Binomial Distribution Examples In Real Life
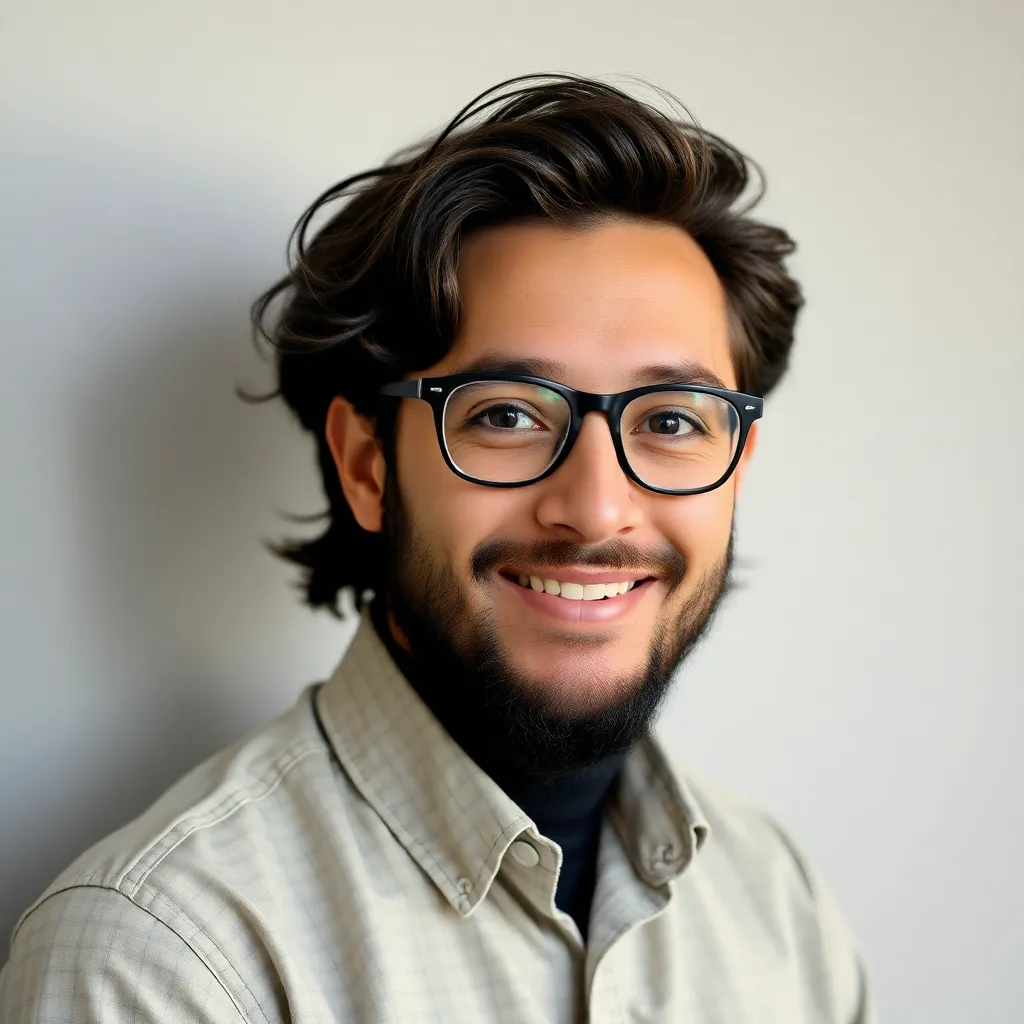
Juapaving
May 13, 2025 · 6 min read
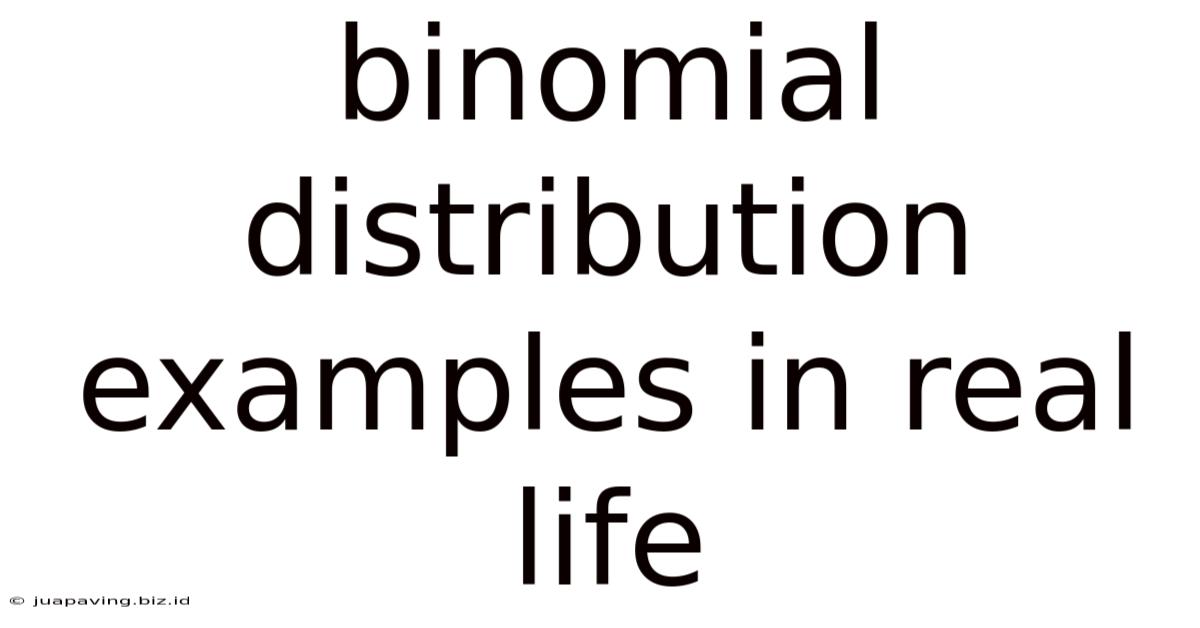
Table of Contents
Binomial Distribution Examples in Real Life: From Coin Flips to Medical Trials
The binomial distribution, a cornerstone of probability and statistics, describes the probability of getting exactly k successes in n independent Bernoulli trials. While the classic example involves flipping a coin, its real-world applications extend far beyond simple games of chance. Understanding this distribution allows us to model and predict outcomes in diverse fields, from manufacturing quality control to medical research. This article delves into numerous real-life examples, illustrating the power and versatility of the binomial distribution.
What is a Binomial Distribution?
Before diving into examples, let's briefly recap the key characteristics of a binomial distribution:
- Fixed Number of Trials (n): The experiment consists of a fixed number of independent trials.
- Independent Trials: The outcome of one trial doesn't affect the outcome of any other trial.
- Two Possible Outcomes: Each trial results in one of two mutually exclusive outcomes: success or failure.
- Constant Probability of Success (p): The probability of success (p) remains constant across all trials. The probability of failure is therefore (1-p), often denoted as q.
The probability of getting exactly k successes in n trials is given by the binomial probability formula:
P(X = k) = (nCk) * p^k * (1-p)^(n-k)
where nCk is the binomial coefficient, calculated as n! / (k! * (n-k)!).
Real-Life Examples of Binomial Distribution
The applications of the binomial distribution are vast and varied. Let's explore some compelling examples across different domains:
1. Quality Control in Manufacturing
Imagine a factory producing light bulbs. Each bulb has a certain probability of being defective (p). A quality control inspector randomly selects a sample of n bulbs and tests them. The number of defective bulbs in the sample follows a binomial distribution. This allows the factory to estimate the overall defect rate and adjust its manufacturing processes accordingly. A high number of defective bulbs in the sample indicates a potential problem needing immediate attention. Keywords: quality control, manufacturing, defect rate, binomial probability
2. Medical Trials and Clinical Studies
Binomial distributions are frequently used in medical research to assess the effectiveness of new treatments. Consider a clinical trial testing a new drug. Each patient in the trial is considered a Bernoulli trial. A 'success' is defined as a positive response to the treatment, while 'failure' represents no response or adverse effects. The binomial distribution helps researchers determine the probability of observing a certain number of successful outcomes given a sample size and a hypothesized success rate. Keywords: clinical trials, medical research, drug effectiveness, success rate, treatment response
3. A/B Testing in Marketing and Web Development
A/B testing is a crucial tool for optimizing website design, marketing campaigns, and other online initiatives. In A/B testing, two versions (A and B) of a webpage, advertisement, or email are presented to different groups of users. The number of users who respond positively (e.g., click a button, make a purchase) follows a binomial distribution. By analyzing the results using statistical tests based on the binomial distribution, marketers can determine which version performs better. Keywords: A/B testing, conversion rate, click-through rate, online marketing, website optimization
4. Opinion Polls and Surveys
Opinion polls and surveys rely heavily on statistical sampling and probability distributions. When surveying a population to gauge public opinion on a particular issue (e.g., a political candidate or a social policy), each respondent represents a Bernoulli trial. A 'success' is defined as a positive response (e.g., supporting a candidate). The binomial distribution helps predict the margin of error and the confidence interval for the estimated proportion of the population holding the desired opinion. Keywords: opinion polls, surveys, public opinion, sampling, margin of error, confidence interval
5. Genetics and Mendelian Inheritance
In genetics, the inheritance of traits often follows Mendelian laws. Consider a simple case where a single gene determines a particular trait (e.g., flower color). If one allele is dominant and another is recessive, the offspring's genotype (and resulting phenotype) follows a binomial distribution. For example, if we cross two heterozygous parents (carrying one dominant and one recessive allele), the probability of offspring inheriting the recessive trait follows a binomial distribution. Keywords: Mendelian inheritance, genetics, allele, genotype, phenotype, probability of inheritance
6. Sports and Games of Chance
While coin flips are a classic example, the binomial distribution extends to many other sporting events and games of chance. Consider a basketball player's free-throw attempts. Assuming each attempt is independent and has a constant probability of success (p), the number of successful free throws in n attempts follows a binomial distribution. This allows us to calculate the likelihood of the player making a certain number of free throws in a game. Similar applications exist in baseball (batting average), golf (putts made), and other sports. Keywords: sports statistics, free throws, batting average, probability of success, games of chance
7. Risk Assessment and Insurance
Insurance companies use binomial distributions to model risks and calculate premiums. Consider a car insurance company. Each insured driver represents a Bernoulli trial. A 'success' means the driver does not have an accident during the policy period, while 'failure' represents an accident. By estimating the probability of accidents (p) for different driver profiles, the company can determine the appropriate premiums to cover expected losses. Similar applications exist in health insurance, life insurance, and other areas of risk assessment. Keywords: risk assessment, insurance, probability of accidents, premium calculation, risk management
8. Reliability Engineering
In reliability engineering, the binomial distribution helps assess the reliability of systems and components. Imagine a system composed of n independent components, each with a probability of failure (p). The number of failed components follows a binomial distribution. Engineers can use this to estimate the overall system reliability and identify critical components requiring more attention. Keywords: reliability engineering, system reliability, component failure, probability of failure, system design
Beyond the Basics: Applications and Extensions
The binomial distribution serves as a foundation for more complex statistical models and analyses. Its limitations, primarily the assumptions of independent trials and constant success probability, often necessitate the use of more sophisticated techniques for real-world scenarios. However, the fundamental principles remain applicable in many situations.
For instance, understanding the binomial distribution is crucial for interpreting the results of hypothesis testing, particularly in situations where we're testing proportions or rates. This ties back to many of the examples already discussed, such as A/B testing and clinical trials. Determining whether an observed difference in success rates is statistically significant often relies on a framework built upon the binomial distribution.
Furthermore, as the number of trials (n) becomes large and the probability of success (p) is not too close to 0 or 1, the binomial distribution can be approximated by the normal distribution. This approximation simplifies calculations significantly and allows for the use of powerful statistical tools based on the normal distribution.
Conclusion
The binomial distribution, despite its seemingly simple formulation, is a powerful and versatile tool with wide-ranging applications across numerous fields. From assessing the quality of manufactured goods to evaluating the effectiveness of medical treatments and analyzing marketing campaign data, the principles of binomial probability provide invaluable insights for decision-making in a variety of contexts. Understanding its fundamental properties and applications is essential for anyone working with probability, statistics, or data analysis in the real world. By grasping its core concepts, individuals can better understand and interpret data, leading to improved decision-making across many industries and disciplines.
Latest Posts
Latest Posts
-
Moment Of Force Questions And Answers Pdf
May 13, 2025
-
Which Biomes Have The Greatest Biodiversity
May 13, 2025
-
What Is 10 Percent Of 80000
May 13, 2025
-
Organelle That Packages And Delivers Proteins
May 13, 2025
-
The Process Of Attracting Qualified Job Applicants Is Called
May 13, 2025
Related Post
Thank you for visiting our website which covers about Binomial Distribution Examples In Real Life . We hope the information provided has been useful to you. Feel free to contact us if you have any questions or need further assistance. See you next time and don't miss to bookmark.