Example Of Inferential Statistics In Psychology
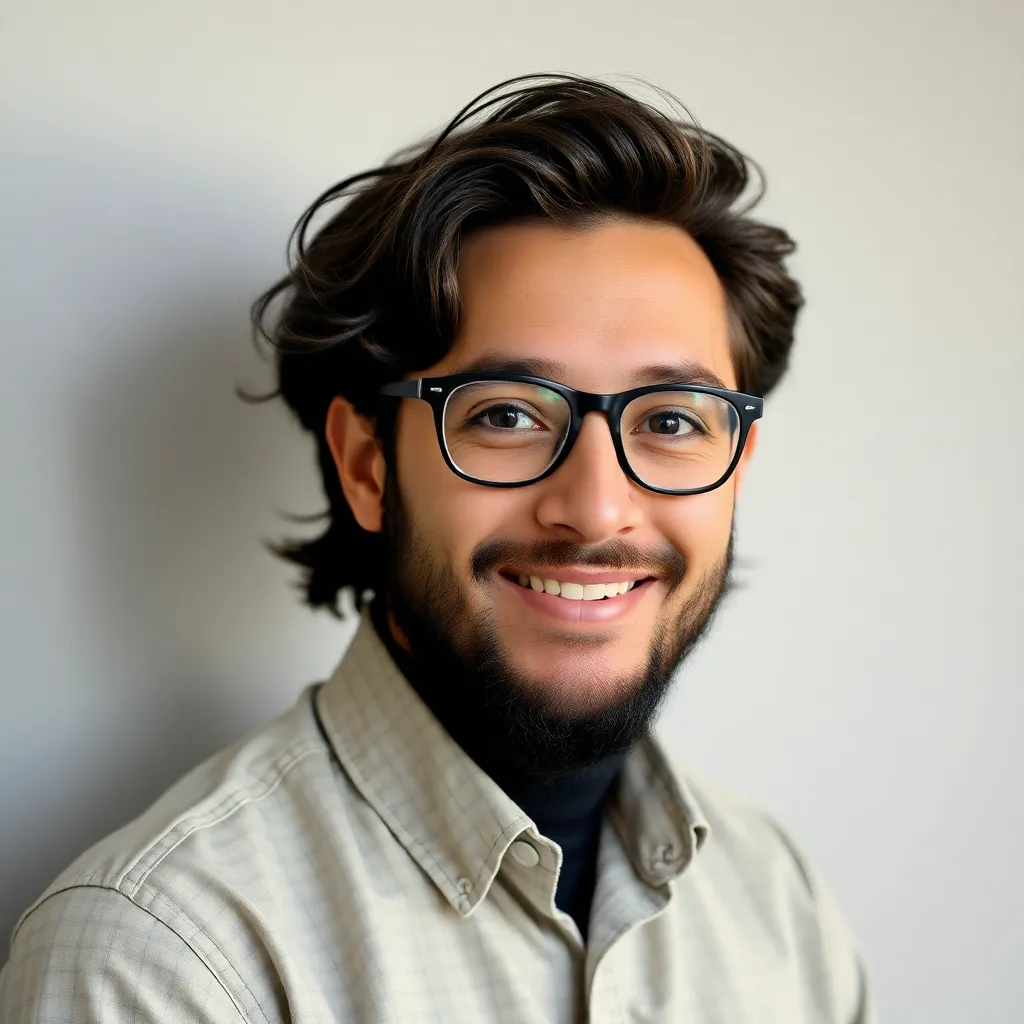
Juapaving
May 25, 2025 · 7 min read
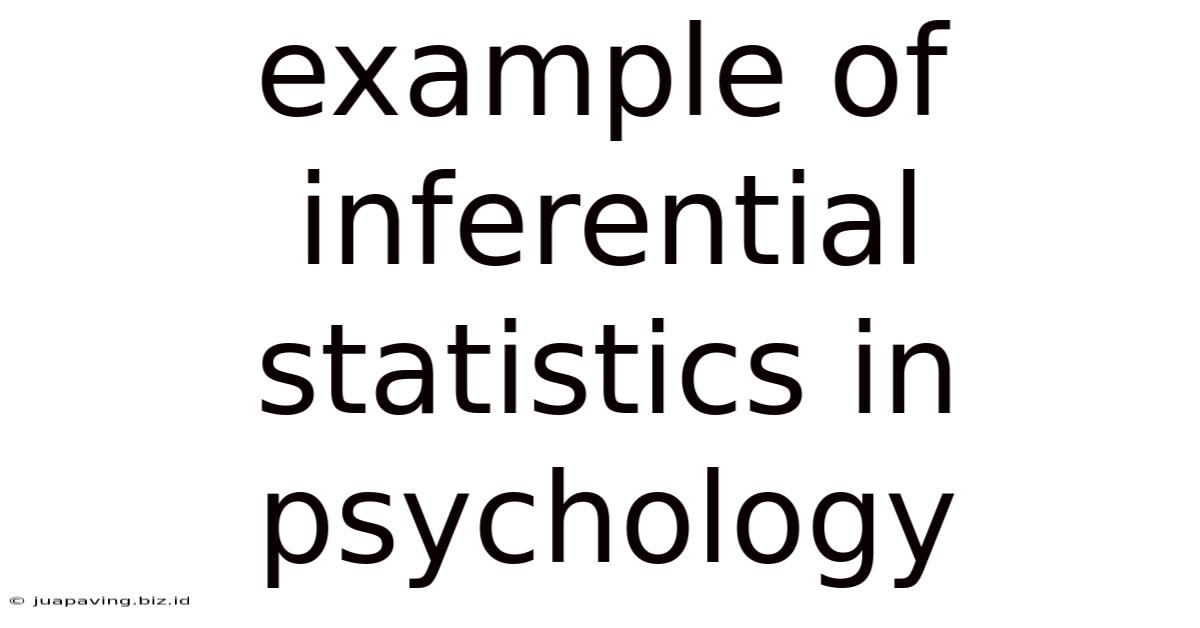
Table of Contents
Inferential Statistics in Psychology: Unveiling Patterns and Making Predictions
Inferential statistics play a crucial role in psychology, allowing researchers to move beyond simply describing data to drawing meaningful conclusions about populations based on sample data. Unlike descriptive statistics, which summarize data, inferential statistics help us make inferences, generalizations, and predictions about larger groups. This article explores various examples of inferential statistics commonly employed in psychological research, highlighting their applications and interpretations.
Understanding the Foundation: Hypothesis Testing
At the heart of inferential statistics lies hypothesis testing. This process involves formulating a testable hypothesis about a population parameter (e.g., the average IQ score of college students), collecting data from a representative sample, and then using statistical tests to determine the probability of observing the obtained results if the null hypothesis (typically stating no effect or difference) were true. If this probability (the p-value) is sufficiently low (typically below a pre-defined significance level, often 0.05), the null hypothesis is rejected, and the alternative hypothesis (the researcher's prediction) is supported.
Type I and Type II Errors
It's crucial to understand that hypothesis testing isn't foolproof. Two types of errors can occur:
-
Type I Error (False Positive): Rejecting the null hypothesis when it is actually true. This means concluding there's a significant effect when there isn't one. The probability of a Type I error is equal to the significance level (alpha).
-
Type II Error (False Negative): Failing to reject the null hypothesis when it is false. This means missing a real effect that actually exists. The probability of a Type II error is denoted by beta. Power (1-β) represents the probability of correctly rejecting a false null hypothesis.
Key Inferential Statistical Tests in Psychology
Numerous inferential statistical tests exist, each designed for specific types of data and research questions. Below are some prominent examples:
1. t-tests: Comparing Means
t-tests are used to compare the means of two groups. There are different types of t-tests:
-
Independent Samples t-test: Compares the means of two independent groups (e.g., comparing the anxiety levels of participants who received a relaxation technique versus those who did not).
- Example: A researcher wants to investigate the effectiveness of cognitive behavioral therapy (CBT) in reducing depression symptoms. They randomly assign participants to either a CBT group or a control group receiving no treatment. After the intervention, they compare the mean depression scores of the two groups using an independent samples t-test. A significant t-test result would suggest CBT is effective.
-
Paired Samples t-test: Compares the means of two related groups (e.g., comparing the same participants' pre- and post-treatment scores).
- Example: A study examines the impact of a new sleep training program on insomnia. Researchers measure participants' sleep quality before and after the program using a standardized sleep questionnaire. A paired samples t-test would analyze whether there's a statistically significant improvement in sleep quality from pre- to post-intervention.
2. Analysis of Variance (ANOVA): Comparing Means Across Multiple Groups
ANOVA extends the t-test to compare the means of three or more groups.
-
One-way ANOVA: Compares means across different levels of one independent variable (e.g., comparing the performance of students under three different teaching methods).
- Example: A psychologist investigates the effects of different learning environments (online, hybrid, in-person) on student engagement. They collect engagement scores from students in each learning environment and use a one-way ANOVA to determine if there are significant differences in engagement levels across the three groups.
-
Two-way ANOVA: Compares means across different levels of two or more independent variables (e.g., investigating the interaction effects of teaching method and student motivation on academic performance).
- Example: Expanding the previous example, a researcher could also incorporate student motivation levels (high vs. low) as a second independent variable. A two-way ANOVA would analyze the main effects of teaching method and motivation, as well as their interaction effect on student engagement.
3. Correlation: Measuring the Relationship Between Variables
Correlation assesses the strength and direction of the linear relationship between two continuous variables. The correlation coefficient (r) ranges from -1 to +1, where:
-
+1 indicates a perfect positive correlation (as one variable increases, the other increases).
-
-1 indicates a perfect negative correlation (as one variable increases, the other decreases).
-
0 indicates no linear relationship.
- Example: A researcher investigates the relationship between hours of sleep and academic performance. They collect data on both variables from a sample of students and calculate the correlation coefficient. A positive correlation would suggest that students who sleep more tend to perform better academically.
4. Regression Analysis: Predicting One Variable from Another
Regression analysis extends correlation by allowing researchers to predict the value of one variable (dependent variable) based on the value of one or more other variables (independent variables).
-
Simple Linear Regression: Predicts a dependent variable from a single independent variable.
- Example: Building on the sleep and academic performance example, a researcher might use simple linear regression to predict a student's GPA based on their average hours of sleep per night.
-
Multiple Linear Regression: Predicts a dependent variable from two or more independent variables.
- Example: A researcher might predict job satisfaction (dependent variable) using factors like salary, work-life balance, and supervisor support (independent variables).
5. Chi-Square Test: Analyzing Frequencies
The Chi-square test is used to analyze categorical data and determine if there's a significant association between two or more categorical variables.
* **Example:** A psychologist might investigate whether there's a relationship between gender and preferred coping mechanisms (problem-focused vs. emotion-focused). They would use a Chi-square test to analyze the frequencies of each coping mechanism across genders.
6. Non-parametric Tests: Handling Non-normal Data
When the assumptions of parametric tests (like t-tests and ANOVA) are violated (e.g., the data isn't normally distributed), non-parametric tests are used. These tests are less powerful but more robust to violations of assumptions.
-
Mann-Whitney U test: Non-parametric equivalent of the independent samples t-test.
-
Wilcoxon signed-rank test: Non-parametric equivalent of the paired samples t-test.
-
Kruskal-Wallis test: Non-parametric equivalent of the one-way ANOVA.
Choosing the Appropriate Inferential Test
Selecting the correct inferential statistical test is crucial for valid research. The choice depends on several factors:
- Type of data: Continuous (interval or ratio) or categorical (nominal or ordinal).
- Number of groups being compared: Two or more.
- Relationship between groups: Independent or related.
- Assumptions of the test: Normality, homogeneity of variance, etc.
Interpreting Results and Reporting Findings
Once a statistical test is conducted, the results must be interpreted correctly and reported clearly. Key elements of reporting include:
- The statistical test used.
- The degrees of freedom (df).
- The test statistic (e.g., t, F, χ²).
- The p-value.
- The effect size. Effect size measures the magnitude of the observed effect, providing a more complete picture than just the p-value.
Beyond Basic Tests: Advanced Inferential Techniques
The examples above represent fundamental inferential statistical tests. Psychology research also employs more sophisticated techniques, including:
-
Factor analysis: Used to identify underlying latent variables that explain correlations among observed variables.
-
Structural equation modeling (SEM): Tests complex relationships between multiple variables, including latent variables.
-
Multilevel modeling: Analyzes data with hierarchical structures (e.g., students nested within classrooms, nested within schools).
-
Survival analysis: Analyzes time-to-event data (e.g., time until relapse in a clinical trial).
Conclusion
Inferential statistics are indispensable tools in psychological research, enabling researchers to draw conclusions about populations based on sample data. By correctly applying appropriate statistical tests and interpreting results, psychologists can gain valuable insights into human behavior, mental processes, and the effectiveness of interventions. Understanding the principles of hypothesis testing, the various inferential tests, and their limitations is essential for conducting rigorous and meaningful psychological research. The continuous evolution of statistical methods ensures psychologists can tackle increasingly complex research questions and contribute to a deeper understanding of the human mind.
Latest Posts
Latest Posts
-
Antarctica Governing The Icy Continent Essay
May 25, 2025
-
The American Embassy Chimamanda Ngozi Adichie
May 25, 2025
-
Summary Of Stave 2 A Christmas Carol
May 25, 2025
-
12th Night Act 1 Scene 5
May 25, 2025
-
Test Bank Lewis Medical Surgical 12th Edition
May 25, 2025
Related Post
Thank you for visiting our website which covers about Example Of Inferential Statistics In Psychology . We hope the information provided has been useful to you. Feel free to contact us if you have any questions or need further assistance. See you next time and don't miss to bookmark.