Data Are Plotted On Line Graphs According To
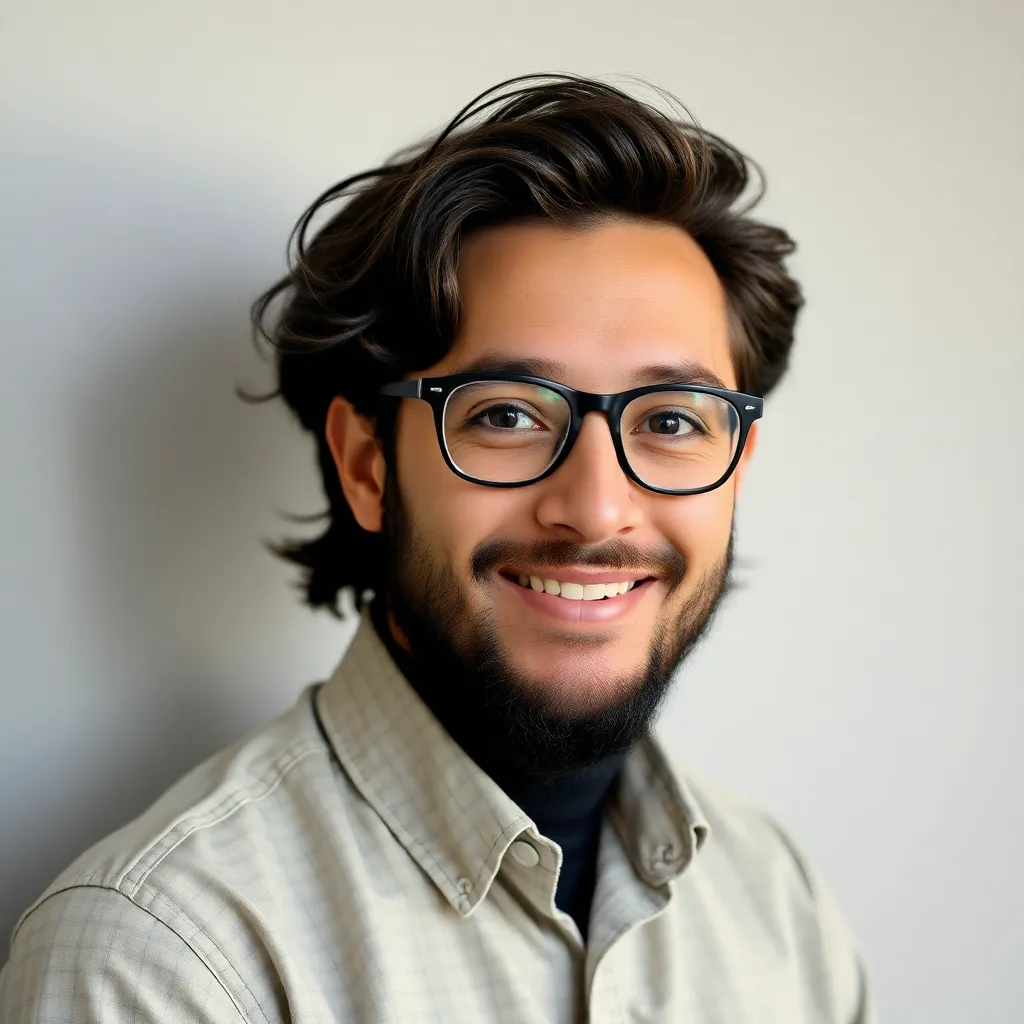
Juapaving
May 25, 2025 · 6 min read
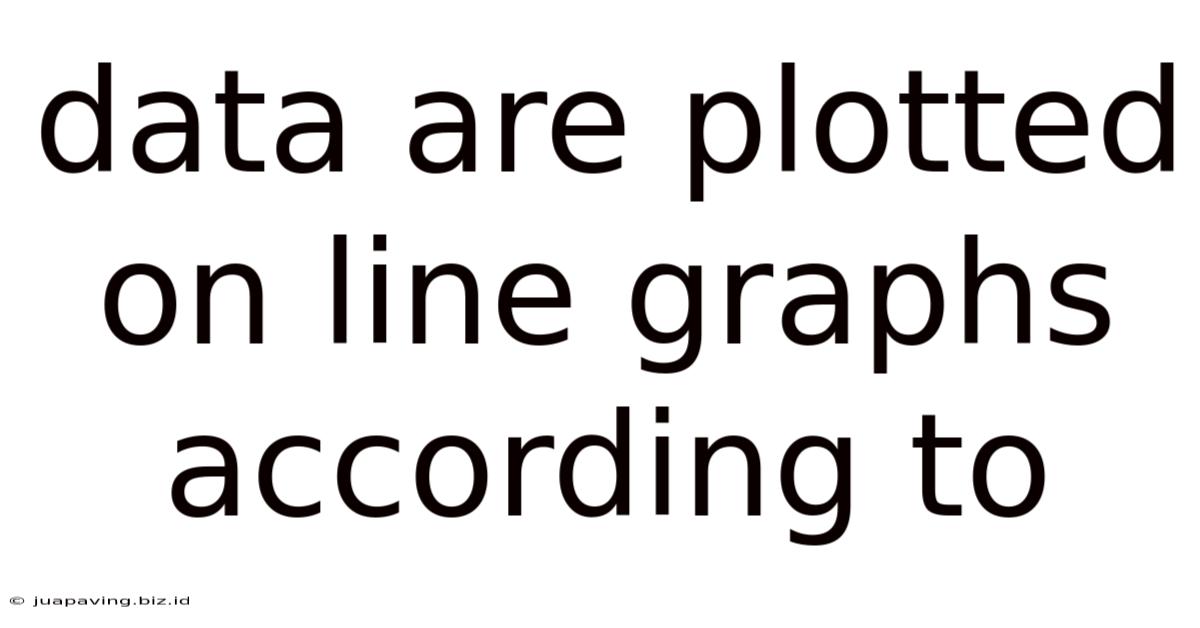
Table of Contents
Data are Plotted on Line Graphs According To: A Comprehensive Guide
Line graphs are powerful visual tools used to display data that changes over time or across a continuous scale. Understanding how data is plotted on a line graph is crucial for effective data analysis and communication. This comprehensive guide will explore the principles behind line graph construction, delve into different types of line graphs, and discuss best practices for creating clear and informative visualizations.
Understanding the Components of a Line Graph
Before diving into the specifics of data plotting, let's review the fundamental components of a line graph:
1. The X-axis (Horizontal Axis): The Independent Variable
The x-axis typically represents the independent variable. This is the variable that is manipulated or measured, and its values are plotted along the horizontal line. Common examples of independent variables include:
- Time: Days, weeks, months, years, etc. Time series data is frequently displayed using line graphs.
- Distance: Meters, kilometers, miles, etc.
- Temperature: Degrees Celsius, Fahrenheit, Kelvin, etc.
- Concentration: Molarity, percentage, etc.
2. The Y-axis (Vertical Axis): The Dependent Variable
The y-axis represents the dependent variable. This is the variable that is being measured or observed, and its values are plotted along the vertical line. The dependent variable's value depends on the independent variable. Examples of dependent variables include:
- Sales Revenue: The revenue generated over time.
- Population Growth: The change in population over time.
- Plant Height: The height of a plant over time.
- Reaction Rate: The speed of a chemical reaction at different temperatures.
3. Data Points: The Intersection of Variables
Data points are represented by dots or other symbols placed at the intersection of the x and y values for each data point. These points represent individual observations or measurements.
4. Line Segments: Connecting the Dots
Line segments connect the data points, illustrating the trend or relationship between the independent and dependent variables. The slope of the line indicates the rate of change. A steep slope indicates a rapid change, while a shallow slope indicates a gradual change.
5. Labels and Titles: Providing Context
Clear and concise labels are essential for understanding the graph. Labels should identify the variables represented on each axis (including units), and a title should clearly describe the data presented. A legend may also be necessary if multiple lines are plotted on the same graph.
Types of Line Graphs and Data Plotting Methods
Several types of line graphs exist, each suited for different data types and purposes:
1. Simple Line Graph: One Variable Over Time
The simplest line graph shows a single dependent variable plotted against an independent variable, usually time. This is ideal for tracking changes over a period. For example, tracking the daily sales of a product over a month. The data is plotted by finding the intersection of the x-axis value (date) and the y-axis value (sales) and marking a point. These points are then connected by a line.
2. Multiple Line Graph: Comparing Multiple Variables
Multiple line graphs allow the comparison of multiple dependent variables against the same independent variable. This type of graph is excellent for comparing trends across different categories or groups. For example, comparing sales of different product lines over a year. Each data series (product line) gets its own line, typically distinguished by different colors or line styles, and a legend explains which line represents which data series.
3. Grouped Line Graph: Showing Subcategories
Grouped line graphs are similar to multiple line graphs, but they group data points for different subcategories within a larger category. This is useful when more granularity is needed. For instance, comparing sales of different product lines within each region.
4. Stacked Line Graph: Showing Component Parts
Stacked line graphs show how different components contribute to a total value over time. Each line represents a component, and the lines are stacked on top of each other to show the cumulative total. This is useful for visualizing proportions of different parts of a whole. For example, displaying the sales contribution of various products to overall revenue.
Best Practices for Creating Effective Line Graphs
Creating effective line graphs requires attention to detail. Here are some key best practices:
- Choose the Right Graph Type: Select the type of line graph that best suits your data and the message you want to convey.
- Clear and Concise Labels: Use clear and concise labels for both axes and provide a descriptive title.
- Consistent Scaling: Maintain consistent scaling on both axes to avoid misinterpretations.
- Appropriate Line Style and Colors: Use visually distinct line styles and colors to differentiate multiple lines in a multiple line graph.
- Data Point Markers (Optional): While not always necessary, data point markers can improve readability, especially for dense datasets.
- Minimize Clutter: Avoid unnecessary details that could distract the reader from the main message.
- Data Accuracy: Ensure that the data is accurate and appropriately represented.
- Contextual Information: Provide sufficient contextual information to help the audience understand the data's significance.
Advanced Considerations in Line Graph Plotting
Beyond the basic principles, there are more advanced considerations to create sophisticated and informative line graphs:
- Interpolation vs. Extrapolation: Interpolation involves estimating values within the range of the existing data, while extrapolation involves estimating values outside the range. Extrapolation should be done cautiously, as it can lead to inaccurate conclusions.
- Smoothing Techniques: Smoothing techniques can help to highlight trends in noisy data by reducing the visual impact of minor fluctuations. However, smoothing can also mask important details, so it should be used judiciously.
- Statistical Analysis: Incorporating statistical measures, such as confidence intervals or trend lines, can enhance the insights derived from the line graph.
- Choosing the Right Software: Various software packages, such as spreadsheet programs (e.g., Microsoft Excel, Google Sheets), statistical software (e.g., R, SPSS), and data visualization tools (e.g., Tableau, Power BI), offer different capabilities for creating line graphs.
Examples of Data Plotted on Line Graphs
Let's consider a few real-world examples illustrating how data is plotted on line graphs:
Example 1: Stock Prices Over Time
The x-axis represents the date (independent variable), and the y-axis represents the closing price of a stock (dependent variable). Each data point represents the closing price on a particular date. The line connecting these points shows the trend of the stock price over time.
Example 2: Website Traffic Over Months
The x-axis represents the month (independent variable), and the y-axis represents the number of website visitors (dependent variable). Each data point shows the number of visitors in a particular month. The line demonstrates the website traffic trend over the months.
Example 3: Temperature Changes Throughout the Day
The x-axis represents the time of day (independent variable), and the y-axis represents the temperature (dependent variable). Each point indicates the temperature at a specific time. The line illustrates how temperature changes throughout a day.
Example 4: Growth of a Plant Over Weeks
The x-axis represents the week (independent variable), and the y-axis represents the height of a plant (dependent variable). Each data point reflects the plant's height at the end of each week. The line reveals the plant's growth pattern over the weeks.
Conclusion
Line graphs are versatile tools for visualizing data changes over time or across a continuous scale. By understanding the principles of data plotting, choosing the appropriate graph type, and following best practices, you can create clear, informative, and compelling visualizations that effectively communicate data insights. Remember to always consider your audience and choose the graph type and presentation that best suits their needs and understanding. Effective line graph creation is a crucial skill for data analysis and communication in diverse fields.
Latest Posts
Latest Posts
-
Antarctica Governing The Icy Continent Essay
May 25, 2025
-
The American Embassy Chimamanda Ngozi Adichie
May 25, 2025
-
Summary Of Stave 2 A Christmas Carol
May 25, 2025
-
12th Night Act 1 Scene 5
May 25, 2025
-
Test Bank Lewis Medical Surgical 12th Edition
May 25, 2025
Related Post
Thank you for visiting our website which covers about Data Are Plotted On Line Graphs According To . We hope the information provided has been useful to you. Feel free to contact us if you have any questions or need further assistance. See you next time and don't miss to bookmark.