Examples Of Experimental And Theoretical Probability
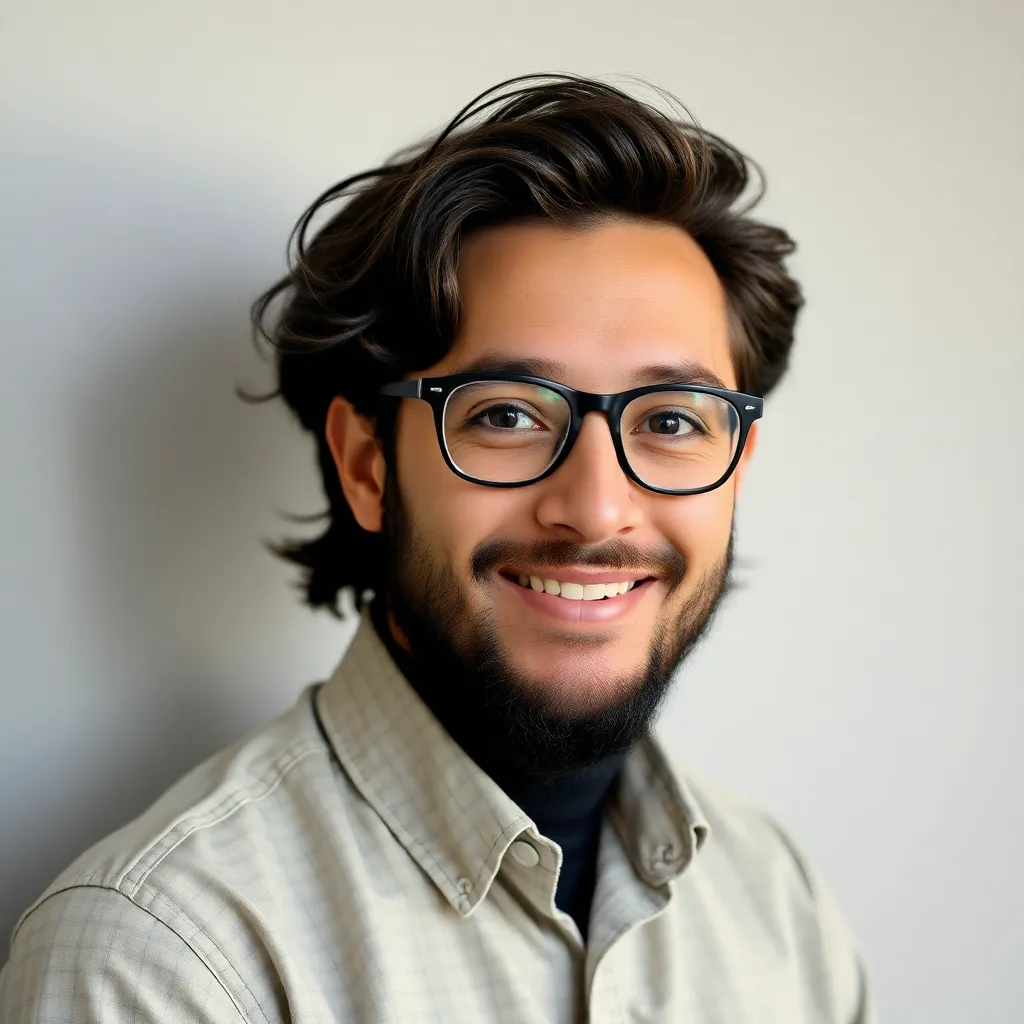
Juapaving
May 12, 2025 · 7 min read
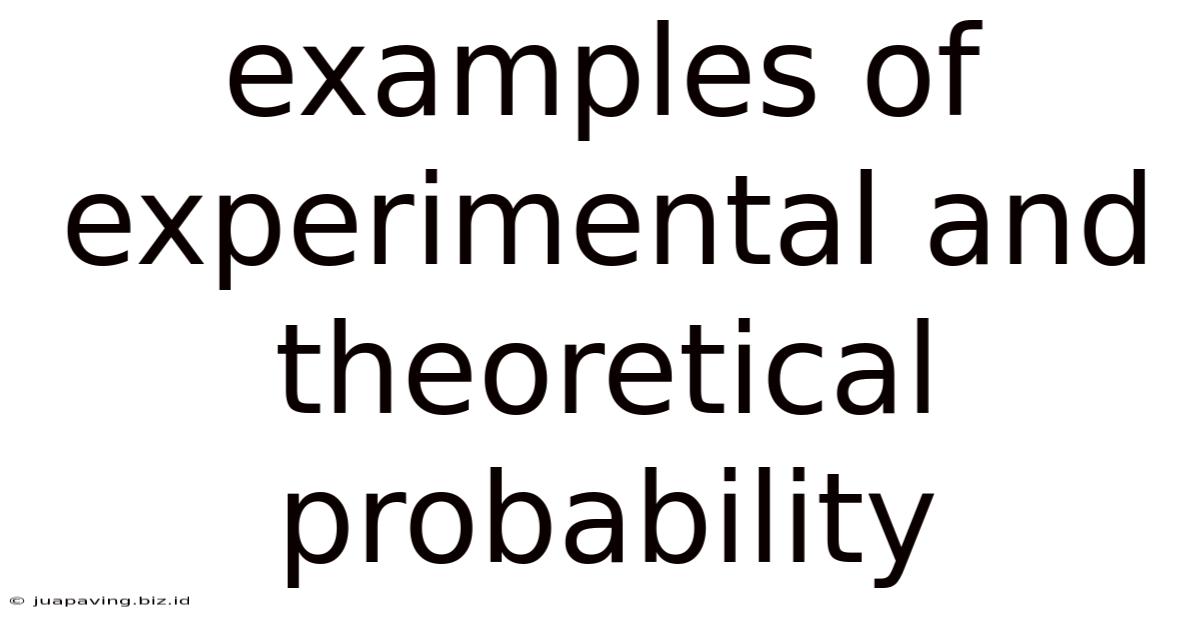
Table of Contents
Examples of Experimental and Theoretical Probability
Probability, a cornerstone of mathematics and statistics, quantifies the likelihood of an event occurring. It's a fundamental concept used across various fields, from weather forecasting to medical research and even gambling. Understanding probability involves grasping two key types: experimental and theoretical probability. While both relate to the chance of an event, their approaches differ significantly. This article delves into the nuances of each, providing numerous real-world examples to solidify your comprehension.
Theoretical Probability: The Expected Outcome
Theoretical probability relies on logic and reasoning to predict the likelihood of an event. It assumes a perfectly fair and unbiased scenario. The calculation is straightforward:
Theoretical Probability = (Number of favorable outcomes) / (Total number of possible outcomes)
Let's illustrate with several examples:
Example 1: Coin Toss
Consider a fair coin toss. The theoretical probability of getting heads is:
- Favorable outcome: Getting heads (1)
- Total possible outcomes: Heads or tails (2)
- Theoretical Probability of heads: 1/2 or 50%
Similarly, the theoretical probability of getting tails is also 1/2 or 50%. This is because we assume the coin is perfectly balanced, giving each outcome an equal chance.
Example 2: Rolling a Die
When rolling a standard six-sided die, the theoretical probability of rolling a specific number (e.g., a 3) is:
- Favorable outcome: Rolling a 3 (1)
- Total possible outcomes: Rolling a 1, 2, 3, 4, 5, or 6 (6)
- Theoretical Probability of rolling a 3: 1/6
The probability of rolling any specific number is 1/6, while the probability of rolling an even number (2, 4, or 6) is 3/6, which simplifies to 1/2.
Example 3: Drawing Cards from a Deck
From a standard deck of 52 playing cards, the theoretical probability of drawing a specific card (e.g., the Ace of Spades) is:
- Favorable outcome: Drawing the Ace of Spades (1)
- Total possible outcomes: 52 cards
- Theoretical Probability of drawing the Ace of Spades: 1/52
The probability of drawing a heart is 13/52 (1/4), as there are 13 hearts in a deck. The probability of drawing a King is 4/52 (1/13), as there are four Kings.
Experimental Probability: The Observed Outcome
Experimental probability, unlike its theoretical counterpart, is based on actual observations and experiments. It's the ratio of the number of times an event occurs to the total number of trials.
Experimental Probability = (Number of times the event occurred) / (Total number of trials)
The accuracy of experimental probability increases with the number of trials. The more trials conducted, the closer the experimental probability gets to the theoretical probability. However, it's crucial to remember that even with numerous trials, there's no guarantee of perfect alignment.
Example 1: Tossing a Coin (Experiment)
Let's say you toss a coin 100 times. You observe 53 heads and 47 tails. The experimental probability of getting heads is:
- Number of heads: 53
- Total number of tosses: 100
- Experimental Probability of heads: 53/100 or 53%
Note that this differs from the theoretical probability of 50%. This difference arises due to random variation in the experiment. If you increased the number of tosses to 1000 or 10,000, the experimental probability would likely be closer to 50%.
Example 2: Rolling a Die (Experiment)
You roll a die 60 times. The results are:
- 1: 10 times
- 2: 8 times
- 3: 12 times
- 4: 9 times
- 5: 11 times
- 6: 10 times
The experimental probability of rolling a 3 is:
- Number of times a 3 was rolled: 12
- Total number of rolls: 60
- Experimental Probability of rolling a 3: 12/60 = 1/5 or 20%
Again, this differs from the theoretical probability of 1/6 (approximately 16.7%). The discrepancy is due to the limited number of trials.
Example 3: Analyzing Rainfall Data
Meteorologists use experimental probability extensively. If a city experienced rain on 30 out of 90 days in a particular season, the experimental probability of rain during that season is:
- Number of rainy days: 30
- Total number of days: 90
- Experimental Probability of rain: 30/90 = 1/3 or approximately 33.3%
This data helps predict future rainfall probabilities for that season, though variations are expected.
Comparing Theoretical and Experimental Probability
The core difference lies in their approach. Theoretical probability is a prediction based on ideal conditions, while experimental probability is an observation based on real-world trials. The relationship between them is crucial:
-
Large Number of Trials: As the number of trials in an experiment increases, the experimental probability tends to converge towards the theoretical probability. This is a fundamental principle of the Law of Large Numbers.
-
Bias and Error: Experimental probability can be influenced by various factors, including bias in the experimental setup or random chance. Theoretical probability, on the other hand, assumes an ideal, unbiased scenario.
-
Applications: Theoretical probability is useful for simpler scenarios with known outcomes, while experimental probability is essential for analyzing real-world data where the underlying mechanisms may be complex or unknown.
Advanced Examples and Applications
The concepts of theoretical and experimental probability extend far beyond simple coin tosses and dice rolls. Let's explore some more sophisticated applications:
Example 4: Genetics and Inheritance
In genetics, theoretical probability is used to predict the likelihood of offspring inheriting specific traits based on the parents' genotypes. Punnett squares, a common tool in genetics, employ theoretical probability to visualize the possible combinations of alleles in offspring. Experimental probability is used when examining the actual inheritance patterns in a large population. There might be deviations from the theoretical predictions due to factors like genetic drift or environmental influences.
Example 5: Medical Trials and Drug Efficacy
Clinical trials extensively use experimental probability. Researchers test a drug on a sample population and track its effectiveness. The experimental probability of the drug's success is calculated based on the number of successful outcomes (e.g., patients showing improvement) divided by the total number of participants. This probability helps determine the drug's efficacy and safety before widespread use.
Example 6: Quality Control in Manufacturing
In manufacturing, experimental probability is crucial for quality control. A company might randomly sample its products to test for defects. The experimental probability of finding a defective product is calculated from the sample, providing an estimate of the overall defect rate. This helps companies identify and address production issues.
Example 7: Sports Statistics and Predictions
Sports analysts use experimental probability to predict game outcomes and player performance. A player's batting average in baseball, for example, is an experimental probability: the number of hits divided by the number of at-bats. This helps predict their likelihood of getting a hit in future games.
Example 8: Insurance and Risk Assessment
Insurance companies rely heavily on probability to assess risks and set premiums. They use experimental probability from historical data (e.g., accident rates, mortality rates) to estimate the likelihood of specific events. This allows them to set premiums that adequately cover potential payouts while maintaining profitability.
Example 9: Weather Forecasting
Meteorologists utilize both theoretical and experimental probability in weather forecasting. Theoretical models might predict the probability of rainfall based on atmospheric conditions, while experimental probability comes into play when analyzing historical weather data to refine these predictions.
Conclusion: Bridging the Gap Between Theory and Reality
Theoretical and experimental probability are complementary approaches to understanding chance. While theoretical probability provides a framework for ideal situations, experimental probability offers a way to test and refine these theoretical models using real-world observations. The strength of both lies in their combined application – using theoretical frameworks to guide experimentation and then using experimental results to refine and improve our understanding of the world around us. By mastering these concepts, you gain a powerful toolset for analyzing data, making predictions, and understanding the inherently uncertain nature of many events in life.
Latest Posts
Latest Posts
-
Is Cellular Respiration Exothermic Or Endothermic
May 14, 2025
-
Common Multiples Of 4 And 6
May 14, 2025
-
5 Letter Word Starting With H A
May 14, 2025
-
Describing Words That Begin With O
May 14, 2025
-
5 Letter Words Starting With Roo
May 14, 2025
Related Post
Thank you for visiting our website which covers about Examples Of Experimental And Theoretical Probability . We hope the information provided has been useful to you. Feel free to contact us if you have any questions or need further assistance. See you next time and don't miss to bookmark.