Ed Is Collecting Data On A Students Latency
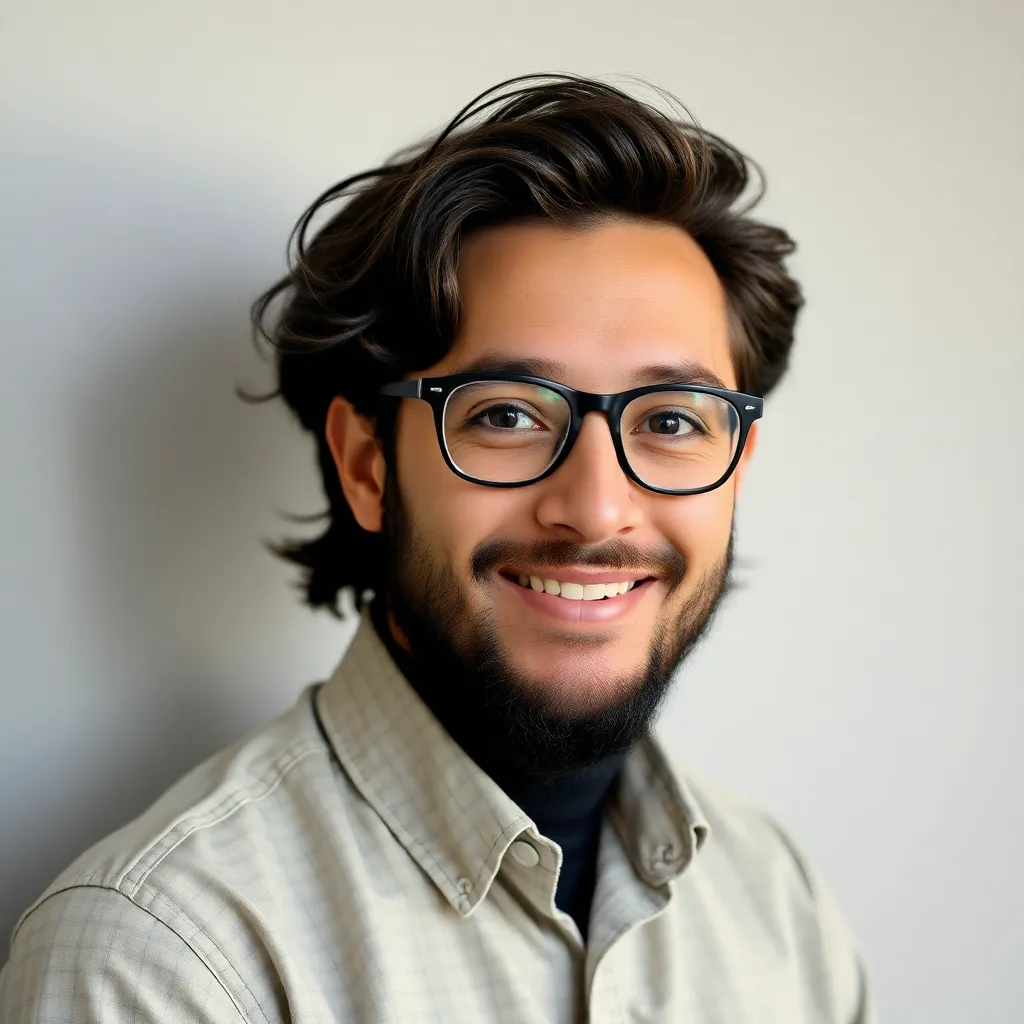
Juapaving
May 25, 2025 · 6 min read
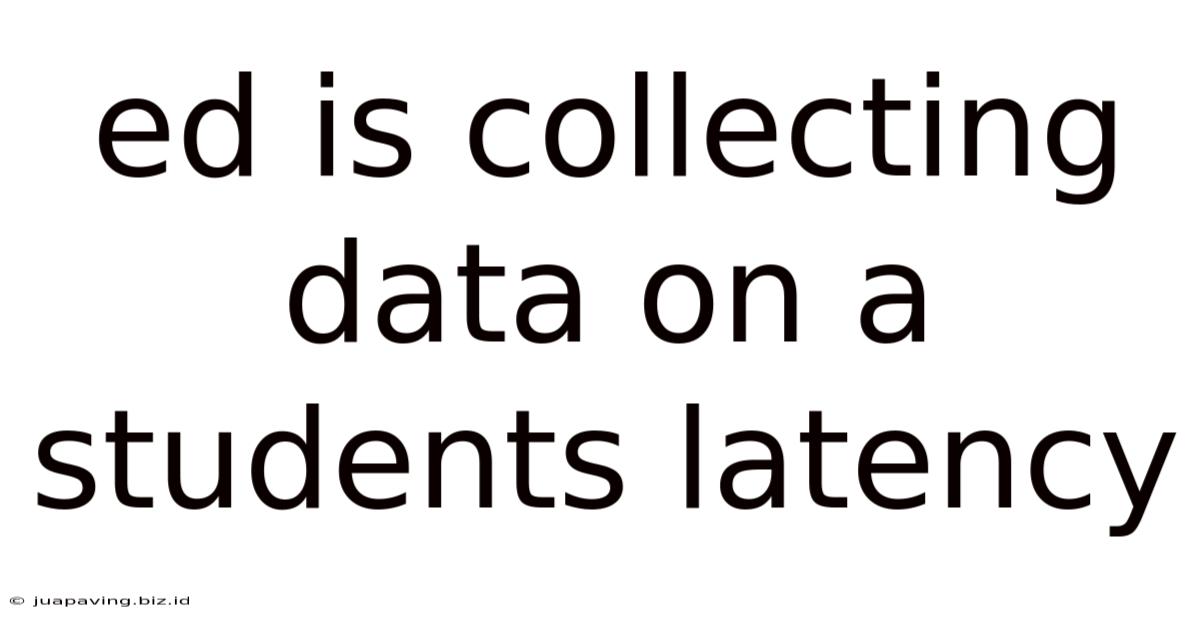
Table of Contents
Ed Is Collecting Data on Student Latency: Understanding the Implications and Best Practices
The digital age has transformed education, with online learning platforms becoming increasingly prevalent. This shift has brought many benefits, but it also presents new challenges. One key area requiring attention is student latency, the delay between a student's action and the platform's response. Ed, a hypothetical online learning platform, is collecting data on student latency to improve its services and enhance the learning experience. This article delves into the significance of collecting this data, the potential implications, and best practices for handling it responsibly and effectively.
Why Ed is Collecting Data on Student Latency
Understanding student latency is crucial for several reasons:
1. Enhancing User Experience:
High latency directly impacts the user experience. Slow loading times, laggy interactions, and delayed feedback can frustrate students, leading to decreased engagement and potentially impacting learning outcomes. By analyzing latency data, Ed can identify bottlenecks and optimize its platform for smoother, more responsive performance. This translates to a more enjoyable and efficient learning environment.
2. Identifying Technical Issues:
Latency data can pinpoint technical problems within the platform. Unexpected spikes in latency might indicate server issues, network congestion, or problems with specific features. By identifying these issues quickly, Ed can take proactive steps to resolve them, minimizing disruptions to student learning. This proactive approach improves the platform's reliability and overall stability.
3. Optimizing Platform Performance:
Collecting and analyzing latency data allows Ed to continuously improve the platform's performance. This might involve upgrading servers, optimizing code, or improving network infrastructure. By focusing on areas that contribute to high latency, Ed can deliver a faster and more efficient learning experience for all its users. The iterative nature of this data-driven approach leads to continuous enhancement.
4. Personalizing the Learning Experience:
Latency data, when combined with other data points, can contribute to personalized learning experiences. For instance, if a particular student consistently experiences high latency, Ed might provide alternative learning materials or adjust the delivery method to accommodate their individual circumstances. This individualized approach addresses unique challenges and fosters inclusive learning.
5. Improving Content Delivery:
Latency data can help optimize the delivery of learning content. By analyzing which types of content (videos, images, interactive exercises) contribute most to latency, Ed can make informed decisions about content optimization and delivery methods. This can involve compressing files, using optimized image formats, or employing content delivery networks (CDNs). Effective content delivery is vital for a seamless learning journey.
Potential Implications of Collecting Student Latency Data
While collecting latency data offers numerous benefits, it's crucial to consider the potential implications:
1. Privacy Concerns:
Collecting any user data raises privacy concerns. Ed must ensure it complies with all relevant data protection regulations (like GDPR or CCPA) and maintains the confidentiality and security of student data. Transparent data usage policies are essential to build trust and maintain user confidence. Data anonymization and aggregation techniques are crucial to protect individual student identities.
2. Data Security:
The collected latency data must be securely stored and protected against unauthorized access or breaches. Implementing robust security measures, including encryption and access controls, is essential. Regular security audits and penetration testing can help identify and address vulnerabilities proactively. Data security should be a top priority to prevent potential harm and maintain user trust.
3. Bias and Fairness:
Latency can be influenced by factors beyond the platform's control, such as a student's internet connection or device capabilities. Ed must be mindful of potential biases and ensure its analysis doesn't unfairly penalize students based on their technological resources. Fairness and equity must be central considerations in data analysis and interpretation.
4. Over-reliance on Data:
While data-driven insights are valuable, Ed should avoid over-reliance on latency data alone. It's important to consider other factors, such as student engagement, learning outcomes, and feedback, to gain a holistic understanding of the learning experience. A balanced approach that combines data analysis with qualitative feedback is crucial for comprehensive insights.
5. Ethical Considerations:
Ed must consider the ethical implications of collecting and using student latency data. Transparency about data collection practices, informed consent, and the responsible use of data are paramount. Ethical considerations should guide all aspects of data collection, analysis, and interpretation.
Best Practices for Handling Student Latency Data
To effectively and responsibly utilize student latency data, Ed should adopt these best practices:
1. Data Minimization:
Only collect the minimum necessary latency data. Avoid collecting unnecessary information that could compromise privacy or security. Focus on collecting data that directly contributes to improving platform performance and the learning experience.
2. Transparency and Consent:
Be transparent with students about the data collection practices. Obtain informed consent before collecting and using any student data. Clearly explain how the data will be used and what measures are in place to protect privacy and security. Transparency builds trust and fosters a positive relationship with users.
3. Data Anonymization and Aggregation:
Anonymize and aggregate the latency data whenever possible to protect student identities. This involves removing personally identifiable information and combining data from multiple students to create general trends and patterns. Anonymization is a crucial step in protecting student privacy.
4. Robust Security Measures:
Implement robust security measures to protect the collected data from unauthorized access, breaches, or loss. This includes encryption, access controls, regular security audits, and incident response plans. Security should be a priority to ensure data integrity and prevent misuse.
5. Regular Monitoring and Analysis:
Regularly monitor and analyze the collected latency data to identify trends, patterns, and potential issues. Use data visualization techniques to make the data easier to understand and interpret. Continuous monitoring allows for proactive problem-solving and ongoing platform optimization.
6. Continuous Improvement:
Use the insights from the data analysis to continuously improve the platform's performance and enhance the learning experience. This iterative process involves testing different solutions, monitoring their impact, and adjusting accordingly. Continuous improvement is essential for delivering a high-quality learning experience.
7. Collaboration and Feedback:
Collaborate with educators and students to gather feedback on the platform's performance and identify areas for improvement. Incorporate this feedback into the data analysis and decision-making process. Collaboration fosters a user-centric approach to platform development.
8. Compliance with Regulations:
Ensure compliance with all relevant data protection regulations and privacy laws. This might include GDPR, CCPA, or other regional regulations. Compliance demonstrates responsibility and builds trust.
9. Data Retention Policies:
Establish clear data retention policies and procedures. Only retain the data for as long as necessary for its intended purpose. Securely delete the data once it is no longer needed. Data retention policies are crucial for responsible data management.
10. Regular Audits and Reviews:
Conduct regular audits and reviews of the data collection and handling practices to ensure compliance with regulations, best practices, and ethical considerations. Regular audits contribute to continuous improvement and risk mitigation.
Conclusion: Data-Driven Improvement for Ed
Ed's initiative to collect data on student latency represents a proactive approach to improving the online learning experience. By carefully considering the potential implications and adhering to best practices, Ed can harness the power of data to enhance platform performance, personalize learning, and ensure a positive and effective learning environment for all its students. This data-driven approach will not only benefit the platform but also contribute to a more equitable and efficient future for online education. The ethical and responsible handling of this data is paramount to maintaining trust and fostering a positive learning experience. By prioritizing privacy, security, and transparency, Ed can pave the way for a more effective and enjoyable online learning journey for its students.
Latest Posts
Latest Posts
-
Amigos De Todas Partes Vhl Answers
May 25, 2025
-
A Christmas Carol Stave 5 Summary
May 25, 2025
-
Closed Innovation Is Associated With Which Organizational Inertia Problem
May 25, 2025
-
Which Of The Following Is A Valid Offer
May 25, 2025
-
What Point Of View Is A Rose For Emily
May 25, 2025
Related Post
Thank you for visiting our website which covers about Ed Is Collecting Data On A Students Latency . We hope the information provided has been useful to you. Feel free to contact us if you have any questions or need further assistance. See you next time and don't miss to bookmark.