Data Combines With Context To Become Which Of The Following
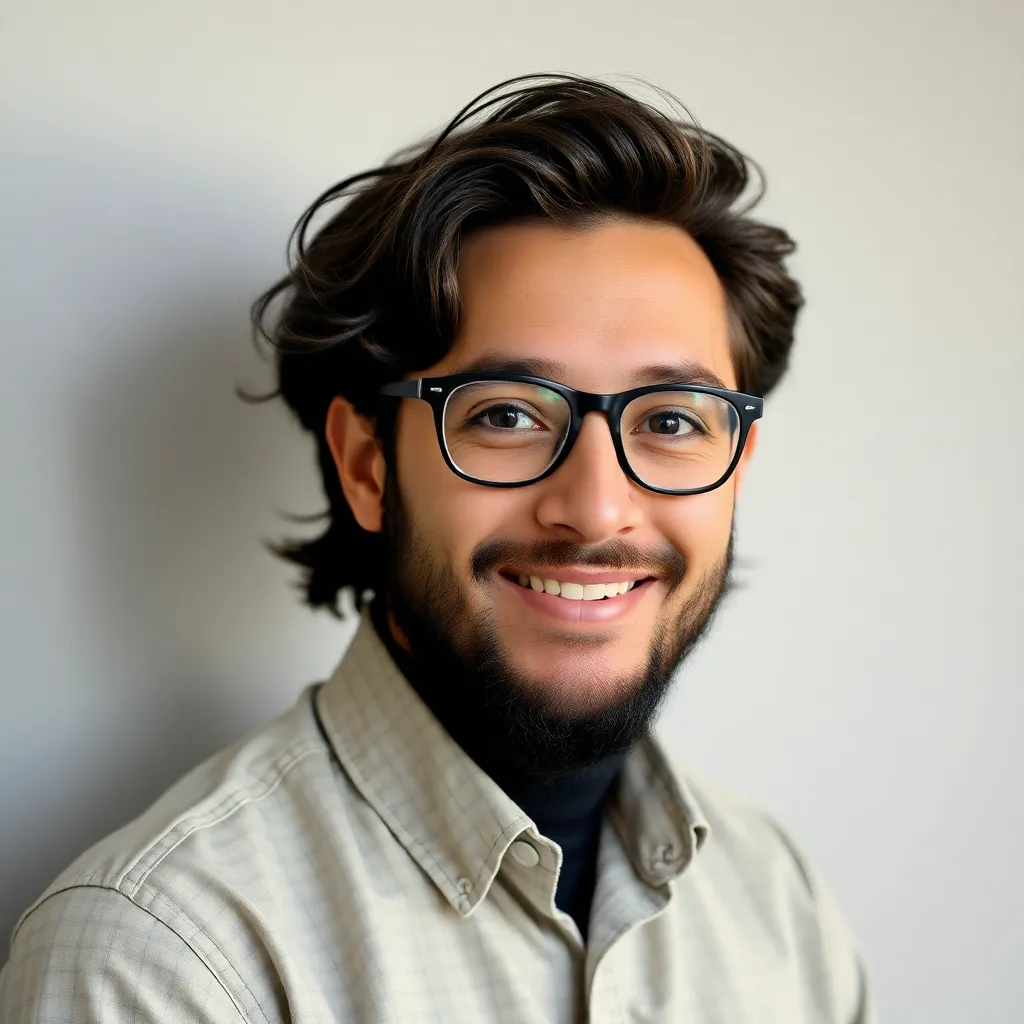
Juapaving
May 24, 2025 · 6 min read
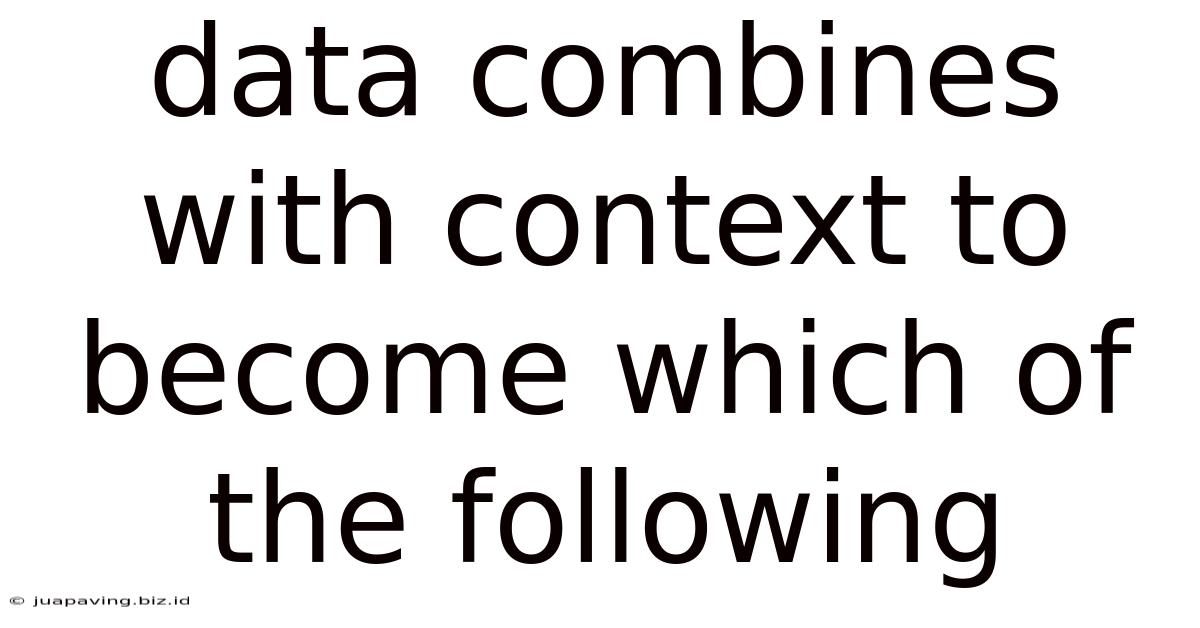
Table of Contents
Data Combined with Context Becomes Information: Unveiling the Power of Meaningful Insights
Data, in its raw form, is simply a collection of facts and figures. It's the unorganized, uninterpreted stuff that sits in databases and spreadsheets. Think numbers, names, dates, and events – all devoid of inherent meaning. However, when we add context to this raw data, it transforms into something significantly more valuable: information. This transformation is crucial for understanding the world around us, making informed decisions, and gaining a competitive edge in today's data-driven environment.
Understanding the Data-to-Information Transformation
The journey from data to information is a fundamental concept in data science, business intelligence, and knowledge management. It's not a simple switch, but rather a process of enriching raw data points with meaningful details that give them relevance and significance. This context can come from various sources, including:
1. Time and Place: The Pillars of Context
Consider a single data point: "Sales = $10,000". By itself, it's meaningless. However, adding context such as time (e.g., "Sales in Q3 2024 = $10,000") and place (e.g., "Sales in the London branch in Q3 2024 = $10,000") immediately transforms this figure into useful information. We now understand the sales performance within a specific timeframe and geographical location. This contextualization allows for comparisons, trend analysis, and informed decision-making.
2. Relationships and Associations: Unveiling Connections
Contextualization often involves understanding the relationships between different data points. Imagine a dataset containing customer purchase history. A single purchase might not tell much. But, by connecting this purchase to other data points like customer demographics, website browsing history, and previous interactions with customer service, we gain a much richer understanding of the customer's behavior, preferences, and potential future needs. This allows businesses to personalize marketing campaigns, improve customer service, and develop more effective products.
3. Background Knowledge and Domain Expertise: The Human Element
Context isn't always explicitly present in the data itself. Often, it requires human interpretation and domain expertise. For instance, analyzing medical data requires a deep understanding of physiology and disease processes. Similarly, interpreting financial data necessitates a grasp of accounting principles and economic trends. The human element brings crucial background knowledge that transforms raw data into meaningful insights. This expertise adds layers of understanding that algorithms alone might miss.
4. Comparative Data and Benchmarks: Establishing Contextual Norms
Comparing data to benchmarks or similar datasets establishes a critical contextual frame. Consider the sales data mentioned earlier. Comparing the $10,000 sales figure to previous quarters' sales, sales of other branches, or industry averages paints a much clearer picture of performance. Is this sales figure good, bad, or average? The answer depends entirely on the comparative context.
The Crucial Role of Context in Different Fields
The importance of contextualizing data varies across numerous fields. Here are some notable examples:
1. Business Intelligence and Analytics: Driving Strategic Decisions
In the business world, context is paramount. Analyzing website traffic data without knowing the marketing campaigns running concurrently provides an incomplete picture. Similarly, understanding customer churn requires considering factors such as product quality, customer service interactions, and competitive offerings. Contextualized data powers informed decision-making, leading to improved strategies and business outcomes.
Key Applications:
- Marketing Analytics: Understanding campaign performance requires contextualizing website traffic data with advertising spend, social media engagement, and conversion rates.
- Sales Analytics: Analyzing sales data needs context from market trends, competitor activity, and economic indicators.
- Customer Relationship Management (CRM): Understanding customer behavior necessitates contextualizing purchase history with demographics, preferences, and interactions.
2. Healthcare: Improving Patient Outcomes
In healthcare, context is a matter of life and death. A patient's blood pressure reading is only meaningful when considered alongside other vital signs, medical history, lifestyle factors, and current medications. Contextualizing medical data enables accurate diagnoses, personalized treatment plans, and improved patient outcomes.
Key Applications:
- Disease diagnosis: Contextualizing symptoms with medical imaging, lab results, and patient history.
- Treatment optimization: Tailoring medication dosages and therapies based on patient-specific factors.
- Public health surveillance: Analyzing disease outbreaks requires considering factors like location, population demographics, and environmental conditions.
3. Scientific Research: Validating Hypotheses and Discovering Patterns
Scientific research heavily relies on data analysis. However, the raw data alone doesn't tell the entire story. Scientists must contextualize their findings with existing literature, theoretical frameworks, and experimental design. Context is critical in validating hypotheses, identifying causal relationships, and drawing meaningful conclusions.
Key Applications:
- Clinical trials: Contextualizing results with patient demographics, treatment protocols, and blinding techniques.
- Environmental studies: Contextualizing pollution levels with geographical location, weather patterns, and industrial activity.
- Genomic research: Contextualizing gene expression data with patient phenotypes, environmental factors, and genetic variations.
4. Law Enforcement and Security: Enhancing Public Safety
In law enforcement, contextualizing data is crucial for crime prevention and solving investigations. Connecting crime scene evidence with witness testimonies, suspect information, and geographical location helps investigators build a comprehensive understanding of the events. Similarly, contextualizing security data allows for proactive threat detection and prevention.
Key Applications:
- Crime scene investigation: Contextualizing evidence with witness accounts, suspect profiles, and crime patterns.
- Cybersecurity: Contextualizing network traffic with user behavior, geographic location, and system logs to detect malicious activities.
- Fraud detection: Contextualizing financial transactions with customer profiles and historical spending patterns to identify fraudulent activities.
The Challenges of Contextualizing Data
While the benefits are clear, contextualizing data presents significant challenges:
- Data Silos: Data is often scattered across different systems and departments, making integration and contextualization difficult.
- Data Quality: Inaccurate or incomplete data can lead to flawed interpretations.
- Data Security and Privacy: Contextualizing data requires careful consideration of privacy regulations and security protocols.
- Computational Complexity: Analyzing large datasets and establishing relationships between different data points can be computationally intensive.
- Human Bias: Subjective interpretations can introduce bias into the contextualization process.
Overcoming the Challenges: Strategies for Effective Contextualization
Addressing these challenges requires a multi-faceted approach:
- Data Integration: Implementing robust data integration strategies to bring data from different sources together.
- Data Cleaning and Validation: Employing rigorous data cleaning and validation techniques to ensure data accuracy.
- Data Governance: Establishing clear data governance policies to manage data quality, security, and privacy.
- Advanced Analytics Techniques: Utilizing advanced analytics techniques such as machine learning and natural language processing to automate contextualization.
- Human-in-the-Loop Systems: Combining automated methods with human expertise to ensure accurate and unbiased interpretations.
Conclusion: Data + Context = Information – The Foundation of Knowledge
In conclusion, the combination of data and context is essential for transforming raw facts into meaningful information. This process is crucial across various fields, driving informed decision-making, innovation, and improved outcomes. While challenges exist in achieving effective contextualization, the rewards of harnessing the power of meaningful insights are undeniable. By overcoming the hurdles and adopting a strategic approach to data integration, analysis, and interpretation, we can unlock the full potential of data and create a future powered by knowledge. The journey from data to information is not just about technology; it's about understanding the world through a lens of meaningful context.
Latest Posts
Latest Posts
-
How Are Jalousie Windows Normally Opened And Closed
May 24, 2025
-
Match Each Term To Its Description
May 24, 2025
-
Analysis The Gift Of The Magi
May 24, 2025
-
Narrative Of The Life Of Frederick Douglass Chapter 10 Summary
May 24, 2025
-
Which Of The Following Best Describes A Front End Developer
May 24, 2025
Related Post
Thank you for visiting our website which covers about Data Combines With Context To Become Which Of The Following . We hope the information provided has been useful to you. Feel free to contact us if you have any questions or need further assistance. See you next time and don't miss to bookmark.