Approximate The Measures Of Center For Following Gfdt
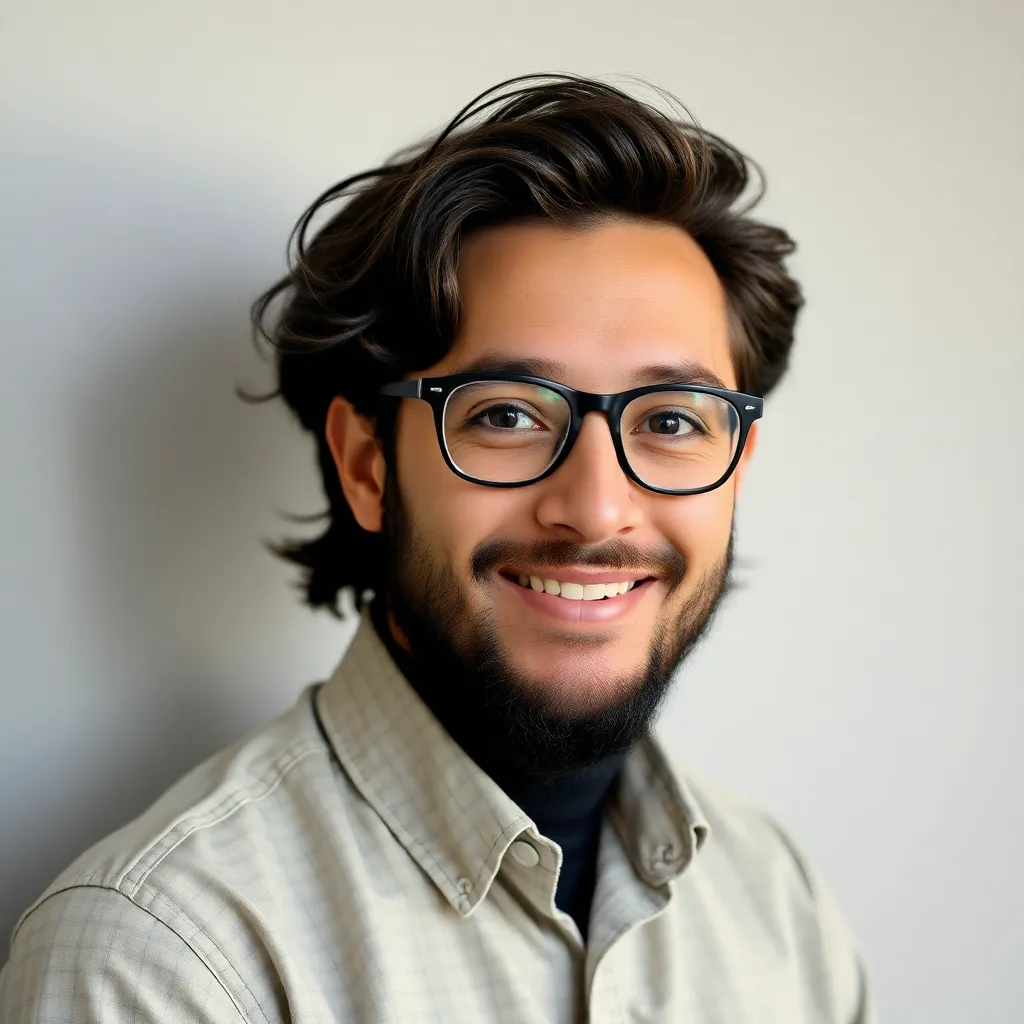
Juapaving
May 23, 2025 · 6 min read
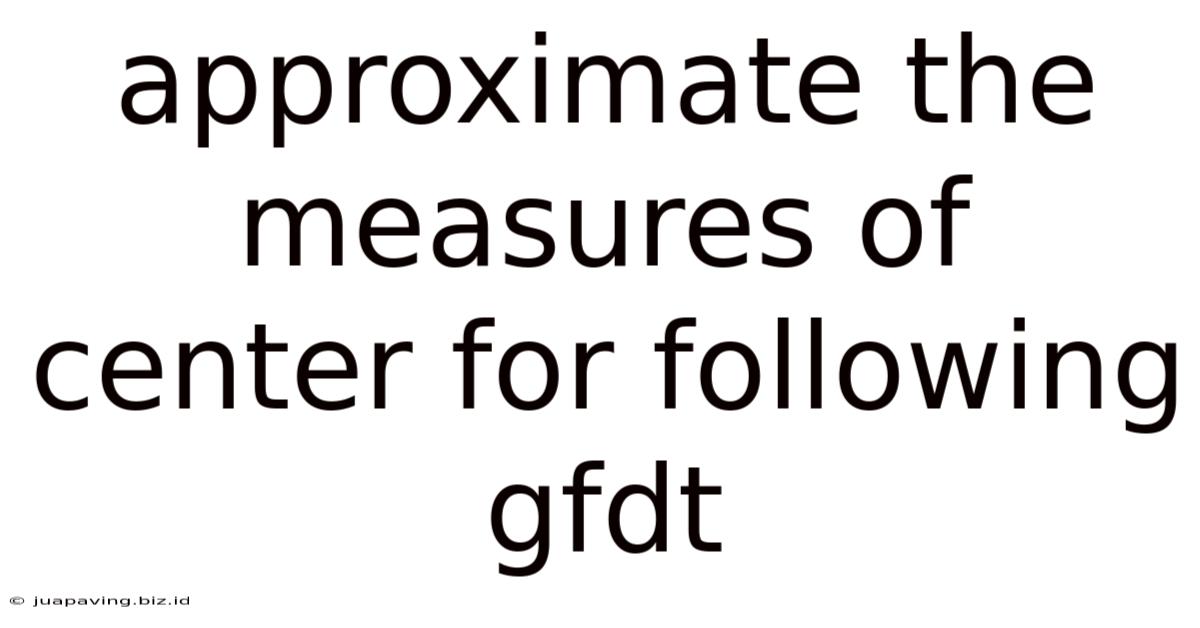
Table of Contents
Approximating Measures of Center for Grouped Frequency Distribution Tables (GFDTs)
Understanding the central tendency of a dataset is crucial in statistics. Measures of center, such as the mean, median, and mode, provide a single value that summarizes the typical or central value of a dataset. However, when dealing with large datasets, it's often more practical to organize the data into a grouped frequency distribution table (GFDT). This article delves into the methods used to approximate the measures of center from a GFDT, focusing on their applications and limitations.
What is a Grouped Frequency Distribution Table (GFDT)?
A GFDT is a way to summarize data by grouping values into classes or intervals and counting the frequency (number of occurrences) within each interval. This is particularly useful for large datasets where examining each individual data point is impractical. For instance, instead of listing hundreds of individual exam scores, we can group them into ranges (e.g., 90-100, 80-89, 70-79, etc.) and show the number of students who scored within each range.
Key Components of a GFDT:
- Class Intervals: Ranges of values that group the data. These intervals should be mutually exclusive (non-overlapping) and exhaustive (covering all data points).
- Class Frequency: The number of data points that fall within each class interval.
- Class Midpoint: The midpoint of each class interval, calculated as (lower limit + upper limit) / 2. This is crucial for approximating the mean.
- Cumulative Frequency: The running total of frequencies, indicating the number of data points up to a particular class interval. Useful for finding the median.
Approximating the Mean from a GFDT
The mean, or average, is calculated by summing all values and dividing by the total number of values. Since individual data points are not available in a GFDT, we approximate the mean using the class midpoints and their corresponding frequencies. The formula is:
Approximate Mean (x̄) = Σ(fᵢ * mᵢ) / Σfᵢ
Where:
- fᵢ = frequency of the i-th class interval
- mᵢ = midpoint of the i-th class interval
- Σfᵢ = total frequency (sum of all frequencies)
Example:
Let's say we have a GFDT of student ages:
Age Range | Frequency (fᵢ) | Midpoint (mᵢ) | fᵢ * mᵢ |
---|---|---|---|
18-20 | 5 | 19 | 95 |
21-23 | 10 | 22 | 220 |
24-26 | 15 | 25 | 375 |
27-29 | 8 | 28 | 224 |
Total | 40 | 914 |
Approximate Mean (x̄) = 914 / 40 = 22.85
Therefore, the approximate average age of the students is 22.85 years.
Limitations of Approximating the Mean:
The mean calculated from a GFDT is an approximation. The precision depends on the width of the class intervals. Narrower intervals lead to a more accurate approximation, while wider intervals introduce more error. Also, this method assumes that the data within each interval is evenly distributed, which may not always be true.
Approximating the Median from a GFDT
The median is the middle value when the data is ordered. In a GFDT, we approximate the median by identifying the class containing the median value and then using linear interpolation within that class.
Steps to Approximate the Median:
- Locate the Median Position: The median position is (n + 1) / 2, where 'n' is the total frequency.
- Identify the Median Class: Find the class interval whose cumulative frequency is greater than or equal to the median position.
- Linear Interpolation: Use the following formula to approximate the median:
Median ≈ L + [(n/2 - CF) / f] * w
Where:
- L = lower limit of the median class
- n = total frequency
- CF = cumulative frequency of the class before the median class
- f = frequency of the median class
- w = width of the median class
Example (using the same age data):
- Median Position = (40 + 1) / 2 = 20.5
- Median Class: The cumulative frequency of the 21-23 age range is 15 (5+10), and the cumulative frequency of the 24-26 age range is 25 (15+10). Thus, the median class is 24-26.
- Median ≈ 24 + [(20.5 - 15) / 15] * 3 = 24 + (5.5/15) * 3 ≈ 25.1
Therefore, the approximate median age is 25.1 years.
Limitations of Approximating the Median:
Similar to the mean, the median approximation is subject to error. The accuracy depends on the class interval width and the assumption of even data distribution within each interval. Linear interpolation is a simplification and might not perfectly capture the true median, especially if the data distribution is significantly skewed within the median class.
Approximating the Mode from a GFDT
The mode is the value that appears most frequently. In a GFDT, we approximate the mode by identifying the class with the highest frequency. This class is called the modal class. To get a more precise estimate, we can use the following formula:
Mode ≈ L + [(f₁ - f₀) / (2f₁ - f₀ - f₂)] * w
Where:
- L = lower limit of the modal class
- f₁ = frequency of the modal class
- f₀ = frequency of the class before the modal class
- f₂ = frequency of the class after the modal class
- w = width of the modal class
Example (using the same age data):
The modal class is 24-26 with a frequency of 15.
Mode ≈ 24 + [(15 - 10) / (2*15 - 10 - 8)] * 3 = 24 + (5/12) * 3 ≈ 25.25
Therefore, the approximate mode is 25.25 years.
Limitations of Approximating the Mode:
The approximated mode is highly dependent on the class interval width. If the class intervals are too wide, the mode might not accurately represent the most frequent value. Additionally, a GFDT might have more than one modal class (bimodal or multimodal) if multiple classes have the same highest frequency. The formula provided is only suitable for unimodal distributions. In cases of bimodal or multimodal distributions, it's better to simply report the modal classes.
Choosing the Appropriate Measure of Center
The choice of which measure of center (mean, median, or mode) to use depends on the nature of the data and the research question.
- Mean: Best suited for symmetrical distributions where extreme values don't significantly influence the result. Sensitive to outliers.
- Median: Less sensitive to outliers than the mean and is a better choice for skewed distributions. Provides a robust measure of the central tendency.
- Mode: Useful for identifying the most common value, especially for categorical or discrete data. Less informative for continuous data with many unique values.
Interpreting Approximations and Reporting Results
When working with GFDTs, it's crucial to remember that the measures of center are approximations. Always report the results as approximations and acknowledge the limitations inherent in using a GFDT. Clearly state the method used for approximation (e.g., linear interpolation for the median). Additionally, consider presenting the data visually using histograms or other graphical representations to gain further insights into the data distribution.
By understanding the methods for approximating measures of center from GFDTs and their limitations, researchers can effectively analyze and interpret large datasets, making informed conclusions based on the central tendency of the data. Remember to carefully consider the data's characteristics and choose the most appropriate measure of center for your specific research question. The combination of understanding the methodology, acknowledging the limitations, and using appropriate visualization techniques enhances the clarity and reliability of your statistical analysis.
Latest Posts
Latest Posts
-
Where Did Gatsby And Daisy First Meet
May 25, 2025
-
A Major Drawback Of Is That Winning The Debate
May 25, 2025
-
Characters In Diary Of Anne Frank
May 25, 2025
-
Miracles Of Jesus In The Book Of John
May 25, 2025
-
Perform Scalp Massage Movements Starting At
May 25, 2025
Related Post
Thank you for visiting our website which covers about Approximate The Measures Of Center For Following Gfdt . We hope the information provided has been useful to you. Feel free to contact us if you have any questions or need further assistance. See you next time and don't miss to bookmark.