A Histogram Is A Graphical Representation Of
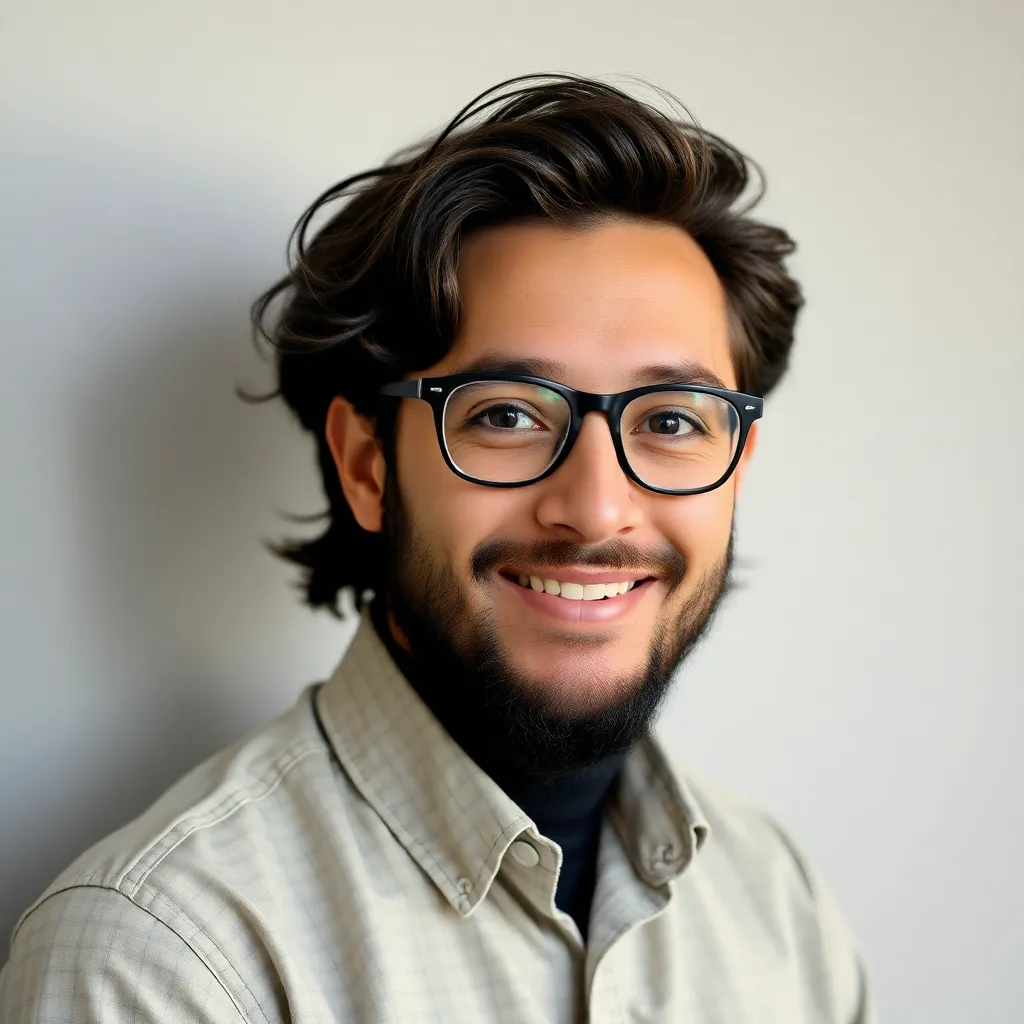
Juapaving
Apr 20, 2025 · 7 min read

Table of Contents
A Histogram is a Graphical Representation of Data Distribution
Histograms are powerful tools for visualizing data distributions. They provide a clear and concise way to understand the frequency of different values within a dataset. Unlike bar charts, which represent categorical data, histograms depict the distribution of numerical data, showing how often data points fall within specific ranges or bins. This article will delve deep into the intricacies of histograms, explaining their construction, interpretation, and various applications, all while keeping in mind SEO best practices.
Understanding the Fundamentals of Histograms
A histogram is essentially a graphical depiction of a frequency distribution. It displays data using bars of varying heights, where the height of each bar corresponds to the frequency of data points within a particular range or interval. This range is referred to as a "bin" or "class interval." The width of each bar usually remains consistent, representing equal intervals on the x-axis (horizontal). The y-axis (vertical) represents the frequency or count of data points falling within each bin.
Key Components of a Histogram:
- X-axis (Horizontal Axis): Represents the range of values of the variable being measured. This axis is divided into bins or intervals.
- Y-axis (Vertical Axis): Represents the frequency or count of data points that fall within each bin.
- Bins (Class Intervals): These are the ranges of values into which the data is divided. The choice of bin width is crucial and significantly impacts the histogram's appearance.
- Bars: Rectangular bars whose height corresponds to the frequency of data points within each bin. The bars are adjacent to each other, unlike in bar charts where gaps are present.
Constructing a Histogram: A Step-by-Step Guide
Creating a histogram involves several steps:
-
Data Collection: Begin by gathering the data you want to analyze. This could be anything from student test scores to the heights of trees in a forest.
-
Determine the Range: Find the minimum and maximum values in your dataset to determine the overall range of your data.
-
Choose the Number of Bins: This is a crucial step. Too few bins will result in a loss of detail, while too many bins might create a jagged and uninformative histogram. There's no single "correct" number of bins, but some common rules of thumb include Sturges' formula (k = 1 + 3.322 log10(n), where n is the number of data points) or the square root rule (k = √n). Experimentation often yields the most visually appealing and informative histogram.
-
Determine the Bin Width: Divide the range of your data by the chosen number of bins to find the width of each bin. It's usually best to use equal-width bins for easier interpretation.
-
Count Frequencies: Count how many data points fall within each bin.
-
Draw the Histogram: Draw the histogram using the calculated frequencies. The horizontal axis represents the bins, and the vertical axis represents the frequency. Draw a bar for each bin, with its height corresponding to the frequency of data points in that bin.
Interpreting Histograms: Unveiling Data Patterns
Once a histogram is constructed, it reveals valuable insights into the data's distribution. Analyzing a histogram involves looking for several key characteristics:
-
Shape: The overall shape of the histogram indicates the type of distribution. Common shapes include:
- Symmetrical: The data is evenly distributed around the center.
- Skewed Right (Positively Skewed): The tail extends to the right, indicating a concentration of data points at lower values and a few high outliers.
- Skewed Left (Negatively Skewed): The tail extends to the left, indicating a concentration of data points at higher values and a few low outliers.
- Uniform: All bins have roughly equal frequencies, suggesting a uniform distribution.
- Bimodal: The histogram has two distinct peaks, suggesting the presence of two separate groups within the data.
- Multimodal: The histogram has more than two peaks, suggesting the data comes from multiple underlying distributions.
-
Center: The center of the distribution can be approximated by looking at the midpoint of the histogram. Measures like the mean, median, and mode can provide more precise estimations.
-
Spread: The spread, or variability, of the data is indicated by the width of the histogram. A wider histogram suggests greater variability than a narrower one. Statistical measures like range, interquartile range (IQR), and standard deviation can quantify this spread.
-
Outliers: Extreme values that fall significantly outside the main body of the data are called outliers. They can be identified as bars separated from the main distribution.
Applications of Histograms: Across Various Fields
Histograms find extensive use across a wide array of fields, providing valuable insights into various data types. Here are some examples:
-
Quality Control: Histograms are frequently used in manufacturing to monitor the quality of products. They can help identify variations in dimensions, weight, or other crucial parameters. This enables manufacturers to adjust their processes to ensure consistent quality.
-
Financial Analysis: Histograms can be used to visualize stock prices, returns, and other financial data. This analysis can help in risk assessment and decision-making.
-
Healthcare: Histograms can illustrate the distribution of patient ages, blood pressure, or other health indicators. This can aid in identifying trends and informing treatment strategies.
-
Environmental Science: Histograms are used to analyze environmental data, such as rainfall patterns, temperature fluctuations, and pollutant levels.
-
Social Sciences: Histograms visualize distributions of survey responses, helping researchers analyze opinions, attitudes, and behaviors.
-
Image Processing: In digital image processing, histograms represent the distribution of pixel intensities, enabling manipulation for contrast enhancement or other image adjustments.
Choosing the Right Bin Width: Optimizing the Histogram
The choice of bin width significantly impacts a histogram's interpretability. Too few bins can obscure important details, resulting in a smoothed representation that masks underlying variations. Conversely, too many bins can lead to a jagged and noisy histogram that is difficult to interpret, highlighting random fluctuations rather than underlying trends.
Several methods exist to choose an appropriate bin width:
-
Sturges' Formula: This is a simple formula that estimates the optimal number of bins based on the number of data points. However, it can be less effective with skewed or multimodal distributions.
-
Square Root Rule: This rule suggests using the square root of the number of data points as the number of bins. It's generally more robust than Sturges' formula for various distributions.
-
Freedman-Diaconis Rule: This method takes into account the data's spread and is particularly useful for skewed distributions. It uses the interquartile range (IQR) to determine the bin width.
Histograms vs. Other Data Visualization Techniques
While histograms are excellent tools for visualizing numerical data distributions, it's important to understand their limitations and the advantages of alternative methods:
-
Bar Charts: Unlike histograms, bar charts display categorical data, not numerical data. They are effective for comparing frequencies across different categories.
-
Box Plots: Box plots provide a summary of the data's distribution, showing the median, quartiles, and outliers. They are less detailed than histograms but effective for comparing distributions across multiple groups.
-
Density Plots: Density plots offer a smooth representation of data distributions, emphasizing the probability density at different values. They are particularly useful for visualizing continuous data.
Advanced Histogram Techniques and Considerations
While basic histogram construction is straightforward, several advanced techniques can enhance their utility:
-
Kernel Density Estimation: This technique smoothes the histogram, creating a more continuous representation of the data distribution. It's especially beneficial when dealing with limited data points or noisy data.
-
Cumulative Frequency Histograms: Instead of showing the frequency of data points within each bin, these histograms display the cumulative frequency, providing information about the proportion of data points below a certain value.
-
Overlaying Multiple Histograms: Comparing multiple datasets can be achieved by overlaying their histograms on the same plot, allowing for a direct visual comparison of their distributions.
Conclusion: The Versatility and Power of Histograms
Histograms provide a powerful and versatile tool for visualizing the distribution of numerical data. By understanding their construction, interpretation, and various applications, data analysts and researchers can gain valuable insights into their data, informing decision-making in diverse fields. The choice of bin width, the consideration of the data's shape, and an understanding of alternative visualization techniques are crucial for maximizing the effectiveness of histograms as a data analysis tool. Remember to tailor your histogram creation to the specific data and the insights you aim to extract. Proper selection of bin width and interpretation of the shape are key to deriving meaningful information from your histograms.
Latest Posts
Latest Posts
-
Classify Each Of The Following As Acidic Basic Or Neutral
Apr 20, 2025
-
Round 7 698 To The Nearest Tenth
Apr 20, 2025
-
What Is The Cube Root Of 2
Apr 20, 2025
-
What Is The Formula For Magnesium Sulfide
Apr 20, 2025
-
Identify The Correct And Incorrect Statements
Apr 20, 2025
Related Post
Thank you for visiting our website which covers about A Histogram Is A Graphical Representation Of . We hope the information provided has been useful to you. Feel free to contact us if you have any questions or need further assistance. See you next time and don't miss to bookmark.