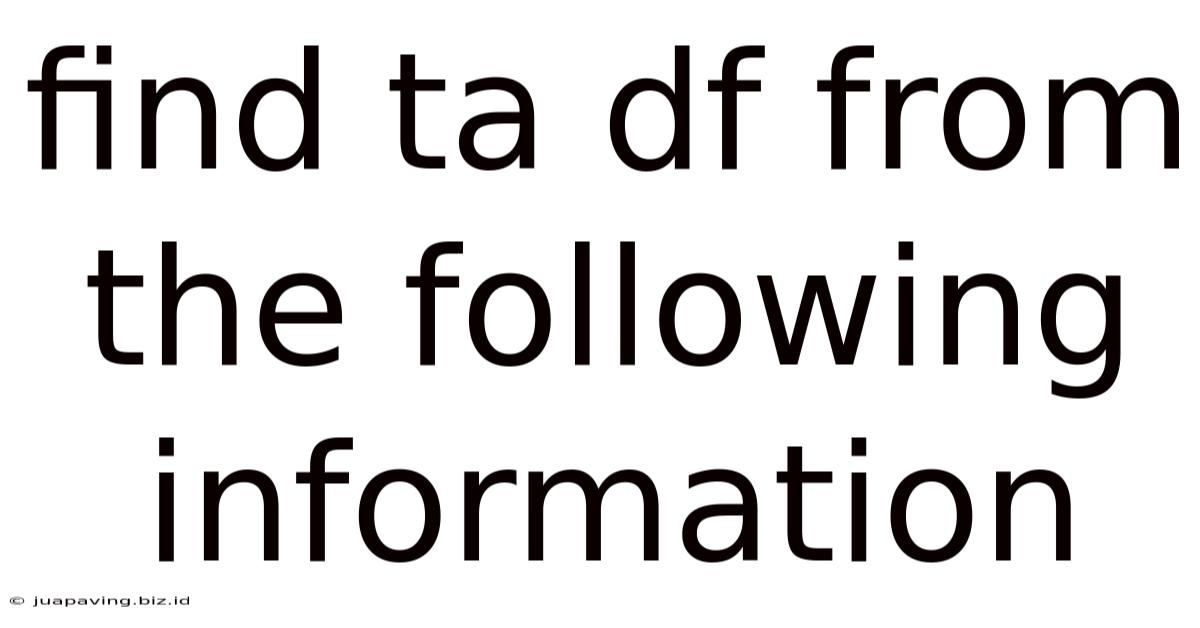
Table of Contents
Finding the Cumulative Distribution Function (CDF) from Given Information
This article comprehensively explores various methods for determining the cumulative distribution function (CDF) from different types of given information. The CDF is a fundamental concept in probability and statistics, providing the probability that a random variable takes on a value less than or equal to a specified value. Understanding how to derive the CDF from various inputs is crucial for various statistical analyses and applications. We'll cover scenarios involving probability mass functions (PMFs), probability density functions (PDFs), and empirical data.
Understanding the Cumulative Distribution Function (CDF)
Before delving into the methods, let's briefly recap the definition of the CDF. For a random variable X, the CDF, denoted as F<sub>X</sub>(x), is defined as:
F<sub>X</sub>(x) = P(X ≤ x)
This means the CDF at a specific value 'x' gives the probability that the random variable X will take on a value less than or equal to 'x'. The CDF possesses several key properties:
- Non-decreasing: F<sub>X</sub>(x) ≤ F<sub>X</sub>(y) if x ≤ y.
- Right-continuous: lim<sub>t→x<sup>+</sup></sub> F<sub>X</sub>(t) = F<sub>X</sub>(x)
- Limits: lim<sub>x→-∞</sub> F<sub>X</sub>(x) = 0 and lim<sub>x→∞</sub> F<sub>X</sub>(x) = 1
These properties are vital for verifying the correctness of a derived CDF.
Method 1: Deriving the CDF from a Probability Mass Function (PMF)
A PMF is used for discrete random variables. It specifies the probability of each individual outcome. To obtain the CDF from a PMF, we simply sum the probabilities of all outcomes less than or equal to the specified value.
Example:
Let's say we have a discrete random variable X representing the number of heads obtained when flipping a fair coin three times. The PMF is:
- P(X=0) = 1/8
- P(X=1) = 3/8
- P(X=2) = 3/8
- P(X=3) = 1/8
To find the CDF, F<sub>X</sub>(x):
- F<sub>X</sub>(0) = P(X ≤ 0) = P(X=0) = 1/8
- F<sub>X</sub>(1) = P(X ≤ 1) = P(X=0) + P(X=1) = 1/8 + 3/8 = 4/8 = 1/2
- F<sub>X</sub>(2) = P(X ≤ 2) = P(X=0) + P(X=1) + P(X=2) = 1/8 + 3/8 + 3/8 = 7/8
- F<sub>X</sub>(3) = P(X ≤ 3) = P(X=0) + P(X=1) + P(X=2) + P(X=3) = 1/8 + 3/8 + 3/8 + 1/8 = 8/8 = 1
Therefore, the CDF is:
F<sub>X</sub>(x) = 0, for x < 0 = 1/8, for 0 ≤ x < 1 = 1/2, for 1 ≤ x < 2 = 7/8, for 2 ≤ x < 3 = 1, for x ≥ 3
Method 2: Deriving the CDF from a Probability Density Function (PDF)
A PDF is used for continuous random variables. It describes the probability density at each point. To find the CDF from a PDF, we integrate the PDF from negative infinity to the specified value 'x'.
Example:
Consider a continuous random variable X with the following PDF:
f<sub>X</sub>(x) = 2x, for 0 ≤ x ≤ 1 = 0, otherwise
To find the CDF, F<sub>X</sub>(x):
For 0 ≤ x ≤ 1:
F<sub>X</sub>(x) = ∫<sub>0</sub><sup>x</sup> 2t dt = [t<sup>2</sup>]<sub>0</sub><sup>x</sup> = x<sup>2</sup>
For x < 0: F<sub>X</sub>(x) = 0
For x > 1: F<sub>X</sub>(x) = ∫<sub>0</sub><sup>1</sup> 2t dt = [t<sup>2</sup>]<sub>0</sub><sup>1</sup> = 1
Therefore, the CDF is:
F<sub>X</sub>(x) = 0, for x < 0 = x<sup>2</sup>, for 0 ≤ x ≤ 1 = 1, for x > 1
Method 3: Estimating the CDF from Empirical Data
When dealing with empirical data (a sample from a population), we can estimate the CDF by calculating the proportion of observations less than or equal to a given value. This is often represented as an empirical cumulative distribution function (ECDF).
Example:
Let's say we have the following sample data: {2, 5, 3, 8, 4, 6, 7, 5, 2, 9}
First, sort the data: {2, 2, 3, 4, 5, 5, 6, 7, 8, 9}
To estimate the ECDF:
- F<sub>X</sub>(2) ≈ 2/10 = 0.2 (2 values are less than or equal to 2)
- F<sub>X</sub>(3) ≈ 3/10 = 0.3 (3 values are less than or equal to 3)
- F<sub>X</sub>(4) ≈ 4/10 = 0.4
- F<sub>X</sub>(5) ≈ 6/10 = 0.6
- F<sub>X</sub>(6) ≈ 7/10 = 0.7
- F<sub>X</sub>(7) ≈ 8/10 = 0.8
- F<sub>X</sub>(8) ≈ 9/10 = 0.9
- F<sub>X</sub>(9) ≈ 10/10 = 1.0
The ECDF provides an approximation of the true CDF based on the available sample. Larger sample sizes generally lead to more accurate estimations.
Applications of the CDF
The CDF is a versatile tool with wide-ranging applications in various fields, including:
- Risk Assessment: Assessing the probability of exceeding a certain threshold (e.g., in financial modeling).
- Reliability Engineering: Determining the probability of a component failing before a certain time.
- Quality Control: Analyzing the distribution of product characteristics to identify defects.
- Hypothesis Testing: Comparing distributions to test hypotheses about population parameters.
- Machine Learning: Evaluating model performance and generating predictions.
Challenges and Considerations
While calculating the CDF is generally straightforward, certain challenges can arise:
- Complex PDFs: Integrating complex PDFs can be mathematically challenging, requiring numerical integration techniques.
- Limited Data: When working with limited empirical data, the ECDF might not accurately represent the true CDF. Statistical methods like kernel density estimation can help improve accuracy.
- Censoring and Truncation: Dealing with censored or truncated data requires adjustments to the CDF estimation methods.
- Multivariate Distributions: For random variables with multiple dimensions, the CDF becomes more complex, requiring multivariate integration or estimation techniques.
Conclusion
Finding the CDF is a fundamental task in probability and statistics. The appropriate method depends on the type of information available: a PMF for discrete variables, a PDF for continuous variables, or empirical data for sample estimations. Understanding the properties of the CDF and potential challenges is crucial for its accurate determination and effective application in various contexts. The applications of the CDF are extensive, making it a critical tool for data analysis, modeling, and decision-making in numerous fields. The examples and methods described here provide a robust foundation for tackling CDF calculations and interpretations effectively. Remember to always check the calculated CDF against its fundamental properties to ensure accuracy and validity. This ensures a more reliable and meaningful interpretation of the data.
Latest Posts
Latest Posts
-
Placebo Effect Therapy Uses The Principle Of
May 28, 2025
-
Gizmo Coastal Winds And Clouds Answer Key
May 28, 2025
-
Developments In South And Southeast Asia
May 28, 2025
-
Why Does Beowulf Fight Grendel Without A Sword
May 28, 2025
-
Completa Cada Oraci N Con La Opci N Correcta
May 28, 2025
Related Post
Thank you for visiting our website which covers about Find Ta Df From The Following Information . We hope the information provided has been useful to you. Feel free to contact us if you have any questions or need further assistance. See you next time and don't miss to bookmark.